Network biology: Recent advances and challenges
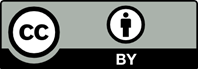
Biological networks have garnered widespread attention. The development of biological networks has spawned the birth of a new interdisciplinary field – network biology. Network biology involves the exploration of complex biological systems through biological networks for better understanding of biological functions. This paper reviews some of the recent development of network biology. On the one hand, various approaches to constructing different types of biological networks are reviewed, and the pros and cons of each approach are discussed; on the other hand, the recent advances of information mining in biological networks are reviewed. The principles of guilt-by-association and guilt-by-rewiring in network biology and their applications are discussed. Although great advances have been achieved in the field of network biology over the past decades, there are still many challenging issues. First, efficient and reliable network inference algorithms for high-dimensional and highly noisy omics data are still in great demand. Second, the research focus will be on multilayer biological network theory. This plays a critical role in the exploration of the multi-scale or dynamical characteristics of complex biomolecular networks by integrating multi-source heterogeneous omics data. Third, a close cooperation among biologists, medical workers, and researchers from network science is still a prerequisite in the applications of network biology. The rapid development of network biology will undoubtedly raise important clues for understanding complex phenotypes in biological systems.
Barabási AL, 2016, Network Science. Cambridge: Cambridge University Press. https://doi.org/10.1109/MNET.2010.5634435
Chen GR, Wang HF, Li X. 2015, Introduction to Complex Networks: Models, Structures and Dynamics. 2nd ed. Beijing: Higher Education Press.
Chen LN, Wang RS, Zhang XS, 2009, Biomolecular Networks: Methods and Applications in Systems Biology. Hoboken: John Wiley and Sons. https://doi.org/10.1002/9780470488065.ch5
Lü JH, Wang P, 2020, Modeling and Analysis of Bio- Molecular Networks. Singapore, Berlin: Springer.
Alon U, 2007, An Introduction to Systems Biology: Design Principles of Biological Circuits. London: Chapman and Hall, CRC.
Costanzo M, VanderSluis B, Koch EN, et al., 2016, A global genetic interaction network maps a wiring diagram of cellular function. Science, 353(6306): aaf1420. https://doi.org/10.1126/science.aaf1420
Wang P, Zhang YH, Lü JH, et al., 2015, Functional characteristics of additional positive feedback in genetic circuits. Nonlinear Dyn, 79(1): 397–408. https://doi.org/10.1007/s11071-014-1673-x
Rolland T, Taşan M, Charloteaux B, et al., 2014, A proteome-scale map of the human interactome network. Cell, 159(5): 1212–1226. https://doi.org/10.1016/j.cell.2014.10.050
Huttlin EL, Ting L, Bruckner RJ, et al., 2015, The BioPlex network: A systematic exploration of the human interactome. Cell, 162(2): 425–440. https://doi.org/10.1016/j.cell.2015.06.043
Huttlin EL, Bruckner RJ, Paulo JA, et al., 2017, Architecture of the human interactome defines protein communities and disease networks. Nature, 545(7655): 505–509. https://doi.org/10.1038/nature22366
Luck K, Kim DK, Lambourne L, et al., 2020, A reference map of the human binary protein interactome. Nature, 580(7803): 402–408. https://doi.org/10.1038/s41586-020-2188-x
Wan C, Borgeson B, Phanse S, et al., 2015, Panorama of ancient metazoan macromolecular complexes. Nature, 525(7569): 339–344. https://doi.org/10.1038/nature14877
Wang P, Chen Y, Lü JH, et al., 2016, Graphical features of functional genes in Human protein interaction network. IEEE Trans Biomed Circuits Syst, 10(3): 707–720. https://doi.org/10.1109/TBCAS.2015.2487299
Lee TI, Rinaldi N, Robert F, et al., 2002, Transcriptional regulatory networks in Saccharomyces cerevisiae. Science, 298(55943): 799–804. https://doi.org/10.1126/science.1075090
Bhler J, Marguerat S, 2013, Transcriptional Regulatory Network. Berlin, New York: Springer. https://doi.org/10.1007/978-1-4419-9863-7_737
Guelzim N, Bottani S, Bourgine P, et al., 2002, Topological and causal structure of the yeast transcriptional regulatory network. Nat Genet, 31(1): 60–63. https://doi.org/10.1038/ng873
Ravasz E, Somera AL, Mongru DA, et al., 2002, Hierarchical organization of modularity in metabolic networks. Science, 297(5586): 1551–1555. https://doi.org/10.1126/science.1073374
Pols T, Sikkema HR, Gaastra BF, et al., 2019, A synthetic metabolic network for physicochemical homeostasis. Nat Commun, 10(1): 4239. https://doi.orgt/10.1038/s41467-019-12287-2
Soyer OS, Salathé M, Bonhoeffer S, 2006, Signal transduction networks: Topology, response and biochemical processes. J Theor Biol, 238(2): 416–425. https://doi.org/10.1016/j.jtbi.2005.05.030
Ikeda F, Lahav G, 2013, Signal transduction and signaling networks. Mol Biol Cell, 24(6): 676. https://doi/.org/10.1091/mbc.E12-12-0877
Papin JA, Hunter T, Palsson BO, et al., 2005, Reconstruction of cellular signalling networks and analysis of their properties. Nat Rev Mol Cell Bio, 6(2): 99–111. https://doi.org/10.1038/nrm1570
Langfelder P, Horvath S, 2008, WGCNA: An R package for weighted correlation network analysis. BMC Bioinformat, 9(1): 559. https://doi.org/10.1186/1471-2105-9-559
Zhang B, Horvath S, 2005, A general framework for weighted gene co-expression network analysis. Stat Appl Genet Mol Biol, 4(1): 17. https://doi.org/10.2202/1544-6115.1128
Lai Y, Wu B, Chen L, et al., 2004, A statistical method for identifying differential gene-gene co-expression patterns. Bioinformatics, 20(17): 3146–3155. https://doi.org/10.1093/bioinformatics/bth379
Van Dam S, Vosa U, van der Graaf A, et al., 2018, Gene co-expression analysis for functional classification and gene disease predictions. Brief Bioinformat, 19(4): 575–592. https://doi.org/10.1093/bib/bbw139
Wang P, Yang CL, Chen H, et al., 2018, Exploring transcription factors reveals crucial members and regulatory networks involved in different abiotic stresses in Brassica napus L. BMC Plant Biol, 18(1): 202. https://doi.org/10.1186/s12870-018-1417-z
Goh K, Cusick ME, Valle D, et al., 2007, The human disease network. Proc Natl Acad Sci U S A, 104(21): 8685–8690. https://doi.org/10.1073/pnas.0701361104
Barabási Al, Gulbahce N, Loscalzo J, 2011, Network medicine: A network-based approach to human disease. Nat Rev, 12(1): 56–68. https://doi.org/10.1038/nrg2918
Yıldırım MA, Goh K, Cusick ME, et al., 2007, Drug-target network. Nat Biotech, 25(10): 1119–1126. https://doi.org/10.1038/nbt1338
Gosak M, Markovic R, Dolensek J, et al., 2018, Network science of biological systems at different scales: A review. Phys Life Rev, 24: 118–135. https://doi.org/10.1016/j.plrev.2017.11.003
Barabási AL, Oltvai Z, 2004, Network biology: Understanding the cell’s functional organization. Nat Rev Genet, 5(2): 101–113. https://doi.ortg/10.1038/nrg1272
Liu C, Ma YF, Zhao J, et al., 2020, Computational network biology: Data, models, and applications. Phys Rep, 846: 1–66. https://doi.org/10.1016/j.physrep.2019.12.004
Yuan M, Lin Y, 2007, Model selection and estimation in the Gaussian graphical model. Biometrika, 94(1): 19–35. https://doi.org/10.1016/j.jmva.2012.01.005
Friedman J, Hastie T, Tibshirani R, 2007, Sparse inverse covariance estimation with the graphical lasso. Biostatistics, 9(3): 432–441. https://doi.org/10.1093/biostatistics/kxm045
Cai T, Liu W, Luo X, 2011, A constrained ℓ1 minimization approach to sparse precision matrix estimation. J Amer Stat Assoc, 106(494): 594–607. https://doi.org/10.1198/jasa.2011.tm10155
Young PM, Trevor H, 2007, Penalized logistic regression for detecting gene interactions. Biostatistics, 9(1): 30–50. https://doi.org/10.1093/biostatistics/kxm010
Roy GG, Geard N, Verspoor K, et al., 2020, PoLoBag: Polynomial lasso bagging for signed gene regulatory network inference from expression data. Bioinformatics, 36(21): 5187–5193. https://doi.org/10.1093/bioinformatics/btaa651
Duarte NC, Becker SA, Jamshidi N, et al., 2007, Global reconstruction of the human metabolic network based on genomic and bibliomic data. Proc Natl Acad Sci U S A, 104(6): 1777–1782. https://doi.org/10.1073/pnas.0610772104
Wang Y, Joshi T, Xu D, et al., 2006, Inferring gene regulatory networks from multiple microarray datasets. Bioinformatics, 22(19): 2413–2420. https://doi.org/10.1093/bioinformatics/btl396
Finkle JD, Wu JJ, Bagheri N, 2018, Windowed granger causal inference strategy improves discovery of gene regulatory networks. Proc Natl Acad Sci U S A, 115(9): 2252–2257. https://doi.org/10.1073/pnas.1710936115
Wang YX, Huang H, 2014, Review on statistical methods for gene network reconstruction using expression data. J Theor Biol, 362: 53–61. https://doi.org/10.1016/j.jtbi.2014.03.040
Xu S, Zhang C, Wang P, et al., 2020, Variational bayesian weighted complex network reconstruction. Inform Sci, 521: 291–306. https://doi.org/10.1016/j.ins.2020.02.050
Jeong H, Mason SP, Barabási AL, et al. 2001, Lethality and centrality in protein networks. Nature, 411(6833): 41–42. https://doi.org/10.1038/35075138
Xu J, Li Y, 2006, Discovering disease-genes by topological features in human protein-protein interaction network. Bioinformatics, 22(22): 2800–2805. https://doi.org/10.1093/bioinformatics/btl467
Wu X, Jiang R, Zhang MQ, et al., 2008, Network-based global inference of human disease genes. Mol Syst Biol, 4(1): 189. https://doi.org/10.1038/msb.2008.27
Fessenden M, 2017, Protein maps chart the causes of disease. Nature, 549(7671): 293–295. https://doi.org/10.1038/549293a
Wang P, LüJH, Yu XH, 2014, Identification of important nodes in directed biological networks: A network motif approach. PLoS One, 9(8): e106132. https://doi.org/10.1371/journal.pone.0106132
Bracken CP, Scott HS, Goodall GJ, 2016, A network-biology perspective of microRNA function and dysfunction in cancer. Nat Rev Genet, 17(12): 719–732. https://doi.org/10.1038/nrg.2016.134
Chen LN, Liu R, Liu ZP, et al., 2012, Detecting early-warning signals for sudden deterioration of complex diseases by dynamical network biomarkers. Sci Rep, 2: 342. https://doi.org/10.1038/srep00342
Azhagesan K, Ravindran B, Raman K, 2018, Network-based features enable prediction of essential genes across diverse organisms. PLoS One, 13(12): e0208722. https://doi.org/10.1371/journal.pone.0208722
Carson MB, Lu H, 2015, Network-based prediction and knowledge mining of disease genes. BMC Med Genomics, 8(Suppl 2): S9. https://doi.org/10.1186/1755-8794-8-S2-S9
Hou L, Chen M, Cho J, et al., 2014, Guilt-by-rewiring: Gene prioritization through network rewiring in genome wide association studies. Hum Mol Genet, 23(10): 2780–2790. https://doi.org/10.1093/hmg/ddt668
Li XY, Li WK, Zeng M, et al., 2020, Network-based methods for predicting essential genes or proteins: A survey. Brief Bioinforma, 21(2): 566–583. https://doi.org/10.1093/bib/bbz017
Newman ME, 2003, The structure and function of complex networks. SIAM Rev, 45(2): 167–256. https://doi.org/10.1137/S003614450342480
Ulrik B, 2001, A faster algorithm for betweenness centrality. J Math Sociol, 25(2): 163–177. https://doi.org/10.1080/0022250X.2001.9990249
Shai C, Shlomo H, Scott K, et al., 2007, A model of Internet topology using k-shell decomposition. Proc Natl Acad Sci U S A, 104(27): 11150–11154. https://doi.org/10.1073/pnas.0701175104
Chen DB, LüLY, Shang MS, et al., 2012, Identifying influential nodes in complex networks. Physica A Stat Mech Appl, 391(4): 1777–1787. https://doi.org/10.1016/j.physa.2011.09.017
Sergey B, Lawrence P, 1998, The anatomy of a large-scale hypertextual web search engine. Comput Networks ISDN Syst, 30: 107–117. https://doi.org/10.1016/S0169-7552(98)00110-X
Lü LY, Chen DB, Ren XL, et al., 2016, Vital nodes identification in complex networks. Phys Rep, 650: 1–63. https://doi.org/10.1016/j.physrep.2016.06.007
Xu S, Wang P, 2017, Identifying important nodes by adaptive LeaderRank. Physica A, 469: 654–664. https://doi.org/10.1016/j.physa.2016.11.034
Xu S, Wang P, Zhang CX, et al., 2019, Spectral learning algorithm reveals propagation capability of complex network. IEEE Trans Cybern, 49(12): 4253–4261. https://doi.org/10.1109/TCYB.2018.2861568
Watts DJ, Strogatz SH, 1998, Collective dynamics of ‘small-world’ networks. Nature, 393(6684): 440–442. https://doi.org/10.1038/30918
Humphries MD, Gurney K, 2008, Network ‘small-worldness: A quantitative method for determining canonical network equivalence. PLoS One, 3(4): e0002051. https://doi.org/10.1371/journal.pone.0002051
Barabási Al, Albert R, 1999, Emergence of scaling in random networks. Science, 286(5439): 509–512. https://doi.org/10.1126/science.286.5439.509
Yu HY, Braun P, Yildirim MA, et al., 2008, High-quality binary protein interaction map of the yeast interactome network. Science, 322(5898): 104–110. https://doi.org/10.1126/science.1158684
Wang P, Lü JH, Yu XH, et al., 2015, Duplication and divergence effect on network motifs in undirected bio-molecular networks. IEEE Trans Biomed Circuits Syst, 9(3): 312–320. https://doi.org/10.1109/TBCAS.2014.2343620
Stark C, Breitkreutz BJ, Reguly T, et al., 2006, BioGRID: A general repository for interaction datasets. Nucl Acids Res, 34(90001): D535–D539. https://doi.org/10.1093/nar/gkj109
Hamosh A, Scott AF, Amberger JS, et al., 2005, Online mendelian inheritance in man (OMIM), a knowledgebase of human genes and genetic disorders. Nucl Acids Res, 33(1): D514–D517. https://doi.org/10.1093/nar/gki033
Fields S, Song O, 1989, A novel genetic system to detect protein-protein interactions. Nature, 340(6230): 245–246. https://doi.org/10.1038/340245a0
SenGupta DJ, Zhang B, Kraemer B, et al., 1996, A three-hybrid system to detect RNA-protein interactions in vivo. Proc Natl Acad Sci U S A, 93(16): 8496–8501. https://doi.org/10.1073/pnas.93.16.8496
Yang JS, Garriga-Canut M, Link N, et al., 2018, rec- YnH enables simultaneous many-by-many detection of direct protein-protein and protein-RNA interactions. Nat Commun, 9(1): 3747. https://doi.org/10.1038/s41467-018-06128-x.
Szklarczyk D, Gable AL, Nastou KC, et al., 2021, The STRING database in 2021: Customizable protein-protein networks, and functional characterization of user-uploaded gene/ measurement sets. Nucl Acids Res, 49(D10: D605–D612. https://doi.org/10.1093/nar/gkaa1074
Peri S, Navarro JD, Amanchy R, et al., 2003, Development of human protein reference database as an initial platform for approaching systems biology in humans. Genome Res, 13(10): 2363–2371. https://doi.org/10.1101/gr.1680803
Vázquez A, Flammini A, Maritan A, et al., 2002, Modeling of protein interaction networks. Complexus, 1(1): 38–44. https://doi.org/10.1159/000067642
Rutjes T, 2007, Duplication-Divergence and Proteome Evolution Networks. Netherlands: Technische Universiteit Eindhoven.
Ispolatov I, Krapivsky PL, Yuryev A, 2005, Duplication-divergence model of protein interaction network,” Phys Rev E, Stat Nonlin Soft Matter Phys, 71(6): 061911. https://doi.org/10.1103/PhysRevE.71.061911
Pastor-Satorras R, Smith E, Solé RV, 2003, Evolving protein interaction networks through gene duplication. J Theor Biol, 222(2): 199–210. https://doi.org/10.1016/S0022-5193(03)00028-6
Xu CS, Liu ZR, Wang R, 2010, How divergence mechanisms influence disassortative mixing property in biology. Physica A Stat Mech Appl, 389(3): 643–650. https://doi.org/10.1016/j.physa.2009.09.016
Wan X, Cai SM, Zhou J, et al., 2010, Emergence of modularity and disassortativity in protein-protein interaction networks. Chaos, 20(4): 045113. https://doi.org/10.1063/1.3517107
Teichmann SA, Babu MM, 2004, Gene regulatory network growth by duplication. Nat Genet, 36(5): 492–496. https://doi.org/10.1038/ng1340
Bhan A, Galas DJ, Dewey TG, 2002, A duplication growth model of gene expression networks. Bioinformatics, 18(11): 1486–1493. https://doi.org/10.1093/bioinformatics/18.11.1486
Wang P, Yu XH, LüJH, 2014, Identification and evolution of structurally dominant nodes in protein-protein interaction networks. IEEE Trans Biomed Circuits. Syst, 8(1): 87–97. https://doi.org/10.1109/TBCAS.2014.2303160
Solé RV, Pastor-Satorras R, Smith E, et al., 2002, A model of large-scale proteome evolution. Adv Complex Syst, 5: 43–54. https://doi.org/10.48550/arXiv.cond-mat/0207311
Mei GF, Wu XQ, Wang YF, et al., 2018, Compressive-sensing-based structure identification for multilayer networks. IEEE Trans Cybern, 48(2): 754–764. https://doi.org/10.1109/TCYB.2017.2655511
Wang YF, Wu XQ, Lü JH, et al., 2020, Topology identification in two-layer complex dynamical networks. IEEE Trans. Netw Sci Eng, 7(1): 538–548. https://doi.org/10.1109/TNSE.2018.2885163
Wu XQ, Zhao XY, Lü JH, et al., 2016, Identifying topologies of complex dynamical networks with stochastic perturbations. IEEE Trans Control Netw, 3(4): 379–389. https://doi.org/10.1109/TCNS.2015.2482178
Zhou J, Yu WW, Li XM, et al., 2009, Identifying the topology of a coupled FitzHugh-Nagumo neurobiological network via a pinning mechanism. IEEE Trans Neural Netw, 20(10): 1679–1684. https://doi.org/10.1109/TNN.2009.2029102
Liu Q, Ma C, Xiang B, et al., 2021, Inferring network structure and estimating dynamical process from Binary- State data via logistic regression. IEEE Trans Syst Man Cybern, 51(8): 4639–4649. https://doi.org/10.1109/TSMC.2019.2945363
Wu RL, Jiang LB, 2021, Recovering dynamic networks in big static datasets. Phys Rep, 912: 1–57. https://doi.org/10.1016/j.physrep.2021.01.003
Remondinin D, Nerettic N, Franceschi C, et al., 2007, Networks from gene expression time series: Characterization of correlation patterns. Int J Bifur Chaos, 17(7): 2477–2483. https://doi.org/10.1142/S0218127407018543
Song L, Langfelder P, Horvath S, 2012, Comparison of co-expression measures: Mutual information, correlation, and model based indices. BMC Bioinformat, 13: 328. https://doi.org/10.1186/1471-2105-13-328
Hong S, Chen X, Li J, et al., 2013, Canonical correlation analysis for RNA-seq co-expression networks. Nucl Acids Res, 41(8): e95. https://doi.org/10.1093/nar/gkt145
Skinnider M, Squair J, Foster L, 2019, Evaluating measures of association for single-cell transcriptomics. Nat Methods, 16: 381–386. https://doi.org/10.1038/s41592-019-0372-4
Liu ZP, 2018, Towards precise reconstruction of gene regulatory networks by data integration. Quant Biol, 6: 113–128. https://doi.org/10.1007/s40484-018-0139-4
Zhang X, Zhao X, He K, et al., 2012, Inferring gene regulatory networks from gene expression data by path consistency algorithm based on conditional mutual information. Bioinformatics, 28(1): 98–104. https://doi.org/10.1093/bioinformatics/btr626
Meyer P, Lafitte F, Bontempi G, 2008, minet: A R/ Bioconductor package for inferring large transcriptional networks using mutual information. BMC Bioinformatics, 9: 461. https://doi.org/10.1186/1471-2105-9-461
Wu XQ, Wang WH, Zheng WX, 2012, Inferring topologies of complex networks with hidden variables. Phys Rev E, 86(4): 046106. https://doi.org/10.1103/PhysRevE.86.046106
Beyer A, Bandyopadhyay S, Ideker T, 2007, Integrating physical and genetic maps: From genomes to interaction networks. Nat Rev Genet, 8(9): 699–710. https://doi.org/10.1038/nrg2144
Ghanbari M, Lasserre J, Vingron M, 2015, Reconstruction of gene networks using prior knowledge. BMC Syst Biol, 9(1): 84. https://doi.org/10.1186/s12918-015-0233-4
Altarawy D, Eid FE, Heath LS, 2017, PEAK: Integrating curated and noisy prior knowledge in gene regulatory network inference. J Comput Biol, 24(9): 863–873. https://doi.org/10.1089/cmb.2016.0199
Jansen R, Yu H, Greenbaum D, et al., 2003, A bayesian networks approach for predicting protein-protein interactions from genomic data. Science, 302(5644): 449–453. https://doi.org/10.1126/science.1087361
Xiao N, Zhou A, Kempher M, et al., 2022, Disentangling direct from indirect relationships in association networks. Proc Natl Acad Sci U S A, 119(2): e2109995119. https://doi.org/10.1073/pnas.2109995119
Chowdhury H, Bhattacharyya D, Kalita J, et al., 2019, (Differential) co-expression analysis of gene expression: A survey of best practices,” IEEE/ACM Trans Comput Biol Bioinform, 17(4): 1154–1173. https://doi.org/10.1109/TCBB.2019.2893170
Tesson B, Breitling R, Jansen R, 2010, DiffCoEx: A simple and sensitive method to find differentially coexpressed gene modules. BMC Bioinformatics, 11: 497. https://doi.org/10.1186/1471-2105-11-497
Watson M, 2006, CoXpress: Differential co-expression in gene expression data. BMC Bioinformatics, 7: 509. https://doi.org/10.1186/1471-2105-7-509
Ha J, Baladandayuthapani V, Do K, 2015, DINGO: Differential network analysis in genomics. Bioinformatics, 31(21): 3413–3420. https://doi.org/10.1093/bioinformatics/btv406
Wang P, Wang DJ, 2021, Gene differential co-expression networks based on RNA-seq data, construction and its applications. IEEE/ACM Trans Comput Biol Bioinform. https://doi.org/10.1109/TCBB.2021.3103280
Liu XP, Liu ZP, Zhao XM, et al., 2012, Identifying disease genes and module biomarkers by differential interactions. J Amer Med Inform Assoc, 19(2): 241–248. https://doi.org/10.1136/amiajnl-2011-000658
Liu XP, Chang X, Liu R, et al., 2017, Quantifying critical states of complex diseases using single-sample dynamic network biomarkers. PLoS Comput Biol, 13(7): e1005633. https://doi.org/10.1371/journal.pcbi.1005633
Tu JJ, Le OY, Yuan Z, et al., 2021, Differential network analysis by simultaneously considering changes in gene interactions and gene expression. Bioinformatics, 37(23): 4414–4423. https://doi.org/10.1093/bioinformatics/btab502
Hudson NJ, Reverter A, Dalrymple BP, 2009, A differential wiring analysis of expression data correctly identifies the gene containing the causal mutation. PLoS Comput Biol, 5(5): 1000382. https://doi.org/10.1371/journal.pcbi.1000382
Tian WD, Zhang LV, Tasan M, et al., 2008, Combining guilt-by-association and guilt-by-profiling to predict Saccharomyces cerevisiae gene function. Genome Biol, 9: S1. https://doi.org/10.1186/gb-2008-9-s1-s7
Shin H, Sheu B, Joseph M, et al., 2008, Guilt-by-association feature selection: Identifying biomarkers from proteomic profiles. J Biomed Inform, 41(1): 124–136. https://doi.org/10.1016/j.jbi.2007.04.003
Kitsak M, Gallos LK, Havlin S, et al., 2010, Identification of influential spreaders in complex networks. Nat Phys, 6(11): 888–893. https://doi.org/10.1038/NPHYS1746
Wang P, 2021, Statistical identification of important nodes in biological systems. J Syst Sci Complex, 34(4): 1454–1470. https://doi.org/10.1007/s11424-021-0001-2
Lü LY, Zhou T, Zhang QM, et al., 2016, The H-index of a network node and its relation to degree and coreness. Nat Commun, 7: 10168. https://doi.org/10.1038/ncomms10168
Koschützki D, Schwöbbermeyer H, Schreiber F, 2007, Ranking of network elements based on functional substructures. J Theor Biol, 248(3): 471–479. https://doi.org/10.1016/j.jtbi.2007.05.038
Rual JF, Venkatesan K, Hao T, et al., 2005, Towards a proteome-scale map of the human protein-protein interaction network. Nature, 437(7062): 1173–1178. https://doi.org/10.1038/nature04209
Cui Y, Cai M, Dai Y, et al., 2018, A hybrid network-based method for the detection of disease-related genes. Physica A, 492: 389–394. https://doi.org/10.1016/j.physa.2017.10.026
Milo R, Shen-Orr S, Itzkovitz S, et al., 2002, Network motifs: Simple building blocks of complex networks. Science, 298(5594): 824–827. https://doi.org/10.1126/science.298.5594.824
Shen-Orr SS, Milo R, Mangan S, et al., 2002, Network motifs in the transcriptional regulation network of Escherichia coli. Nat Genet, 31(1): 64–68. https://doi.org/10.1038/ng881
Louie B, Higdon R, Kolker E, 2009, A statistical model of protein sequence similarity and function similarity reveals overly-specific function predictions. PLoS One, 4(10): e7546. https://doi.org/10.1371/journal.pone.0007546
Watson JD, 2011, Molecular Biology of the Gene. London: Pearson.
Sharan R, Ulitsky I, Shamir R, 2007, Network-based prediction of protein function. Mol Syst Biol, 3: 88. https://doi.org/10.1038/msb4100129
Klie S, Nikoloski Z, Selbig J, 2014, Biological cluster evaluation for gene function prediction. J Comput Biol, 21(6): 428–445. https://doi.org/10.1089/cmb.2009.0129
Liu YY, Slotine JJ, Barabási AL, 2011, Controllability of complex networks. Nature, 473(7346): 167–173. https://doi.org/10.1038/nature10011
Wuchty S, 2014, Controllability in protein interaction networks. Proc Natl Acad Sci U S A, 111(19): 7156–7160. https://doi.org/10.1073/pnas.1311231111
Liu XM, Pan LQ, 2015, Identifying driver nodes in the human signaling network using structural controllability analysis. IEEE/ACM Trans Comput Biol Bioinform, 12(2): 467–472. https://doi.org/10.1109/TCBB.2014.2360396
Zhang XF, Le OY, Zhu Y, et al., 2015, Determining minimum set of driver nodes in protein-protein interaction networks. BMC Bioinformatics, 16: 146. https://doi.org/10.1186/s12859-015-0591-3
Vinayagam A, Gibson TE, Lee HJ, et al., 2016, Controllability analysis of the directed human protein interaction network identifies disease genes and drug targets. Proc Natl Acad Sci U S A, 113(18): 4976–4981. https://doi.org/10.1073/pnas.1603992113
Yan G, Vertes PE, Towlson EK, et al., 2017, Network control principles predict neuron function in the Caenorhabditis elegans connectome. Nature, 550(7677): 519–523. https://doi.org/10.1038/nature24056
Wang P, Wang DJ, Lü JH, 2019, Controllability analysis of a gene network for Arabidopsis thaliana reveals characteristics of functional gene families. IEEE/ACM Trans Comput Biol Bioinform, 16(3): 912–924. https://doi.org/10.1109/TCBB.2018.2821145
Guo WF, Zhang SW, Zeng T, et al., 2020, Network control principles for identifying personalized driver genes in cancer. Brief Bioinform, 21(5): 1641–1662. https://doi.org/10.1093/bib/bbz089
Zheng W, Wang DJ, Zou XF, 2019, Control of multilayer biological networks and applied to target identification of complex diseases. BMC Bioinformatics, 20: 271. https://doi.org/10.1186/s12859-019-2841-2
Subramanian A, Tamayo P, Mootha VK, et al., 2005, Gene set enrichment analysis: A knowledge-based approach for interpreting genome-wide expression profiles. Proc Natl Acad Sci U S A, 102(43): 15545–15550. https://doi.org/10.1073/pnas.0506580102
Subramanian A, Kuehn H, Gould J, et al., 2007, GSEA-P: A desktop application for gene set enrichment analysis. Bioinformatics, 23(23): 3251–3253. https://doi.org/10.1093/bioinformatics/btm369
Huang DW, Sherman BT Lempicki RA, 2009, Systematic and integrative analysis of large gene lists using DAVID bioinformatics resources. Nature Pro, 4(1): 44–57. https;//doi.org/10.1038/nprot.2008.211
Yu GC, Wang LG, Han YY, et al., 2012, ClusterProfiler: An R package for comparing biological themes among gene clusters. OMICS, 16(5): 284–287. https://doi.org/10.1089/omi.2011.0118
Zheng Q, Wang XJ, 2008, GOEAST: A web-based software toolkit for gene ontology enrichment analysis. Nucl Acids Res, 36: 358–363. https://doi.org/10.1093/nar/gkn276
Pawson T, Linding R, 2008, Network medicine. FEBS Lett, 582(8): 1266–1270. https://doi.org/10.1016/j.febslet.2008.02.011
Chen Y, Zhu J, Lum PY, et al., 2008, Variations in DNA elucidate molecular networks that cause disease. Nature, 452(7186): 429–435. https://doi.org/10.1038/nature06757
Valle I, Roweth HG, Malloy MW, et al., 2021, Network medicine framework shows that proximity of polyphenol targets and disease proteins predicts therapeutic effects of polyphenols. Nature Food, 2: 143–155. https://doi.org/10.1038/s43016-021-00243-7
Erler JT, Linding R, 2012, Network medicine strikes a blow against breast cancer. Cell, 149(4): 731–733. https://doi.org/10.1016/j.cell.2012.04.014
Lee MJ, Ye AS, Gardino AK, et al., 2012, Sequential application of anticancer drugs enhances cell death by rewiring apoptotic signaling networks. Cell, 149(4): 780–794. https://doi.org/10.1016/j.cell.2012.03.031
Cheng F, Desai RJ, Handy DE, et al., 2018, Network-based approach to prediction and population-based validation of in silico drug repurposing. Nat Commun, 9: 2691. https://doi.org/10.1038/s41467-018-05116-5
Cheng F, István A, Barabási AL, 2019, Network-based prediction of drug combinations. Nat Commun, 10: 1197. https://doi.org/10.1038/s41467-019-09186-x
Zhou Y, Hou Y, Shen J, et al., 2020, Network-based drug repurposing for novel coronavirus 2019-nCoV/SARS-CoV-2. Cell Discov, 6: 14. https://doi.org/10.1038/s41421-020-0153-3
Gysi DM, Valle TD, Zitnik M, et al., 2021, Network medicine framework for identifying drug-repurposing opportunities for COVID-19. Proc Natl Acad Sci. U S A, 118(19): e2025581118. https://doi.org/10.1073/pnas.2025581118
Stumpf MP, Wiuf C, May RM, 2005, Subnets of scale-free networks are not scale-free: Sampling properties of networks. Proc Natl Acad Sci U S A, 102(12): 4221–4224. https://doi.org/10.1073/pnas.0501179102
Sathyanarayanan A, Gupta R, Thompson EW, et al., 2020, A comparative study of multi-omics integration tools for cancer driver gene identification and tumour subtyping. Brief Bioinform, 21(6): 1920–1936. https://doi.org/10.1093/bib/bbz121
Zander M, Lewsey MG, Clark NM, et al., 2020, Publisher correction: Integrated multi-omics framework of the plant response to jasmonic acid. Nat Plants, 6(8): 1065–1065. https://doi.org/10.1038/s41477-020-0743-y
Bodein A, Scott-Boyer MP0, Perin O, et al., 2022, Interpretation of network-based integration from multi-omics longitudinal data. Nucl Acids Res, 50(5): e27. https://doi.org/10.1093/nar/gkab1200
Taylor KJ, Sims AH, Liang L, et al., 2010, Dynamic changes in gene expression in vivo predict prognosis of tamoxifen-treated patients with breast cancer. Breast Cancer Res, 12(3): R39. https://doi.org/10.1186/bcr2593
Alcalá-Corona SA, Sandoval-Motta S, Espinal-Enríquez J, et al., 2021, Modularity in biological networks. Front Genet, 12: 1708–1708. https://doi.org/10.3389/fgene.2021.701331
Mucha PJ, Richardson T, Macon K, et al., 2010, Community structure in time-dependent, multiscale, and multiplex networks. Science, 328(5980): 876–878. https://doi.10.1126/science.1184819
Agostino GD, Scala A, 2014, Networks of Networks: The Last Frontier of Complexity. Berlin: Springer. https://doi.org/10.1007/978-3-319-03518-5
Bianconi G, 2018, Multilayer Networks: Structure and Function. Oxford: Oxford University Press. https://doi.org/10.1093/oso/9780198753919.001.0001
Pilosof S, Porter MA, Pascual M, et al., 2017, The multilayer nature of ecological networks. Nat Ecol Evol, 1(4): 0101. https://doi.org/10.1038/s41559-017-0101
Liu XM, Maiorino E, Halu A, et al., 2020, Robustness and lethality in multilayer biological molecular networks. Nat Commun, 11(1): 6043. https://doi.org/10.1038/s41467-020-19841-3
Shang HX, Liu ZP, 2021, Prioritizing Type 2 diabetes genes by weighted PageRank on bilayer heterogeneous networks. IEEE/ACM Trans Comput Biol Bioinform, 18(1): 336–346. https://doi.org/10.1109/TCBB.2019.2917190
Shang HX, Liu ZP, 2020, Network-based prioritization of cancer genes by integrative ranks from multi-omics data. Comput Biol Med, 119: 103692. https://doi.org/10.1016/j.compbiomed.2020.103692
Li J, Zhao PX, 2016, Mining functional modules in heterogeneous biological networks using multiplex PageRank approach. Front Plant Sci, 7: 903. https://doi.org/10.3389/fpls.2016.00903