Machine learning boosts three-dimensional bioprinting
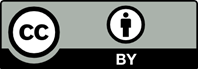
Correction for this article is available: view correction
Three-dimensional (3D) bioprinting is a computer-controlled technology that combines biological factors and bioinks to print an accurate 3D structure in a layerby-layer fashion. 3D bioprinting is a new tissue engineering technology based on rapid prototyping and additive manufacturing technology, combined with various disciplines. In addition to the problems in in vitro culture process, the bioprinting procedure is also afflicted with a few issues: (1) difficulty in looking for the appropriate bioink to match the printing parameters to reduce cell damage and mortality; and (2) difficulty in improving the printing accuracy in the printing process. Datadriven machine learning algorithms with powerful predictive capabilities have natural advantages in behavior prediction and new model exploration. Combining machine learning algorithms with 3D bioprinting helps to find more efficient bioinks, determine printing parameters, and detect defects in the printing process. This paper introduces several machine learning algorithms in detail, summarizes the role of machine learning in additive manufacturing applications, and reviews the research progress of the combination of 3D bioprinting and machine learning in recent years, especially the improvement of bioink generation, the optimization of printing parameter, and the detection of printing defect.
Xiaoya Z, Liuchao J,Jingchao J, 2022, A survey of additive manufacturing reviews. MSAM, 1(4):21.
Jiang J, 2020, A novel fabrication strategy for additive manufacturing processes. J Clean Prod, 272:122916. https://doi.org/10.1016/j.jclepro.2020.122916
Al-Kharusi G, Dunne NJ, Little S, et al., 2022, The role of machine learning and design of experiments in the advancement of biomaterial and tissue engineering research. Bioengineering, 9(10):561.
Gholami P, Ahmadi-pajouh MA, Abolftahi N, et al., 2018, Segmentation and measurement of chronic wounds for bioprinting. IEEE J Biomed Health, 22(4):1269–1277. https://doi.org/10.1109/JBHI.2017.2743526
Shin J, Lee Y, Li Z, et al., 2022, Optimized 3D bioprinting technology based on machine learning: A review of recent trends and advances. Micromachines, 13(3):363.
Ravanbakhsh H, Karamzadeh V, Bao G, et al., 2021, Emerging technologies in multi-material bioprinting. Adv Mater, 33(49):2104730. https://doi.org/10.1002/adma.202104730
Malekpour A, Chen X, 2022, Printability and cell viability in extrusion-based bioprinting from experimental, computational, and machine learning views. J Funct Biomater, 13(2):40.
Ong CS, Yesantharao P, Huang CY, et al., 2018, 3D bioprinting using stem cells. Pediatr Res, 83(1):223–231. https://doi.org/10.1038/pr.2017.252
Sklare SC, Richey WL, Vinson BT, et al., 2017, Directed self-assembly software for single cell deposition. Int J Bioprint, 3(2):100–108.
Datta P, Barui A, Wu Y, et al., 2018, Essential steps in bioprinting: From pre- to post-bioprinting. Biotechnol Adv, 36(5):1481–1504. https://doi.org/10.1016/j.biotechadv.2018.06.003
Zolfagharian A, Denk M, Kouzani AZ, et al., 2020, Effects of topology optimization in multimaterial 3D bioprinting of soft actuators. Int J Bioprint, 6(2):50–60.
Ng WL, Chan A, Ong YS, et al., 2020, Deep learning for fabrication and maturation of 3D bioprinted tissues and organs. Virtual Phys Prototyp, 15(3):340–358. https://doi.org/10.1080/17452759.2020.1771741
He H, Yang Y, Pan Y, 2019, Machine learning for continuous liquid interface production: Printing speed modelling. J Manuf Syst, 50:236–246. https://doi.org/10.1016/j.jmsy.2019.01.004
Yu C, Jiang J, 2020, A perspective on using machine learning in 3D bioprinting. Int J Bioprint, 6(1):1–8.
Freeman S, Calabro S, Williams R, et al., 2022, Bioink formulation and machine learning-empowered bioprinting optimization. Front Bioeng Biotechnol, 10:913579.
Jin Z, Zhang Z, Demir K, et al., 2020, Machine learning for advanced additive manufacturing. Matter, 3(5): 1541–1556. https://doi.org/10.1016/j.matt.2020.08.023
Omairi A, Ismail Z H, 2021, Towards machine learning for error compensation in additive manufacturing[J]. Appl Sci, 11(5):2375. https://doi.org/10.3390/app11052375
Qin J, Hu F, Liu Y, et al., 2022, Research and application of machine learning for additive manufacturing. Addit Manuf, 52:102691. https://doi.org/10.1016/j.addma.2022.102691
Jiang J, Xu X, Xiong Y, et al., 2020, A novel strategy for multi-part production in additive manufacturing. Int J Adv Manuf Technol, 109(5):1237–1248. https://doi.org/10.1007/s00170-020-05734-8
Ko H, Witherell P, Lu Y, et al., 2021, Machine learning and knowledge graph based design rule construction for additive manufacturing. Addit Manuf, 37:101620. https://doi.org/10.1016/j.addma.2020.101620
Li Z, Zhang Z, Shi J, et al., 2019, Prediction of surface roughness in extrusion-based additive manufacturing with machine learning. Robot Cim-Int Manuf, 57: 488–495. https://doi.org/10.1016/j.rcim.2019.01.004
Zhu Z, Anwer N, Huang Q, et al., 2018, Machine learning in tolerancing for additive manufacturing. CIRP Ann, 67(1):157–160. https://doi.org/10.1016/j.cirp.2018.04.119
Jiang J, Xiong Y, Zhang Z, et al., 2022, Machine learning integrated design for additive manufacturing. J Intell Manuf, 33(4):1073–1086. https://doi.org/10.1007/s10845-020-01715-6
Ghayoomi Mohammadi M, Mahmoud D, Elbestawi M, 2021, On the application of machine learning for defect detection in L-PBF additive manufacturing. Opt Laser Technol, 143:107338. https://doi.org/10.1016/j.optlastec.2021.107338
Caggiano A, Zhang J, Alfieri V, et al., 2019, Machine learning-based image processing for on-line defect recognition in additive manufacturing. CIRP Ann, 68(1):451–454. https://doi.org/10.1016/j.cirp.2019.03.021
Gobert C, Reutzel EW, Petrich J, et al., 2018, Application of supervised machine learning for defect detection during metallic powder bed fusion additive manufacturing using high resolution imaging. Addit Manuf, 21:517–528. https://doi.org/10.1016/j.addma.2018.04.005
Li R, Jin M, Paquit VC, 2021, Geometrical defect detection for additive manufacturing with machine learning models. Mater Design, 206:109726. https://doi.org/10.1016/j.matdes.2021.109726
Bezdek JC, Chuah SK, Leep D, 1986, Generalized k-nearest neighbor rules. Fuzzy Set Syst, 18(3):237–256. https://doi.org/10.1016/0165-0114(86)90004-7
Gou J, Ma H, Ou W, et al., 2019, A generalized mean distance-based k-nearest neighbor classifier. Expert Syst Appl, 115:356–372. https://doi.org/10.1016/j.eswa.2018.08.021
Wang C, Shi Y, Fan X, et al., 2019, Attribute reduction based on k-nearest neighborhood rough sets. Int J Approx Reason, 106:18–31. https://doi.org/10.1016/j.ijar.2018.12.013
Gallego A-J, Calvo-Zaragoza J, Valero-Mas JJ, et al., 2018, Clustering-based k-nearest neighbor classification for large-scale data with neural codes representation. Pattern Recogn, 74:531–543. https://doi.org/10.1016/j.patcog.2017.09.038
Zhang Q, Zhou H, Jiang Y, et al., 2019, A simple joint modulation format identification and OSNR monitoring scheme for IMDD OOFDM transceivers using K-nearest neighbor algorithm. Appl Sci, 9(18):3892.
Ertuğrul ÖF, Tağluk ME, 2017, A novel version of k nearest neighbor: Dependent nearest neighbor. Appl Soft Comput, 55:480–490. https://doi.org/10.1016/j.asoc.2017.02.020
Shahabi H, Shirzadi A, Ghaderi K, et al., 2020, Flood detection and susceptibility mapping using sentinel-1 remote sensing data and a machine learning approach: Hybrid intelligence of bagging ensemble based on k-nearest neighbor classifier. Remote Sens, 12(2):266.
Zhang Z, Jiang T, Li S, et al., 2018, Automated feature learning for nonlinear process monitoring—An approach using stacked denoising autoencoder and k-nearest neighbor rule. J Process Contr, 64:49–61. https://doi.org/10.1016/j.jprocont.2018.02.004
Basheer IA, Hajmeer M, 2000, Artificial neural networks: Fundamentals, computing, design, and application. J Microbiol Methods, 43(1):3–31. https://doi.org/10.1016/S0167-7012(00)00201-3
Wu Y-c, Feng J-w, 2018, Development and application of artificial neural network. Wireless Pers Commun, 102(2):1645–1656. https://doi.org/10.1007/s11277-017-5224-x
Huang Y, 2009, Advances in artificial neural networks– methodological development and application[J]. Algo, 2(3):973–1007. https://doi.org/10.3390/algor2030973
Yang GR, Wang X-J, 2020, Artificial neural networks for neuroscientists: A primer. Neuron, 107(6):1048–1070. https://doi.org/10.1016/j.neuron.2020.09.005
Abiodun OI, Jantan A, Omolara AE, et al., 2018, State-of-the-art in artificial neural network applications: A survey. Heliyon, 4(11):e00938. https://doi.org/10.1016/j.heliyon.2018.e00938
Vlachas PR, Pathak J, Hunt BR, et al., 2020, Backpropagation algorithms and reservoir computing in recurrent neural networks for the forecasting of complex spatiotemporal dynamics. Neural Netw, 126:191–217. https://doi.org/10.1016/j.neunet.2020.02.016
Chan LW, Fallside F, 1987, An adaptive training algorithm for back propagation networks. Comput Speech Lang, 2(3):205–218. https://doi.org/10.1016/0885-2308(87)90009-X
Hameed AA, Karlik B, Salman MS, 2016, Back-propagation algorithm with variable adaptive momentum. Knowledge- Based Syst, 114:79–87. https://doi.org/10.1016/j.knosys.2016.10.001
Wright LG, Onodera T, Stein MM, et al., 2022, Deep physical neural networks trained with backpropagation. Nature, 601(7894):549–555. https://doi.org/10.1038/s41586-021-04223-6
Lawrence S, Giles CL, Ah Chung T, et al., 1997, Face recognition: a convolutional neural-network approach. IEEE Trans Neural Netw, 8(1):98–113. https://doi.org/10.1109/72.554195
Jaderberg M, Simonyan K, Vedaldi A, et al., 2016, Reading text in the wild with convolutional neural networks. Int J Comput Vis, 116(1):1–20. https://doi.org/10.1007/s11263-015-0823-z
Acharya UR, Oh SL, Hagiwara Y, et al., 2017, A deep convolutional neural network model to classify heartbeats. Comput Biol Med, 89:389–396. https://doi.org/10.1016/j.compbiomed.2017.08.022
Zhou D-X, 2020, Theory of deep convolutional neural networks: Downsampling. Neural Netw, 124:319–327. https://doi.org/10.1016/j.neunet.2020.01.018
Yamashita R, Nishio M, Do RKG, et al., 2018, Convolutional neural networks: An overview and application in radiology. Insights Imaging, 9(4):611–629. https://doi.org/10.1007/s13244-018-0639-9
Brodrick PG, Davies AB, Asner GP, 2019, Uncovering ecological patterns with convolutional neural networks. Trends Ecol Evol, 34(8):734–745. https://doi.org/10.1016/j.tree.2019.03.006
Alhussein M, Aurangzeb K, Haider SI, 2020, Hybrid CNN-LSTM model for short-term individual household load forecasting. IEEE Access, 8:180544–180557. https://doi.org/10.1109/ACCESS.2020.3028281
Tian C, Ma J, Zhang C, et al, 2018, A deep neural network model for short-term load forecast based on long short-term memory network and convolutional neural network[J]. Energies, 11(12):3493. https://doi.org/10.3390/en11123493
Kim HY, Won CH, 2018, Forecasting the volatility of stock price index: A hybrid model integrating LSTM with multiple GARCH-type models. Expert Syst Appl, 103:25–37. https://doi.org/10.1016/j.eswa.2018.03.002
Park K, Choi Y, Choi WJ, et al., 2020, LSTM-based battery remaining useful life prediction with multi-channel charging profiles. IEEE Access, 8:20786–20798. https://doi.org/10.1109/ACCESS.2020.2968939
Yu Y, Si X, Hu C, et al., 2019, A review of recurrent neural networks: LSTM cells and network architectures. Neural Comput, 31(7):1235–1270. https://doi.org/10.1162/neco_a_01199
Chimmula VKR, Zhang L, 2020, Time series forecasting of COVID-19 transmission in Canada using LSTM networks. Chaos Solitons Fract, 135:109864. https://doi.org/10.1016/j.chaos.2020.109864
Shahid F, Zameer A, Muneeb M, 2020, Predictions for COVID-19 with deep learning models of LSTM, GRU and Bi-LSTM. Chaos Solitons Fract, 140:110212. https://doi.org/10.1016/j.chaos.2020.110212
Zhao J, Mao X, Chen L, 2019, Speech emotion recognition using deep 1D & 2D CNN LSTM networks. Biomed Signal Process, 47:312–323. https://doi.org/10.1016/j.bspc.2018.08.035
Kim T-Y, Cho S-B, 2019, Predicting residential energy consumption using CNN-LSTM neural networks. Energy, 182:72–81. https://doi.org/10.1016/j.energy.2019.05.230
Graves A, Schmidhuber J, 2005, Framewise phoneme classification with bidirectional LSTM and other neural network architectures. Neural Netw, 18(5):602–610. https://doi.org/10.1016/j.neunet.2005.06.042
Wang G, Hao J, Ma J, et al., 2011, A comparative assessment of ensemble learning for credit scoring. Expert Syst Appl, 38(1):223–230. https://doi.org/10.1016/j.eswa.2010.06.048
Xu R, Lin H, Lu K, et al, 2021, A forest fire detection system based on ensemble learning[J]. Forests, 12(2):217. https://doi.org/10.3390/f12020217
Krawczyk B, Minku LL, Gama J, et al., 2017, Ensemble learning for data stream analysis: A survey. Inform Fusion, 37:132–156. https://doi.org/10.1016/j.inffus.2017.02.004
Dong X, Yu Z, Cao W, et al., 2020, A survey on ensemble learning. Front Comput, 14(2):241–258. https://doi.org/10.1007/s11704-019-8208-z
Mienye ID, Sun Y, 2022, A survey of ensemble learning: Concepts, algorithms, applications, and prospects. IEEE Access, 10:99129–99149. https://doi.org/10.1109/ACCESS.2022.3207287
Mao S, Chen J-W, Jiao L, et al., 2019, Maximizing diversity by transformed ensemble learning. Appl Soft Comput, 82:105580. https://doi.org/10.1016/j.asoc.2019.105580
Wang G, Sun J, Ma J, et al., 2014, Sentiment classification: The contribution of ensemble learning. Decis Support Syst, 57:77–93. https://doi.org/10.1016/j.dss.2013.08.002
Hamori S, Kawai M, Kume T, et al., 2018, Ensemble learning or deep learning? Application to default risk analysis. J Risk Financ Manage, 11(1):12.
Xu R, Lin H, Lu K, et al., 2021, A forest fire detection system based on ensemble learning. Forests, 12(2):217.
Yang Y, Lv H, Chen N, 2022, A survey on ensemble learning under the era of deep learning. Artif Intell Rev: 1–45. https://doi.org/10.1007/s10462-022-10283-5
Ghorbanzadeh O, Blaschke T, Gholamnia K, et al, 2019, Evaluation of different machine learning methods and deep-learning convolutional neural networks for landslide detection[J]. Remote Sens, 11(2):196. https://doi.org/10.3390/rs11020196
Khan A, Sohail A, Zahoora U, et al., 2020, A survey of the recent architectures of deep convolutional neural networks. Artif Intell Rev, 53(8):5455–5516. https://doi.org/10.1007/s10462-020-09825-6
Reina-Romo E, Mandal S, Amorim P, et al., 2021, Towards the experimentally-informed in silico nozzle design optimization for extrusion-based bioprinting of shear-thinning hydrogels. Front Bioeng Biotechnol, 9:701778.
Allencherry J, Pradeep N, Shrivastava R, et al., 2022, Investigation of hydrogel and gelatin bath formulations for extrusion-based 3D bioprinting using deep learning. Procedia CIRP, 110:360–365. https://doi.org/10.1016/j.procir.2022.06.064
Xu H, Liu Q, Casillas J, et al., 2022, Prediction of cell viability in dynamic optical projection stereolithography-based bioprinting using machine learning. J Intell Manuf, 33(4):995–1005. https://doi.org/10.1007/s10845-020-01708-5
Wu D, Xu C, 2018, Predictive modeling of droplet formation processes in inkjet-based bioprinting. J Manuf Sci E-T Asme, 140(10):101007. https://doi.org/10.1115/1.4040619
Lee J, Oh SJ, An SH, et al., 2020, Machine learning-based design strategy for 3D printable bioink: Elastic modulus and yield stress determine printability. Biofabrication, 12(3):035018. https://doi.org/10.1088/1758-5090/ab8707
Yamanishi C, Parigoris E, Takayama S, 2020, Kinetic analysis of label-free microscale collagen gel contraction using machine learning-aided image analysis. Front Bioeng Biotechnol, 8:582602.
Tourlomousis F, Jia C, Karydis T, et al., 2019, Machine learning metrology of cell confinement in melt electrowritten three-dimensional biomaterial substrates. Microsyst Nanoeng, 5(1):1–19.
Safir F, Vu N, Tadesse LF, et al., 2022, Detecting bacteria in multi-cellular samples with combined acoustic bioprinting and Raman spectroscopy. arXiv preprint arXiv:2206.09304.
Nadernezhad A, Groll J, 2022, Machine learning reveals a general understanding of printability in formulations based on rheology additives. Adv Sci, 9(29):2202638. https://doi.org/10.1002/advs.202202638
Guan J, You S, Xiang Y, et al., 2021, Compensating the cell-induced light scattering effect in light-based bioprinting using deep learning. Biofabrication, 14(1):015011. https://doi.org/10.1088/1758-5090/ac3b92
Bone JM, Childs CM, Menon A, et al., 2020, Hierarchical machine learning for high-fidelity 3D printed biopolymers. ACS Biomater Sci Eng, 6(12):7021–7031. https://doi.org/10.1021/acsbiomaterials.0c00755
Shi J, Song J, Song B, et al., 2019, Multi-objective optimization design through machine learning for drop-on-demand bioprinting. Engineering, 5(3):586–593. https://doi.org/10.1016/j.eng.2018.12.009
Shi J, Wu B, Song B, et al., 2018, Learning-based cell injection control for precise drop-on-demand cell printing. Ann Biomed Eng, 46(9):1267–1279. https://doi.org/10.1007/s10439-018-2054-2
Fu Z, Angeline V, Sun W, 2021, Evaluation of printing parameters on 3D extrusion printing of pluronic hydrogels and machine learning guided parameter recommendation. Int J Bioprint, 7(4):434.
Tian S, Stevens R, McInnes BT, et al., 2021, Machine assisted experimentation of extrusion-based bioprinting systems. Micromachines, 12(7):780.
Ruberu K, Senadeera M, Rana S, et al., 2021, Coupling machine learning with 3D bioprinting to fast track optimisation of extrusion printing. Appl Mater Today, 22:100914. https://doi.org/10.1016/j.apmt.2020.100914
Jin Z, Zhang Z, Shao X, et al., 2021, Monitoring anomalies in 3D bioprinting with deep neural networks. ACS Biomater Sci Eng. https://doi.org/10.1021/acsbiomaterials.0c01761
Bonatti AF, Vozzi G, Kai Chua C, et al., 2022, A deep learning approach for error detection and quantification in extrusion-based bioprinting. Mater Today, 70:131–135. https://doi.org/10.1016/j.matpr.2022.09.006
Tebon PJ, Wang B, Markowitz AL, et al., 2022, Drug screening at single-organoid resolution via bioprinting and interferometry. bioRxiv, 2021:2021–2031.
Tröndle K, Miotto G, Rizzo L, et al., 2022, Deep learning-assisted nephrotoxicity testing with bioprinted renal spheroids. Int J Bioprint, 8(2):528.