Deep learning for polymer scaffold bioimage analysis: Opportunities and challenges
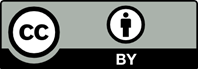
Significant efforts have been made to advance bioprinted scaffold research in cell biology, tissue engineering, and drug screening studies. Ideal scaffolds should demonstrate suitable mechanical properties, excellent biocompatibility, and bioactivities. However, the design and preparation of such scaffolds are challenging. Imaging modalities, including magnetic resonance imaging, micro-computed tomography, ultrasound imaging, optical coherence tomography, and confocal laser scanning microscopy, are commonly used to visualize the interior architecture of bioprinted scaffolds, as well as the surrounding cells and tissues. The obtained bioimages provide direct insight into the biological functionalities of the scaffold, though their interpretation may lead to differing viewpoints and even debates. This review explores deep learning (DL) methods employed for image analysis, including restoration, segmentation, and classification. First, current DL methods for biological image processing are summarized, such as convolutional neural network, U-Net, and generative adversarial network. The corresponding outcomes of these methods reveal cell–scaffold and tissue–scaffold interactions, providing guidance for scaffold design in specific applications. Thereafter, the challenges and limitations of DL applications are highlighted, such as building DL models using smaller bioimage datasets, interpreting DL models, vision-language model-guided bioimage analysis, and developing intelligent analysis platforms. Hence, this review would mark a paradigm shift in polymer scaffold designs and the associated performance.

- Jing L, Wang X, Leng B, et al. Engineered nanotopography on the microfibers of 3D-printed PCL scaffolds to modulate cellular responses and establish an in vitro tumor model. ACS Appl Bio Mater. 2021;4(2):1381-1394. doi: 10.1021/acsabm.0c01243
- Tian S, Stevens R, McInnes BT, Lewinski NA. Machine assisted experimentation of extrusion-based bioprinting systems. Micromachines (Basel). 2021;12(7):780. doi: 10.3390/mi12070780
- Ritzau-Reid KI, Callens SJP, Xie R, et al. Microfibrous scaffolds guide stem cell lumenogenesis and brain organoid engineering. Adv Mater. 2023;35(41):e2300305. doi: 10.1002/adma.202300305
- Sun J, Vijayavenkataraman S, Liu H. An overview of scaffold design and fabrication technology for engineered knee meniscus. Materials (Basel). 2017;10(1):29. doi: 10.3390/ma10010029
- Gupta V, Khan Y, Berkland CJ, Laurencin CT, Detamore MS. Microsphere-based scaffolds in regenerative engineering. Annu Rev Biomed Eng. 2017;19:135-161. doi: 10.1146/annurev-bioeng-071516-044712
- Shepherd DV, Shepherd JH, Best SM, Cameron RE. 3D imaging of cells in scaffolds: direct labelling for micro CT. J Mater Sci Mater Med. 2018;29(6):86. doi: 10.1007/s10856-018-6089-6
- Finamore TA, Curtis TE, Tedesco JV, Grandfield K, Roeder RK. Nondestructive, longitudinal measurement of collagen scaffold degradation using computed tomography and gold nanoparticles. Nanoscale. 2019;11(10):4345-4354. doi: 10.1039/c9nr00313d
- Zielińska A, Karczewski J, Eder P, et al. Scaffolds for drug delivery and tissue engineering: the role of genetics. J Control Release. 2023;359:207-223. doi: 10.1016/j.jconrel.2023.05.042
- Mertens ME, Hermann A, Bühren A, et al. Iron oxide-labelled collagen scaffolds for non-invasive MR imaging in tissue engineering. Adv Funct Mater. 2014;24(6): 754-762. doi: 10.1002/adfm.201301275
- Farooq V, Onuma Y, Radu M, et al. Optical coherence tomography (OCT) of overlapping bioresorbable scaffolds: from benchwork to clinical application. EuroIntervention. 2011;7(3):386-399. doi: 10.4244/EIJV7I3A64
- Holmes C, Tabrizian M, Bagnaninchi PO. Motility imaging via optical coherence phase microscopy enables label-free monitoring of tissue growth and viability in 3D tissue-engineering scaffolds. J Tissue Eng Regen Med. 2015;9(5):641-645. doi: 10.1002/term.1687
- Chen J, Huang D, Wang L, et al. 3D bioprinted multiscale composite scaffolds based on gelatin methacryloyl (GelMA)/ chitosan microspheres as a modular bioink for enhancing 3D neurite outgrowth and elongation. J Colloid Interface Sci. 2020;574:162-173. doi: 10.1016/j.jcis.2020.04.040
- Serpooshan V, Zhao M, Metzler SA, et al. The effect of bioengineered acellular collagen patch on cardiac remodeling and ventricular function post myocardial infarction. Biomaterials. 2013;34(36):9048-9055. doi: 10.1016/j.biomaterials.2013.08.017
- Loai S, Szulc DA, Cheng HM. Three-dimensional bioprinted MR-trackable regenerative scaffold for postimplantation monitoring on T1-weighted MRI. J Magn Reson Imaging. 2022;56(2):570-578. doi: 10.1002/jmri.28057
- Cunha FB, Pomini KT, Plepis AMG, et al. In vivo biological behavior of polymer scaffolds of natural origin in the bone repair process. Molecules. 2021;26(6):1598. doi: 10.3390/molecules26061598
- Sun Y, Responte D, Xie H, et al. Nondestructive evaluation of tissue engineered articular cartilage using time-resolved fluorescence spectroscopy and ultrasound backscatter microscopy. Tissue Eng Part C Methods. 2012; 18(3):215-226. doi: 10.1089/ten.TEC.2011.0343
- Mueller KMA, Topping GJ, Schwaminger SP, et al. Visualization of USPIO-labelled melt-electrowritten scaffolds by non-invasive magnetic resonance imaging. Biomater Sci. 2021;9(13):4607-4612. doi: 10.1039/d1bm00461a
- Chen W, Yang J, Liao W, et al. In vitro remodeling and structural characterization of degradable polymer scaffold-based tissue-engineered vascular grafts using optical coherence tomography. Cell Tissue Res. 2017; 370(3):417-426. doi: 10.1007/s00441-017-2683-z
- Yao K, Sun J, Huang K, et al. Analyzing cell-scaffold interaction through unsupervised 3D nuclei segmentation. Int J Bioprint. 2021;8(1):495. doi: 10.18063/ijb.v8i1.495
- Monllau JC, Poggioli F, Erquicia J, et al. Magnetic resonance imaging and functional outcomes after a polyurethane meniscal scaffold implantation: minimum 5-year follow-up. Arthroscopy. 2018;34(5):1621-1627. doi: 10.1016/j.arthro.2017.12.019
- Li X, Zou Q, Wei J, et al. The degradation regulation of 3D printed scaffolds for promotion of osteogenesis and in vivo tracking. Compos B Eng. 2021;222:109084. doi: 10.1016/j.compositesb.2021.109084
- Talukdar Y, Avti P, Sun J, Sitharaman B. Multimodal ultrasound-photoacoustic imaging of tissue engineering scaffolds and blood oxygen saturation in and around the scaffolds. Tissue Eng Part C Methods. 2014;20(5): 440-449. doi: 10.1089/ten.TEC.2013.0203
- Wang L, Xu ME, Luo L, Zhou Y, Si P. Iterative feedback bio-printing-derived cell-laden hydrogel scaffolds with optimal geometrical fidelity and cellular controllability. Sci Rep. 2018;8(1):2802. doi: 10.1038/s41598-018-21274-4
- Theus AS, Ning L, Kabboul G, et al. 3D bioprinting of nanoparticle-laden hydrogel scaffolds with enhanced antibacterial and imaging properties. iScience. 2022;25(9): 104947. doi: 10.1016/j.isci.2022.104947
- Li L, Gil CJ, Finamore TA, et al. Methacrylate-modified gold nanoparticles enable non-invasive monitoring of photocrosslinked hydrogel scaffolds. Adv Nanobiomed Res. 2022;2(7):2200022. doi: 10.1002/anbr.202200022
- Mercado KP, Helguera M, Hocking DC, Dalecki D. Estimating cell concentration in three-dimensional engineered tissues using high frequency quantitative ultrasound. Ann Biomed Eng. 2014;42(6):1292-1304. doi: 10.1007/s10439-014-0994-8
- Rivero RE, Capella V, Cecilia Liaudat A, et al. Mechanical and physicochemical behavior of a 3D hydrogel scaffold during cell growth and proliferation. RSC Adv. 2020;10(10):5827-5837. doi: 10.1039/c9ra08162c
- Sandker MJ, Petit A, Redout EM, et al. In situ forming acyl-capped PCLA-PEG-PCLA triblock copolymer based hydrogels. Biomaterials. 2013;34(32):8002-8011. doi: 10.1016/j.biomaterials.2013.07.046
- Zhu W, Chu C, Kuddannaya S, et al. In vivo imaging of composite hydrogel scaffold degradation using CEST MRI and two-color NIR imaging. Adv Funct Mater. 2019;29(36):1903753. doi: 10.1002/adfm.201903753
- Leng X, Liu B, Su B, et al. In situ ultrasound imaging of silk hydrogel degradation and neovascularization. J Tissue Eng Regen Med. 2017;11(3):822-830. doi: 10.1002/term.1981
- Yao K, Huang K, Sun J, et al. Scaffold-A549: a benchmark 3D fluorescence image dataset for unsupervised nuclei segmentation. Cogn Comput. 2021;13:1603-1608. doi: 10.1007/s12559-021-09944-4
- Rafieyan S, Vasheghani-Farahani E, Baheiraei N, Keshavarz H. MLATE: machine learning for predicting cell behavior on cardiac tissue engineering scaffolds. Comput Biol Med. 2023;158:106804. doi: 10.1016/j.compbiomed.2023.106804
- Huang K, Hussain A, Wang, Q, et al. Deep Learning: Fundamentals, Theory and Applications. Springer; 2019:2. ISBN:3030060721. doi: 10.1007/978-3-030-06073-2
- You S, Fan Y, Chen Y, et al. Advancements and prospects of deep learning in biomaterials evolution. Cell Rep Phys Sci. 2024;5(9):102116. doi: 10.1016/j.xcrp.2024.102116
- Huang J, Yang G, Li B, et al. Segmentation of cervical cell images based on generative adversarial networks. IEEE Access. 2021;9:115415-115428. doi: 10.1109/access.2021.3104609
- Niioka H, Asatani S, Yoshimura A, Ohigashi H, Tagawa S, Miyake J. Classification of C2C12 cells at differentiation by convolutional neural network of deep learning using phase contrast images. Hum Cell. 2018;31(1):87-93. doi: 10.1007/s13577-017-0191-9
- Appel AA, Anastasio MA, Larson JC, Brey EM. Imaging challenges in biomaterials and tissue engineering. Biomaterials. 2013;34(28):6615-6630. doi: 10.1016/j.biomaterials.2013.05.033
- Nam SY, Ricles LM, Suggs LJ, Emelianov SY. Imaging strategies for tissue engineering applications. Tissue Eng Part B Rev. 2015;21(1):88-102. doi: 10.1089/ten.TEB.2014.0180
- Voigt SP, Ravikumar K, Basu B, et al. Automated image processing workflow for morphological analysis of fluorescence microscopy cell images. JOM. 2021;73(8):2356-2365. doi: 10.1007/s11837-021-04707-w
- Kérourédan O, Ribot EJ, Fricain JC, Devillard R, Miraux S. Magnetic resonance imaging for tracking cellular patterns obtained by laser-assisted bioprinting. Sci Rep. 2018;8(1):15777. doi: 10.1038/s41598-018-34226-9
- Catanzaro V, Digilio G, Capuana F, et al. Gadolinium-labelled cell scaffolds to follow-up cell transplantation by magnetic resonance imaging. J Funct Biomater. 2019;10(3):28. doi: 10.3390/jfb10030028
- Constantinides C, Basnett P, Lukasiewicz B, et al. In vivo tracking and 1H/19F magnetic resonance imaging of biodegradable polyhydroxyalkanoate/polycaprolactone blend scaffolds seeded with labelled cardiac stem cells. ACS Appl Mater Interfaces. 2018;10(30):25056-25068. doi: 10.1021/acsami.8b06096
- El-Fiqi A, Kim JH, Kim HW. Novel bone-mimetic nanohydroxyapatite/collagen porous scaffolds biomimetically mineralized from surface silanized mesoporous nanobioglass/collagen hybrid scaffold: physicochemical, mechanical and in vivo evaluations. Mater Sci Eng C Mater Biol Appl. 2020;110:110660. doi: 10.1016/j.msec.2020.110660
- Palmroth A, Pitkänen S, Hannula M, et al. Evaluation of scaffold microstructure and comparison of cell seeding methods using micro-computed tomography-based tools. J R Soc Interface. 2020;17(165):20200102. doi: 10.1098/rsif.2020.0102
- Ning L, Zhu N, Smith A, et al. Noninvasive three-dimensional in situ and in vivo characterization of bioprinted hydrogel scaffolds using the X-ray propagation-based imaging technique. ACS Appl Mater Interfaces. 2021;13(22):25611-25623. doi: 10.1021/acsami.1c02297
- Zhou Y. The application of ultrasound in 3D bio-printing. Molecules. 2016;21(5):590. doi: 10.3390/molecules21050590
- Deng CX, Hong X, Stegemann JP. Ultrasound imaging techniques for spatiotemporal characterization of composition, microstructure, and mechanical properties in tissue engineering. Tissue Eng Part B Rev. 2016;22(4):311-321. doi: 10.1089/ten.TEB.2015.0453
- Chiu YC, Brey EM, Pérez-Luna VH. A study of the intrinsic autofluorescence of poly (ethylene glycol)-co-(L-lactic acid) diacrylate. J Fluoresc. 2012;22(3):907-913. doi: 10.1007/s10895-011-1029-6
- Boutin ME, Voss TC, Titus SA, Cruz-Gutierrez K, Michael S, Ferrer M. A high-throughput imaging and nuclear segmentation analysis protocol for cleared 3D culture models. Sci Rep. 2018;8(1):11135. doi: 10.1038/s41598-018-29169-0
- Sun J, Yao K, An J, Jing L, Huang K, Huang D. Machine learning and 3D bioprinting. Int J Bioprint. 2023;9(4):717. doi: 10.18063/ijb.717
- Malatesta M. Transmission electron microscopy as a powerful tool to investigate the interaction of nanoparticles with subcellular structures. Int J Mol Sci. 2021;22(23):12789. doi: 10.3390/ijms222312789
- Younesi M, Islam A, Kishore V, Anderson JM, Akkus O. Tenogenic induction of human MSCs by anisotropically aligned collagen biotextiles. Adv Funct Mater 2014;24(36):5762-5770. doi: 10.1002/adfm.201400828
- Iandolo D, Pennacchio FA, Mollo V, et al. Electron microscopy for 3D scaffolds-cell biointerface characterization. Adv Biosyst. 2019;3(2):e1800103. doi: 10.1002/adbi.201800103
- Peddie CJ, Genoud C, Kreshuk A, et al. Volume electron microscopy. Nat Rev Methods Primers. 2022;2:51. doi: 10.1038/s43586-022-00131-9
- Guaccio A, Guarino V, Perez MA, Cirillo V, Netti PA, Ambrosio L. Influence of electrospun fiber mesh size on hMSC oxygen metabolism in 3D collagen matrices: experimental and theoretical evidences. Biotechnol Bioeng. 2011;108(8):1965-1976. doi: 10.1002/bit.23113
- McQuin C, Goodman A, Chernyshev V, et al. CellProfiler 3.0: next-generation image processing for biology. PLoS Biol. 2018;16(7):e2005970. doi: 10.1371/journal.pbio.2005970
- Gómez-de-Mariscal E, García-López-de-Haro C, Ouyang W, et al. DeepImageJ: a user-friendly environment to run deep learning models in ImageJ. Nat Methods. 2021;18(10): 1192-1195. doi: 10.1038/s41592-021-01262-9
- Berg S, Kutra D, Kroeger T, et al. Ilastik: interactive machine learning for (bio)image analysis. Nat Methods. 2019;16(12):1226-1232. doi: 10.1038/s41592-019-0582-9
- Goodfellow I, Pouget-Abadie J, Mirza M, et al. Generative adversarial nets. In: Advances in Neural Information Processing Systems;2014:27. ISBN:9781510800410. doi: 10.1145/3422622
- Zhang S, Qian Z, Huang K, et al. Robust generative adversarial network. Mach Learn. 2023;112(12):5135-5161. doi: 10.1007/s10994-023-06367-0.
- Shahin AI, Guo Y, Amin KM, Sharawi AA. White blood cells identification system based on convolutional deep neural learning networks. Comput Methods Programs Biomed. 2019;168:69-80. doi: 10.1016/j.cmpb.2017.11.015
- Sun J, Jing L, Fan X, Gao X, Liang YC. Electrohydrodynamic printing process monitoring by microscopic image identification. Int J Bioprint. 2018;5(1):164. doi: 10.18063/ijb.v5i1.164.
- Zheng Z, Sun B, He S, et al. Unsupervised deep learning of bright-field images for apoptotic cell classification. SIViP. 2023;17:3657-3664. doi: 10.1007/s11760-023-02592-1
- Ronneberger O, Fischer P, Brox T. U-net: convolutional networks for biomedical image segmentation. MICCAI. 2015;9351:234-241. doi: 10.1007/978-3-319-24574-4_28
- Pfister M, Schützenberger K, Pfeiffenberger U, et al. Automated segmentation of dermal fillers in OCT images of mice using convolutional neural networks. Biomed Opt Express. 2019;10(3):1315-1328. doi: 10.1364/boe.10.001315
- Lugagne JB, Lin H, Dunlop MJ. DeLTA: automated cell segmentation, tracking, and lineage reconstruction using deep learning. PLoS Comput Biol. 2020;16(4):e1007673. doi: 10.1371/journal.pcbi.1007673
- Rautaray SS, Dey S, Pandey M, et al. Nuclei segmentation in cell images using fully convolutional neural networks. Int J Emerg Technol. 2020;11(3):731-737.
- Yao K, Rochman ND, Sun SX. Cell type classification and unsupervised morphological phenotyping from low-resolution images using deep learning. Sci Rep. 2019;9(1): 13467. doi: 10.1038/s41598-019-50010-9
- Jin J, Lu JQ, Wen Y, Tian P, Hu XH. Deep learning of diffraction image patterns for accurate classification of five cell types. J Biophotonics. 2020;13(3):e201900242. doi: 10.1002/jbio.201900242
- Wang H, Rivenson Y, Jin Y, et al. Deep learning enables cross-modality super-resolution in fluorescence microscopy. Nat Methods. 2019;16(1):103-110. doi: 10.1038/s41592-018-0239-0
- Bailo O, Ham DS, Min Shin Y. Red blood cell image generation for data augmentation using conditional generative adversarial networks. In: Proceedings of the IEEE/ CVF Conference on Computer Vision and Pattern Recognition Workshops; 2019: 1039-1048. doi: 10.1109/CVPRW.2019.00136
- Moen E, Bannon D, Kudo T, Graf W, Covert M, Van Valen D. Deep learning for cellular image analysis. Nat Methods. 2019;16(12):1233-1246. doi: 10.1038/s41592-019-0403-1
- Al-Kofahi Y, Zaltsman A, Graves R, Marshall W, Rusu M. A deep learning-based algorithm for 2-D cell segmentation in microscopy images. BMC Bioinformatics. 2018;19(1):365. doi: 10.1186/s12859-018-2375-z
- Lux F, Matula P. DIC image segmentation of dense cell populations by combining deep learning and watershed. In: IEEE 16th International Symposium on Biomedical Imaging; 2019: 236-239. doi: 10.1109/ISBI.2019.8759594
- Inés A, Domínguez C, Heras J, Mata E, Pascual V. Biomedical image classification made easier thanks to transfer and semi-supervised learning. Comput Methods Programs Biomed. 2021;198:105782. doi: 10.1016/j.cmpb.2020.105782
- Laine RF, Jacquemet G, Krull A. Imaging in focus: an introduction to denoising bioimages in the era of deep learning. Int J Biochem Cell Biol. 2021;140:106077. doi: 10.1016/j.biocel.2021.106077
- Li X, Li Y, Zhou Y, et al. Real-time denoising enables high-sensitivity fluorescence time-lapse imaging beyond the shot-noise limit. Nat Biotechnol. 2023;41(2):282-292. doi: 10.1038/s41587-022-01450-8
- Rizk A, Paul G, Incardona P, et al. Segmentation and quantification of subcellular structures in fluorescence microscopy images using Squassh. Nat Protoc. 2014;9(3): 586-596. doi: 10.1038/nprot.2014.037
- Wen T, Tong B, Liu Y, et al. Review of research on the instance segmentation of cell images. Comput Methods Programs Biomed. 2022;227:107211. doi: 10.1016/j.cmpb.2022.107211
- Wen C, Miura T, Fujie Y, et al. Deep-learning-based flexible pipeline for segmenting and tracking cells in 3D image time series for whole brain imaging. bioRxiv. 2018. doi: 10.1101/385567
- Wu C, Entezari A, Zheng K, et al. A machine learning-based multiscale model to predict bone formation in scaffolds. Nat Comput Sci. 2021;1(8):532-541. doi: 10.1038/s43588-021-00115-x
- Wang Y, Mao H, Yi Z. Stem cell motion-tracking by using deep neural networks with multi-output[J]. Neural Comput Appl. 2019;31:3455-3467. doi: 10.1007/s00521-017-3291-2
- Helaehil JV, Lourenço CB, Huang B, et al. In vivo investigation of polymer-ceramic PCL/HA and PCL/β-TCP 3D composite scaffolds and electrical stimulation for bone regeneration. Polymers (Basel). 2021;14(1):65. doi: 10.3390/polym14010065
- Su Z, Yao K, Yang X, et al. Rethinking data augmentation for single-source domain generalization in medical image segmentation. Proc AAAI Conf Artif Intell. 2023;37(2):2366-2374. doi: 10.1609/aaai.v37i2.25332
- Hassija V, Chamola V, Mahapatra A, et al. Interpreting black-box models: a review on explainable artificial intelligence. Cogn Comput. 2024;16(1):45-74. doi: 10.1007/s12559-023-10179-8
- Achiam J, Adler S, Agarwal S, et al. Gpt-4 technical report. arXiv preprint arXiv:2303.08774. doi: 10.48550/arXiv.2303.08774
- Luo R, Sun L, Xia Y, et al. BioGPT: generative pre-trained transformer for biomedical text generation and mining. Brief Bioinform. 2022;23(6):bbac409. doi: 10.1093/bib/bbac409