Application of artificial intelligence in drug repositioning
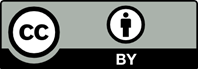
The use of artificial intelligence technologies in biology, pharmacy, and medicine has brought about a dramatic change in these industries. Drug repositioning is a method of drug development in the process of applying existing therapeutic agents to new diseases. This paper first outlines the use of artificial intelligence technology in the field of drug repositioning, then reviews a variety of application methods of artificial intelligence in the realm of drug repositioning, and finally summarizes the advantages and disadvantages of these methods, and proposes the difficulties faced by artificial intelligence in drug repositioning in the future and the corresponding suggestions to achieve the goal of helping researchers to develop more effective methods of drug repositioning.
Peng C, Hu Y, Chen L, et al., 2020, A review of drug repositioning algorithms based on machine learning and big data mining. Adv Pharm, 44(1): 6.
Zhang W, Gu F, Fu YK, et al., 2021, Research progress of drug repositioning in new drug development. Anim Husbandry Vet Med, 53(12): 123–127.
Nelson BS, Kremer DM, Lyssiotis CA, 2018, New tricks for an old drug. Nat Chem Biol, 14: 990–991.
Li FT, Liu MX, Wang XB, et al., 2022, Progress of COVID-19 drug repositioning study. China Pharm Biotechnol, 17(4): 8.
Huang F, Yang HF, Zhu X, 2021, Advances in the application of artificial intelligence in new drug discovery. Adv Pharm, 2021(7): 502–511.
Jarada TN, Rokne JG, Alhajj R, 2020, A review of computational drug repositioning: strategies, approaches, opportunities, challenges, and directions. J Cheminform, 12(1): 46. https://doi.org/10.1186/s13321-020-00450-7
Cheng, Fei X, Liu, et al., 2012, Prediction of drug-target interactions and drug repositioning via network-based inference. PLoS Comput Biol, 8(5): e1002503. https://doi.org/10.1371/journal.pcbi.1002503
Guney E, Menche J, Vidal M, et al., 2016, Network-based in silico drug efficacy screening. Nat Commun, 7(1): 10331. https://doi.org/10.1038/ncomms10331
Wang W, Yang S, Zhang X, et al., 2014, Drug repositioning by integrating target information through a heterogeneous network model. Bioinformatics, 30(20): 2923–2930. https://doi.org/10.1093/bioinformatics/btu403
Chen, X, Liu, Yan GY, 2012, Drug-target interaction prediction by random walk on the heterogeneous network. Mol Biosyst, 8(7): 1970–1978.
Olayan RS, Haitham A, Bajic VB, 2018, DDR: Efficient computational method to predict drug–target interactions using graph mining and machine learning approaches. Bioinformatics, 34(7): 1164–1173. https://doi.org/10.1093/bioinformatics/btx731
Peng J, Li J, Shang X, 2020, A learning-based method for drug-target interaction prediction based on feature representation learning and deep neural network. BMC Bioinformatics, 21(Suppl 13): 394.
Ji B, You ZH, Jiang HJ, et al., 2020, Prediction of drug-target interactions from multi-molecular network based on LINE network representation method. J Transl Med, 18(1): 347. https://doi.org/10.1186/s12967-020-02490-x
Lu XG, Liu F, Li JX, et al., 2021, A drug target prediction method based on multi-source data fusion and network structure perturbation CN112420126A. China: Hunan Province.
He JY, Yang XX, Gong Z, 2021. A computational drug repositioning method based on memory network and attention CN112331275A. China: Jiangsu Province.
Wang X, Li Q, Liu Y, et al., 2022, Drug repositioning of COVID-19 based on mixed graph network and ion channel. Math Biosci Eng, 19(4): 3269–3284.
Wall ME, Rechtsteiner A, Rocha LM, 2002, Singular Value Decomposition and Principal Component Analysis. Springer, Germany.
Shen M, Xiao Y, Golbraikh A, et al., 2003, Development and validation of k-nearest-neighbor QSPR models of metabolic stability of drug candidates. J Med Chem, 46(3): 3013–3020. https://doi.org/10.1021/jm020491t
Susnow RG, Dixon SL, 2003, Use of robust classification techniques for the prediction of human cytochrome P450 2D6 inhibition. J Chem Inform Comput Sci, 43(4): 1308–1315.
Christianini N, Shawe-Taylor J, 2002, Support Vector Machines and other Kernel-based Learning Methods. Cambridge University Press, United Kingdom.
Guengerich FP, 2006, Cytochrome P450s and other enzymes in drug metabolism and toxicity. AAPS J, 8(1): E101–E111.
Cheng F, Yu Y, Shen J, et al., 2011, Classification of cytochrome P450 inhibitors and noninhibitors using combined classifiers. J Chem Inform Model, 51: 996–1011. https://doi.org/10.1021/ci200028n
Napolitano F, Zhao Y, Moreira VM, et al., 2013, Drug repositioning: A machine-learning approach through data integration. J Cheminform, 5(1): 30.
Gottlieb A, Stein GY, Ruppin E, et al., PREDICT: A method for inferring novel drug indications with application to personalized medicine. Mol Syst Biol, 7(1): 496. https://doi.org/10.1038/msb.2011.26
Gönen M, 2012, Predicting drug-target interactions from chemical and genomic kernels using Bayesian matrix factorization. Bioinformatics, 28(18): 2304–2310. https://doi.org/10.1093/bioinformatics/bts360
Aliper A, Plis S, Artemov A, et al., 2016, Deep learning applications for predicting pharmacological properties of drugs and drug repurposing using transcriptomic data. Mol Pharm, 13(7): 2524–2530. https://doi.org/10.1021/acs.molpharmaceut.6b00248
Segler MH, Preuss M, Waller MP, 2018, Planning chemical syntheses with deep neural networks and symbolic AI. Nature, 555(7698): 604–610. https://doi.org/10.1038/nature25978
Hughes TB, Swamidass SJ, 2017, Deep learning to predict the formation of quinone species in drug metabolism. Chem Res Toxicol, 30(2): 642–656.
Turk S, Merget B, Rippmann F, et al., Coupling matched molecular pairs with machine learning for virtual compound optimization. J Chem Inform Model, 57(12): 3079–3085.https://doi.org/10.1021/acs.jcim.7b00298
Zhang L, 2017, Research on Protein Class and Protein- Ligand Interaction Prediction Based on Machine Learning. Shandong University, China.
Chen P, Bao TJ, Yu SH, 2021, Drug relocation recommendation algorithm based on multi-similarity fusion. Comput Technol Dev, 31(1): 168-174.
Zhang H, An XY, Liu CH, 2022, Drug knowledge discovery based on multi-source semantic knowledge graphs: Empirical evidence with drug repositioning. Data Anal Knowl Discov, 6(7): 87–98.
Zeng X, Zhu S, Liu X, et al., 2019, deepDR: A network-based deep learning approach to in silico drug repositioning. Bioinformatics, 35(24): 5191–5198. https://doi.org/10.1093/bioinformatics/btz418
Xuan P, Song Y, Zhang T, et al., 2019, Prediction of potential drug-disease associations through deep integration of diversity and projections of various drug features. Int J Mol Sci,1 20(17): 4102.
Yang M, Luo H, Li Y, et al., 2019, Drug repositioning based on bounded nuclear norm regularization. Bioinformatics, 35(14): i455–i463. https://doi.org/10.1093/bioinformatics/btz331
Zhang W, Xu H, Li X, et al., 2020, DRIMC: An improved drug repositioning approach using Bayesian inductive matrix completion. Bioinformatics, 36(9): 2839–2847. https://doi.org/10.1093/bioinformatics/btaa062
Peng LH, Tian XF, Zhou LQ, et al., 2021, A drug-target relationship prediction method based on deep forest and PU learning, CN112652355A. China: Hunan Province.
Yan Y, Yang M, Zhao H, et al., 2022, Drug repositioning based on multi-view learning with matrix completion. Brief Bioinform, 23(3): bbac054. https://doi.org/10.1093/bib/bbac054