Deciphering novel molecular gene expression signatures and pathways in cystic fibrosis through integrative bioinformatics strategies
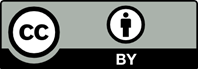
Cystic fibrosis (CF), a fatal autosomal recessive disorder, is triggered by a genetic alteration of the CF transmembrane conductance regulator (CFTR) gene. On a global scale, around one in 3000 live births are affected with CF annually. While diagnosis and therapy are available for CF patients with non-specific and rare mutations, the current research is dedicated to exploring customized biomarkers, genes, signaling networks, and therapy for improving the management of CF. Although still in the early stages of development and validation, mRNA and gene-based treatment strategies are aimed to target patients who are resistant to CFTR gene restoration therapies. In this study, we utilized the systems biology approaches integrated with gene expression analysis to identify novel biomarkers and pathways for CF treatment. At first, out of 54,676 differentially expressed genes, we identified 104 upregulated and 107 downregulated genes. The upregulated genes were largely concentrated on Glutamatergic synapses, and the downregulated genes were enriched in ubiquitin-mediated proteolysis. Utilizing the enrichment analysis, we explored deeper into the pathways linked to these genes, with emphasis on relevant pathways involving bronchial epithelial cells. Following the enrichment analysis, we identified six essential genes: WWP2, RNASEL, CUL1, CDC42, HDAC4, and UBA2. Furthermore, the discovered genes were evaluated using expression profile analysis. Finally, our data indicate that the WWP2 gene has a critical role in CF management. The current findings provide a coherent theoretical foundation for future experiments to further explore the WWP2 gene as a unique and prognostic target for developing an effective CF therapeutic approach.
- Schaffer K. Epidemiology of infection and current guidelines for infection prevention in cystic fibrosis patients. J Hosp Infect. 2015;89(4):309-313.
- Guo J, Garratt A, Hill A. Worldwide rates of diagnosis and effective treatment for cystic fibrosis. J Cyst Fibros. 2022;21(3):456-462. doi: 10.1016/j.jcf.2022.01.009
- Stephenson AL, Stanojevic S, Sykes J, Burgel PR. The changing epidemiology and demography of cystic fibrosis. Presse Med. 2017;46(6 Pt 2):e87-e95. doi: 10.1016/j.lpm.2017.04.012
- Burgel PR, Burnet E, Regard L, Martin C. The changing epidemiology of cystic fibrosis. Chest. 2023;163(1):89-99. doi: 10.1016/j.chest.2022.07.004
- Abubakar Bobbo K, Ahmad U, Chau DM, Nordin N, Abdullah S. A comprehensive review of cystic fibrosis in Africa and Asia. Saudi J Biol Sci. 2023;30(7):103685. doi: 10.1016/j.sjbs.2023.103685
- Strandvik B. Fatty acid metabolism in cystic fibrosis. N Engl J Med. 2004;350(6):605-607. doi: 10.1056/nejme038217
- Castellani S, Di Gioia S, di Toma L, Conese M. Human cellular models for the investigation of lung inflammation and mucus production in cystic fibrosis. Anal Cell Pathol (Amst). 2018;2018:3839803. doi: 10.1155/2018/3839803
- Rommens JM. Identification of the cystic fibrosis gene: Chromosome walking and jumping. Trends Genet. 1989;5(C):363. doi: 10.1016/0168-9525(89)90154-6
- Balloy V, Varet H, Dillies MA, et al. Normal and cystic fibrosis human bronchial epithelial cells infected with Pseudomonas aeruginosa exhibit distinct gene activation patterns. PLoS One. 2015;10(10):e0140979. doi: 10.1371/journal.pone.0140979
- Awatade NT, Wong SL, Hewson CK, et al. Human primary epithelial cell models: Promising tools in the era of cystic fibrosis personalized medicine. Front Pharmacol. 2018;9:1429. doi: 10.3389/fphar.2018.01429
- Cheng SH, Gregory RJ, Marshall J, et al. Defective intracellular transport and processing of CFTR is the molecular basis of most cystic fibrosis. Cell. 1990;63(4):827-834. doi: 10.1016/0092-8674(90)90148-8
- Farinha CM, King-Underwood J, Sousa M, et al. Revertants, low temperature, and correctors reveal the mechanism of F508del-CFTR rescue by VX-809 and suggest multiple agents for full correction. Chem Biol. 2013;20(7):943-955. doi: 10.1016/j.chembiol.2013.06.004
- Capurro V, Tomati V, Sondo E, et al. Partial rescue of f508del-cftr stability and trafficking defects by double corrector treatment. Int J Mol Sci. 2021;22(10):5262. doi: 10.3390/ijms22105262
- Esposito C, Kamper M, Trentacoste J, Galvin S, Pfister H, Wang J. Advances in the cystic fibrosis drug development pipeline. Life. 2023;13(9):1835. doi: 10.3390/life13091835
- Levy H, Jia S, Pan A, et al. Identification of molecular signatures of cystic fibrosis disease status with plasma-based functional genomics. Physiol Genomics. 2019;51(1):27-41. doi: 10.1152/physiolgenomics.00109.2018
- Wei T, Sui H, Su Y, et al. Research advances in molecular mechanisms underlying the pathogenesis of cystic fibrosis: From technical improvement to clinical applications (Review). Mol Med Rep. 2020;22(6):4992-5002. doi: 10.3892/mmr.2020.11607
- Oany AR, Mia M, Pervin T, Hasan MN, Hirashima A. Identification of potential drug targets and inhibitor of the pathogenic bacteria Shigella flexneri 2a through the subtractive genomic approach. In Silico Pharmacol. 2018;6(1):11. doi: 10.1007/s40203-018-0048-2
- Oany AR, Mia M, Pervin T, Junaid M, Hosen SMZ, Moni MA. Design of novel viral attachment inhibitors of the spike glycoprotein (S) of severe acute respiratory syndrome coronavirus-2 (SARS-CoV-2) through virtual screening and dynamics. Int J Antimicrob Agents. 2020;56(6):106177. doi: 10.1016/j.ijantimicag.2020.106177
- Oany AR, Mia M, Pervin T, Alyami SA, Moni MA. Integrative systems biology approaches to identify potential biomarkers and pathways of cervical cancer. J Pers Med. 2021;11(5):363. doi: 10.3390/jpm11050363
- Oany AR, Pervin T, Moni MA. Pharmacoinformatics based elucidation and designing of potential inhibitors against Plasmodium falciparum to target importin α/β mediated nuclear importation. Infect Genet Evol. 2021;88:104699. doi: 10.1016/j.meegid.2020.104699
- Oany AR, Pervin T, Mia M, et al. Vaccinomics approach for designing potential peptide vaccine by targeting Shigella spp. Serine protease autotransporter subfamily protein siga. J Immunol Res. 2017;2017:6412353. doi: 10.1155/2017/6412353
- Rahman MR, Islam T, Zaman T, et al. Identification of molecular signatures and pathways to identify novel therapeutic targets in Alzheimer’s disease: Insights from a systems biomedicine perspective. Genomics. 2020;112(2):1290-1299. doi: 10.1016/j.ygeno.2019.07.018
- Clough E, Barrett T. The gene expression omnibus database. Methods Mol Biol. 2016;1418:93-110. doi: 10.1007/978-1-4939-3578-9_5
- Ritchie ME, Phipson B, Wu D, et al. Limma powers differential expression analyses for RNA-sequencing and microarray studies. Nucleic Acids Res. 2015;73(7):e47. doi: 10.1093/nar/gkv007
- Benjamini BY, Yekutieli D. The control of the false discovery rate in multiple testing under dependency. Ann Stat. 2001;29(4):1165-1188.
- Aubert J, Bar-Hen A, Daudin JJ, Robin S. Determination of the differentially expressed genes in microarray experiments using local FDR. BMC Bioinformatics. 2004;5:125. doi: 10.1186/1471-2105-5-125
- Pawitan Y, Michiels S, Koscielny S, Gusnanto A, Ploner A. False discovery rate, sensitivity and sample size for microarray studies. Bioinformatics. 2005;21(13):3017-3024. doi: 10.1093/bioinformatics/bti448
- Islam MR, Ahmed ML, Kumar Paul B, Bhuiyan T, Ahmed K, Moni MA. Identification of the core ontologies and signature genes of polycystic ovary syndrome (PCOS): A bioinformatics analysis. Informatics Med Unlocked. 2020;18:100304. doi: 10.1016/j.imu.2020.100304
- Jiao X, Sherman BT, Huang DW, et al. DAVID-WS: A stateful web service to facilitate gene/protein list analysis. Bioinformatics. 2012;28(13):1805-1806. doi: 10.1093/bioinformatics/bts251
- Xia J, Gill EE, Hancock REW. NetworkAnalyst for statistical, visual and network-based meta-analysis of gene expression data. Nat Protoc. 2015;10(6):823-844. doi: 10.1038/nprot.2015.052
- Ashburner M, Ball CA, Blake JA, et al. Gene ontology: Tool for the unification of biology. Nat Genet. 2000;25:25-29. doi: 10.1038/75556
- Hulsegge I, Kommadath A, Smits MA. Globaltest and GOEAST: Two different approaches for Gene Ontology analysis. BMC Proc. 2009;3(S4):S10. doi: 10.1186/1753-6561-3-s4-s10
- Kanehisa M, Goto S. KEGG: Kyoto encyclopedia of genes and genomes. Nucleic Acids Res. 2000;28(1):27-30. doi: 10.1093/nar/28.1.27
- Kanehisa M, Sato Y, Kawashima M, Furumichi M, Tanabe M. KEGG as a reference resource for gene and protein annotation. Nucleic Acids Res. 2016;44(D1):D457-D462. doi: 10.1093/nar/gkv1070
- Zhang S, Bodenreider O. Law and order: Assessing and enforcing compliance with ontological modeling principles in the Foundational Model of Anatomy. Comput Biol Med. 2006;36(7-8):674-693. doi: 10.1016/j.compbiomed.2005.04.007
- Szklarczyk D, Franceschini A, Wyder S, et al. STRING v10: Protein-protein interaction networks, integrated over the tree of life. Nucleic Acids Res. 2015;43(D1):D447-D452. doi: 10.1093/nar/gku1003
- Shannon P, Markiel A, Ozier O, et al. Cytoscape: A software environment for integrated models of biomolecular interaction networks. Genome Res. 2003;13:2498-2504. doi: 10.1101/gr.1239303
- Hogue CW, Groll M. An automated method for finding molecular complexes in large protein interaction networks. BMC Bioinformatics. 2001;29(1):137-140. doi: 10.1093/nar/29.1.137
- Chin CH, Chen SH, Wu HH, Ho CW, Ko MT, Lin CY. cytoHubba: Identifying hub objects and sub-networks from complex interactome. BMC Syst Biol. 2014;8 Suppl 4(Suppl 4):S11. doi: 10.1186/1752-0509-8-S4-S11
- Wang G. Genome editing for cystic fibrosis. Cells. 2023;12(12):1555. doi: 10.3390/cells12121555
- Paterson SL, Barry PJ, Horsley AR. Tezacaftor and ivacaftor for the treatment of cystic fibrosis. Expert Rev Respir Med. 2020;14(1):15-30. doi: 10.1080/17476348.2020.1682998
- Kotnala S, Dhasmana A, Kashyap VK, Chauhan SC, Yallapu MM, Jaggi M. A bird eye view on cystic fibrosis: An underestimated multifaceted chronic disorder. Life Sci. 2021;268:118959. doi: 10.1016/j.lfs.2020.118959
- O’Neal WK, Knowles MR. Cystic fibrosis disease modifiers: Complex genetics defines the phenotypic diversity in a monogenic disease. Annu Rev Genomics Hum Genet. 2018;19:201-222. doi: 10.1146/annurev-genom-083117-021329
- Bienvenu T, Nguyen-Khoa T. Current and future diagnosis of cystic fibrosis: Performance and limitations. Arch Pédiatr. 2020;27 Suppl 1:eS19-eS24. doi: 10.1016/S0929-693X(20)30046-4
- Neutelings T, Lambert CA, Nusgens BV, Colige AC. Effects of mild cold shock (25 C) followed by warming up at 37 C on the cellular stress response. PLoS One. 2013;8(7):e69687. doi: 10.1371/journal.pone.0069687
- Sit ST, Manser E. Rho GTPases and their role in organizing the actin cytoskeleton. J Cell Sci. 2011;124(5):679-683. doi: 10.1242/jcs.064964
- Ferru-Clément R, Fresquet F, Norez C, et al. Involvement of the Cdc42 pathway in CFTR post-translational turnover and in its plasma membrane stability in airway epithelial cells. PLoS One. 2015;10(3):e0118943. doi: 10.1371/journal.pone.0118943
- Gundu C, Arruri VK, Yadav P, et al. Dynamin-Independent Mechanisms of Endocytosis and Receptor Trafficking. Cells. 2022;11(16):2557. doi: 10.3390/cells11162557
- Ganeshan R, Nowotarski K, Di A, Nelson DJ, Kirk KL. CFTR surface expression and chloride currents are decreased by inhibitors of N-WASP and actin polymerization. Biochim Biophys Acta. 2007;1773(2):192-200. doi: 10.1016/j.bbamcr.2006.09.031
- Jaganathan D, Bruscia EM, Kopp BT. Emerging concepts in defective macrophage phagocytosis in cystic fibrosis. Int J Mol Sci. 2022;23(14):7750. doi: 10.3390/ijms23147750
- Boyle MP. Strategies for identifying modifier genes in cystic fibrosis. Proc Am Thorac Soc. 2007;4(1):52-57. doi: 10.1513/pats.200605-129JG
- Barone S, Cassese E, Alfano AI, Brindisi M, Summa V. Chasing a breath of fresh air in cystic fibrosis (CF): Therapeutic potential of selective HDAC6 inhibitors to tackle multiple pathways in CF pathophysiology. J Med Chem. 2022;65(4):3080-3097. doi: 10.1021/acs.jmedchem.1c02067
- Yoon S, Kang G, Eom GH. Hdac inhibitors: Therapeutic potential in fibrosis-associated human diseases. Int J Mol Sci. 2019;20(6):1329. doi: 10.3390/ijms20061329
- Wang Z, Qin G, Zhao TC. HDAC4: Mechanism of regulation and biological functions. Epigenomics. 2014;6(1):139-150. doi: 10.2217/epi.13.73
- Xie CM, Wei W, Sun Y. Role of SKP1-CUL1-F-box-protein (SCF) E3 ubiquitin ligases in skin cancer. J Genet Genomics. 2013;40(3):97-106. doi: 10.1016/j.jgg.2013.02.001
- Li R, Xu F, Wu X, Ji S, Xia R. CUL1-mediated organelle fission pathway inhibits the development of chronic obstructive pulmonary disease. Comput Math Methods Med. 2020;2020:5390107. doi: 10.1155/2020/5390107
- Roffel MP, Bracke KR, Heijink IH, Maes T. miR-223: A key regulator in the innate immune response in asthma and COPD. Front Med (Lausanne). 2020;7:196. doi: 10.3389/fmed.2020.00196
- Liu Q, Gao Y, Ci X. Role of Nrf2 and its activators in respiratory diseases. Oxid Med Cell Longev. 2019;2019:7090534. doi: 10.1155/2019/7090534
- Wei R, Chen G, Algehainy N, et al. RNase L is involved in liposaccharide-induced lung inflammation. Viruses. 2020;12(1):73. doi: 10.3390/v12010073
- Haque AA, Weinmann P, Biswas S, et al. RNA immunogenic assay: Simple method for detecting immunogenicity of in vitro transcribed mRNA. Adv Cell Gene Ther. 2020;3(2):1-10. doi: 10.1002/acg2.79
- Tian X, Chen Y, Peng Z, Lin Q, Sun A. NEDD4 E3 ubiquitin ligases: Promising biomarkers and therapeutic targets for cancer. Biochem Pharmacol. 2023;214:115641. doi: 10.1016/j.bcp.2023.115641
- Song MS, Pandolfi PP. The HECT family of E3 ubiquitin ligases and PTEN. Semin Cancer Biol. 2022;85:43-51. doi: 10.1016/j.semcancer.2021.06.012
- Chen H, Chew G, Devapragash N, et al. The E3 ubiquitin ligase WWP2 regulates pro-fibrogenic monocyte infiltration and activity in heart fibrosis. Nat Commun. 2022;13(1):7375. doi: 10.1038/s41467-022-34971-6
- Chen H, Moreno-Moral A, Pesce F, et al. WWP2 regulates pathological cardiac fibrosis by modulating SMAD2 signaling. Nat Commun. 2019;10(1):3616. doi: 10.1038/s41467-019-11551-9