An ink-insensitive deep learning model for improving the printing quality in extrusion-based bioprinting
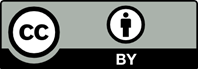
Extrusion-based bioprinting (EBB) is an efficient, simple, and cost-effective bioprinting technique. However, due to the high complexity of biomaterial inks, a trial-and-error approach is typically required for determining the optimal printing parameter configurations. Thus, the quality and reproducibility of printed scaffolds remain a concern. In this study, we integrated flow sensing into the EBB and replaced conventional fixed-value printing parameters by capturing the time-series data of all printing parameters to enhance the monitoring of the printing process. To improve the EBB adaptability, we conducted experiments with three biomaterial inks with distinct properties under various parameter configurations to achieve ink-insensitive linewidth prediction through the construction and assessment of deep learning models. Additionally, two explainable artificial intelligence methods were used to analyze the decision-making process of the deep learning model. This analysis not only enhanced model reliability but also identified key features in printing parameters and time-series data. These results can be used to improve the efficiency and quality of the EBB processes by using various biomaterial inks.

- Eyers DR, Potter AT. Industrial additive manufacturing: a manufacturing systems perspective. Comput Ind. 2017; 92-93:208-218. doi: 10.1016/j.compind.2017.08.002
- Culmone C, Smit G, Breedveld P. Additive manufacturing of medical instruments: a state-of-the-art review. Addit Manuf. 2019;27:461-473. doi: 10.1016/j.addma.2019.03.015
- Gu Z, Fu J, Lin H, He Y. Development of 3D bioprinting: from printing methods to biomedical applications. Asian J Pharma Sci. 2020;15(5):529-557. doi: 10.1016/j.ajps.2019.11.003
- Mir TA, Iwanaga S, Kurooka T, Toda H, Sakai S, Nakamura M. Biofabrication offers future hope for tackling various obstacles and challenges in tissue engineering and regenerative medicine: a perspective. IJB. 2018; 5(1):153. doi: 10.18063/ijb.v5i1.153
- Askari M, Afzali Naniz M, Kouhi M, Saberi A, Zolfagharian A, Bodaghi M. Recent progress in extrusion 3D bioprinting of hydrogel biomaterials for tissue regeneration: a comprehensive review with focus on advanced fabrication techniques. Biomater Sci. 2021;9(3):535-573. doi: 10.1039/D0BM00973C
- Wang Z, Abdulla R, Parker B, Samanipour R, Ghosh S, Kim K. A simple and high-resolution stereolithography-based 3D bioprinting system using visible light crosslinkable bioinks. Biofabrication. 2015;7(4):045009. doi: 10.1088/1758-5090/7/4/045009
- Gudapati H, Dey M, Ozbolat I. A comprehensive review on droplet-based bioprinting: past, present and future. Biomaterials. 2016;102:20-42. doi: 10.1016/j.biomaterials.2016.06.012
- Yusupov V, Churbanov S, Churbanova E, et al. Laser-induced forward transfer hydrogel printing: a defined route for highly controlled process. IJB. 2024;6(3):271. doi: 10.18063/ijb.v6i3.271
- Ozbolat IT, Hospodiuk M. Current advances and future perspectives in extrusion-based bioprinting. Biomaterials. 2016;76:321-343. doi: 10.1016/j.biomaterials.2015.10.076
- Ramesh S, Harrysson OLA, Rao PK, et al. Extrusion bioprinting: recent progress, challenges, and future opportunities. Bioprinting. 2021;21:e00116. doi: 10.1016/j.bprint.2020.e00116
- Badylak S, Freytes D, Gilbert T. Extracellular matrix as a biological scaffold material: structure and function. Acta Biomater. 2009;5(1):1-13. doi: 10.1016/j.actbio.2008.09.013
- Choi DJ, Park SJ, Gu BK, Kim YJ, Chung S, Kim CH. Effect of the pore size in a 3D bioprinted gelatin scaffold on fibroblast proliferation. J Ind Eng Chem. 2018;67:388-395. doi: 10.1016/j.jiec.2018.07.013
- Jiang S, Li SC, Huang C, Chan BP, Du Y. Physical properties of implanted porous bioscaffolds regulate skin repair: focusing on mechanical and structural features. Adv Healthc Mater. 2018;7(6):1700894. doi: 10.1002/adhm.201700894
- Danilevicius P, Georgiadi L, Pateman CJ, Claeyssens F, Chatzinikolaidou M, Farsari M. The effect of porosity on cell ingrowth into accurately defined, laser-made, polylactide-based 3D scaffolds. Appl Surf Sci. 2015;336:2-10. doi: 10.1016/j.apsusc.2014.06.012
- Nosrati H, Aramideh Khouy R, Nosrati A, et al. Nanocomposite scaffolds for accelerating chronic wound healing by enhancing angiogenesis. J Nanobiotechnol. 2021;19(1):1. doi: 10.1186/s12951-020-00755-7
- Gerdes S, Ramesh S, Mostafavi A, Tamayol A, Rivero IV, Rao P. Extrusion-based 3D (Bio)printed tissue engineering scaffolds: process–structure–quality relationships. ACS Biomater Sci Eng. 2021;7(10):4694-4717. doi: 10.1021/acsbiomaterials.1c00598
- Tian S, Zhao H, Lewinski N. Key parameters and applications of extrusion-based bioprinting. Bioprinting. 2021; 23:e00156. doi: 10.1016/j.bprint.2021.e00156
- He Y, Yang F, Zhao H, Gao Q, Xia B, Fu J. Research on the printability of hydrogels in 3D bioprinting. Sci Rep. 2016;6(1):29977. doi: 10.1038/srep29977
- Singh YP, Bandyopadhyay A, Mandal BB. 3D bioprinting using cross-linker-free silk–gelatin bioink for cartilage tissue engineering. ACS Appl Mater Interfaces. 2019; 11(37):33684-33696. doi: 10.1021/acsami.9b11644
- Barros NR, Kim HJ, Gouidie MJ, et al. Biofabrication of endothelial cell, dermal fibroblast, and multilayered keratinocyte layers for skin tissue engineering. Biofabrication. 2021;13(3):035030. doi: 10.1088/1758-5090/aba503
- Gharraei R, Bergstrom DJ, Chen X. Extrusion bioprinting from a fluid mechanics perspective. IJB. 2024; 10(6):3973. doi: 10.36922/ijb.3973
- Wang YJ, Jeng US, Hsu SH. Biodegradable water-based polyurethane shape memory elastomers for bone tissue engineering. ACS Biomater Sci Eng. 2018;4(4):1397-1406. doi: 10.1021/acsbiomaterials.8b00091
- Markstedt K, Mantas A, Tournier I, Martínez Ávila H, Hägg D, Gatenholm P. 3D bioprinting human chondrocytes with nanocellulose–alginate bioink for cartilage tissue engineering applications. Biomacromolecules. 2015;16(5):1489-1496. doi: 10.1021/acs.biomac.5b00188
- Webb B, Doyle BJ. Parameter optimization for 3D bioprinting of hydrogels. Bioprinting. 2017;8:8-12. doi: 10.1016/j.bprint.2017.09.001
- Trachtenberg JE, Placone JK, Smith BT, et al. Extrusion-based 3D printing of poly(propylene fumarate) in a full-factorial design. ACS Biomater Sci Eng. 2016;2(10):1771-1780. doi: 10.1021/acsbiomaterials.6b00026
- Armstrong AA, Norato J, Alleyne AG, Wagoner Johnson AJ. Direct process feedback in extrusion-based 3D bioprinting. Biofabrication. 2019;12(1):015017. doi: 10.1088/1758-5090/ab4d97
- Wang L, Xu M-E, Luo L, Zhou Y, Si P. Iterative feedback bio-printing-derived cell-laden hydrogel scaffolds with optimal geometrical fidelity and cellular controllability. Sci Rep. 2018;8(1):2802. doi: 10.1038/s41598-018-21274-4
- Tseng WC, Liao CY, Chassagne L, Cagneau B. Assessment of a flow-measurement technique for the printability of extrusion-based bioprinting. IEEE Robot Autom Lett. 2024;9(2):1716-1723. doi: 10.1109/LRA.2024.3349972
- Wenger L, Strauß S, Hubbuch J. Automated and dynamic extrusion pressure adjustment based on real-time flow rate measurements for precise ink dispensing in 3D bioprinting. Bioprinting. 2022;28:e00229. doi: 10.1016/j.bprint.2022.e00229
- Brion DAJ, Pattinson SW. Quantitative and real‐time control of 3d printing material flow through deep learning. Adv Intell Syst. 2022;4(11):2200153. doi: 10.1002/aisy.202200153
- Bonatti AF, Vozzi G, Chua CK, Maria CD. A deep learning quality control loop of the extrusion-based bioprinting process. IJB. 2022;8(4):620. doi: 10.18063/ijb.v8i4.620
- Fu Z, Angeline V, Sun W. Evaluation of printing parameters on 3D extrusion printing of pluronic hydrogels and machine learning guided parameter recommendation. IJB. 2024;7(4):434. doi: 10.18063/ijb.v7i4.434
- Ruberu K, Senadeera M, Rana S, et al. Coupling machine learning with 3D bioprinting to fast track optimisation of extrusion printing. Appl Mater Today. 2021;22:100914. doi: 10.1016/j.apmt.2020.100914
- Weeks RD, Ruddock JM, Berrigan JD, Lewis JA, Hardin James O. In‐situ rheology measurements via machine‐learning enhanced direct‐ink‐writing. Adv Intell Syst. 2024:2400293. doi: 10.1002/aisy.202400293
- Angelov PP, Soares EA, Jiang R, Arnold NI, Atkinson PM. Explainable artificial intelligence: an analytical review. WIREs Data Min Knowl. 2021;11(5):e1424. doi: 10.1002/widm.1424
- Hassija V, Chamola V, Mahapatra A, et al. Interpreting Black- Box models: a review on explainable artificial intelligence. Cogn Comput. 2024;16(1):45-74. doi: 10.1007/s12559-023-10179-8
- Hochreiter S, Schmidhuber J. Long short-term memory. Neural Comput. 1997;9(8):1735-1780. doi: 10.1162/neco.1997.9.8.1735
- Bai S, Kolter JZ, Koltun V. An Empirical Evaluation of Generic Convolutional and Recurrent Networks for Sequence Modeling. 2018. Accessed November 20, 2024. http://arxiv.org/abs/1803.01271
- Rajabi M, McConnell M, Cabral J, Ali MA. Chitosan hydrogels in 3D printing for biomedical applications. Carbohydr Polym. 2021;260:117768. doi: 10.1016/j.carbpol.2021.117768
- Huang J, Huang Z, Liang Y, et al. 3D printed gelatin/ hydroxyapatite scaffolds for stem cell chondrogenic differentiation and articular cartilage repair. Biomater Sci. 2021;9(7):2620-2630. doi: 10.1039/D0BM02103B
- Song P, Li M, Zhang B, et al. DLP fabricating of precision GelMA/HAp porous composite scaffold for bone tissue engineering application. Compos B: Eng. 2022;244: 110163. doi: 10.1016/j.compositesb.2022.110163
- Dos Santos Carvalho JD, Rabelo RS, Hubinger MD. Thermo-rheological properties of chitosan hydrogels with hydroxypropyl methylcellulose and methylcellulose. Int J Biol Macromol. 2022;209:367-375.doi: 10.1016/j.ijbiomac.2022.04.035
- Liao CY, Wu WJ, Hsieh CT, Tseng CS, Dai NT, Hsu SH. Design and development of a novel frozen-form additive manufacturing system for tissue engineering applications. 3D Print Addit Manuf. 2016;3(4):216-225. doi: 10.1089/3dp.2015.0042
- Lundberg S, Lee SI. A Unified Approach to Interpreting Model Predictions; 2017. Accessed November 20, 2024. http://arxiv.org/abs/1705.07874
- Fisher A, Rudin C, Dominici F. All Models Are Wrong, but Many Are Useful: Learning a Variable’s Importance by Studying an Entire Class of Prediction Models Simultaneously; 2019. Accessed November 20, 2024. http://arxiv.org/abs/1801.01489
- Ran J, Hu J, Chen L, Shen X, Tong H. Preparation and characterization of gelatin/hydroxyapatite nanocomposite for bone tissue engineering. Polym Compos. 2017;38(8): 1579-1590. doi: 10.1002/pc.23725
- Parker NG, Povey MJW. Ultrasonic study of the gelation of gelatin: phase diagram, hysteresis and kinetics. Food Hydrocolloids. 2012;26(1):99-107. doi: 10.1016/j.foodhyd.2011.04.016