Advancing embryo selection in artificial intelligence-assisted reproductive technologies: A systematic review
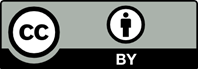
For couples encountering infertility challenges, assisted reproductive technologies (ARTs) offer a path to parenthood. ART procedures, such as in vitro fertilization (IVF), intracytoplasmic sperm injection (ICSI), and embryo implantation, involve the handling of sperm or embryos outside the body. However, the success of ART depends on the accurate selection of viable embryos. Artificial intelligence (AI) is a promising tool with the potential to revolutionize these procedures. This review explores the transformative potential of AI in ART, providing valuable insights into enhanced embryo selection and unlocking new possibilities for the field. Four electronic databases were systematically searched under the Preferred Reporting Items for Systematic Reviews and Meta-Analyses guidelines. From an initial pool of 914 papers, 30 studies were selected for further evaluation. While noting the limitations inherent in the existing body of research, this review offers a broad analysis of AI’s transformative role in embryo selection. It highlights the significant potential of AI to enhance precision, consistency, and efficiency in ART. This review also emphasizes the importance of addressing technical, ethical, and regulatory aspects to ensure responsible and effective integration of these technologies. The findings indicate that AI-based models, such as the iDAScore v2.0, have demonstrated promising results in accurately predicting embryo viability and evaluating the effects of maternal age on embryo viability. Specifically, Bayesian network modeling, with an accuracy rate of 91.3%, aims to optimize IVF and ICSI procedures. In summary, AI stands at the forefront of innovation in ART, offering new hope through more accurate and efficient embryo selection.
- Cox CM, Thoma ME, Tchangalova N, et al. Infertility prevalence and the methods of estimation from 1990 to 2021: A systematic review and meta-analysis. Hum Reprod Open. 2022;2022(4):hoac051. doi: 10.1093/hropen/hoac051
- Borumandnia N, Alavi Majd H, Khadembashi N, Alaii H. Worldwide trend analysis of primary and secondary infertility rates over past decades: A cross-sectional study. Int J Reprod Biomed. 2022;20:37-46. doi: 10.18502/ijrm.v20i1.10407
- Abdullah KAL, Atazhanova T, Chavez-Badiola A, Shivhare SB. Automation in ART: Paving the way for the future of infertility treatment. Reprod Sci. 2023;30(4):1006-1016. doi: 10.1007/s43032-022-00941-y
- Raef B, Ferdousi R. A review of machine learning approaches in assisted reproductive technologies. Acta Inform Med. 2019;27(3):205-211. doi: 10.5455/aim.2019.27.205-211
- Wang R, Pan W, Jin L, et al. Artificial intelligence in reproductive medicine. Reproduction. 2019;158(4):R139-R154. doi: 10.1530/REP-18-0523
- Sunde A, Balaban B. The assisted reproductive technology laboratory: Toward evidence-based practice? Fertil Steril. 2013;100(2):310-318. doi: 10.1016/j.fertnstert.2013.06.032
- Chu KY, Nassau DE, Arora H, Lokeshwar SD, Madhusoodanan V, Ramasamy R. Artificial intelligence in reproductive urology. Curr Urol Rep. 2019;20(9):52. doi: 10.1007/s11934-019-0914-4
- Omur AD. Artificial intelligence in gamete cell selection and microbiologic analysis. J Clin Vet Res. 2022;2(2):1-7. doi: 10.54289/JCVR2200107
- Afnan MAM, Rudin C, Conitzer V, et al. Ethical Implementation of Artificial Intelligence to Select Embryos in in Vitro Fertilization. In: Proceedings of the 2021 AAAI/ ACM Conference on AI, Ethics, and Society. ACM; 2021. p. 316-326. doi: 10.1145/3461702.3462589
- Meseguer M, Kruhne U, Laursen S. Full in vitro fertilization laboratory mechanization: Toward robotic assisted reproduction? Fertil Steril. 2012;97(6):1277-1286. doi: 10.1016/j.fertnstert.2012.03.013
- Polanski LT, Coelho Neto MA, Nastri CO, et al. Time‐lapse embryo imaging for improving reproductive outcomes: Systematic review and meta‐analysis. Ultrasound Obstet Gynecol. 2014;44(4):394-401. doi: 10.1002/uog.13428
- Bormann CL, Curchoe CL, Thirumalaraju P, et al. Deep learning early warning system for embryo culture conditions and embryologist performance in the ART laboratory. J Assist Reprod Genet. 2021;38(7):1641-1646. doi: 10.1007/s10815-021-02198-x
- Fernandez EI, Ferreira AS, Cecílio MHM, et al. Artificial intelligence in the IVF laboratory: Overview through the application of different types of algorithms for the classification of reproductive data. J Assist Reprod Genet. 2020;37(10):2359-2376. doi: 10.1007/s10815-020-01881-9
- Zaninovic N, Rosenwaks Z. Artificial intelligence in human in vitro fertilization and embryology. Fertil Steril. 2020;114(5):914-920. doi: 10.1016/j.fertnstert.2020.09.157
- McKenzie JE, Beller EM, Forbes AB. Introduction to systematic reviews and meta‐analysis. Respirology. 2016;21(4):626-637. doi: 10.1111/resp.12783
- Theilgaard Lassen J, Fly Kragh M, Rimestad J, Nygård Johansen M, Berntsen J. Development and validation of deep learning based embryo selection across multiple days of transfer. Sci Rep. 2023;13(1):4235. doi: 10.1038/s41598-023-31136-3
- Cimadomo D, Chiappetta V, Innocenti F, et al. Towards automation in IVF: Pre-clinical validation of a deep learning-based embryo grading system during PGT-A cycles. J Clin Med. 2023;12(5):1806. doi: 10.3390/jcm12051806
- Johansen MN, Parner ET, Kragh MF, et al. Comparing performance between clinics of an embryo evaluation algorithm based on time-lapse images and machine learning. J Assist Reprod Genet. 2023;40(9):2129-2137. doi: 10.1007/s10815-023-02871-3
- Bori L, Meseguer F, Valera MA, Galan A, Remohi J, Meseguer M. The higher the score, the better the clinical outcome: Retrospective evaluation of automatic embryo grading as a support tool for embryo selection in IVF laboratories. Hum Reprod. 2022;37(6):1148-1160. doi: 10.1093/humrep/deac066
- Glatstein I, Chavez-Badiola A, Curchoe CL. New frontiers in embryo selection. J Assist Reprod Genet. 2023;40(2):223-234. doi: 10.1007/s10815-022-02708-5
- Salih M, Austin C, Warty RR, et al. Embryo selection through artificial intelligence versus embryologists: A systematic review. Hum Reprod Open. 2023;2023(3):hoad031. doi: 10.1093/hropen/hoad031
- Tian T, Kong F, Yang R, et al. A Bayesian network model for prediction of low or failed fertilization in assisted reproductive technology based on a large clinical real-world data. Reprod Biol Endocrinol. 2023;21(1):8. doi: 10.1186/s12958-023-01065-x
- Ueno S, Berntsen J, Okimura T, Kato K. Improved pregnancy prediction performance in an updated deep-learning embryo selection model: A retrospective independent validation study. Reprod Biomed Online. 2024;48(1):103308. doi: 10.1016/j.rbmo.2023.103308
- Berntsen J, Rimestad J, Lassen JT, Tran D, Kragh MF. Robust and generalizable embryo selection based on artificial intelligence and time-lapse image sequences. PLoS One. 2022;17(2):e0262661. doi: 10.1371/journal.pone.0262661
- Chen L, Li W, Liu Y, et al. Non-invasive embryo selection strategy for clinical IVF to avoid wastage of potentially competent embryos. Reprod Biomed Online. 2022;45(1):26-34. doi: 10.1016/j.rbmo.2022.03.006
- Xi Q, Yang Q, Wang M, et al. Individualized embryo selection strategy developed by stacking machine learning model for better in vitro fertilization outcomes: An application study. Reprod Biol Endocrinol. 2021;19(1):53. doi: 10.1186/s12958-021-00734-z
- Ratna MB, Bhattacharya S, McLernon DJ. External validation of models for predicting cumulative live birth over multiple complete cycles of IVF treatment. Hum Reprod. 2023;38(10):1998-2010. doi: 10.1093/humrep/dead165
- Diakiw SM, Hall JMM, VerMilyea MD, et al. Development of an artificial intelligence model for predicting the likelihood of human embryo euploidy based on blastocyst images from multiple imaging systems during IVF. Hum Reprod. 2022;37(8):1746-1759. doi: 10.1093/humrep/deac131
- Sawada Y, Sato T, Nagaya M, et al. Evaluation of artificial intelligence using time-lapse images of IVF embryos to predict live birth. Reprod Biomed Online. 2021;43(5):843-852. doi: 10.1016/j.rbmo.2021.05.002
- Cheredath A, Uppangala S, Asha CS, et al. Combining machine learning with metabolomic and embryologic data improves embryo implantation prediction. Reprod Sci. 2023;30(3):984-994. doi: 10.1007/s43032-022-01071-1
- Patil S, Madiwalar S, Aparanji V. Machine learning techniques to improve the success rate in in-vitro fertilization (IVF) procedure. IOP Conf Ser Mater Sci Eng. 2020;925:012039. doi: 10.1088/1757-899X/925/1/012039
- Giscard d’Estaing S, Labrune E, Forcellini M, et al. A machine learning system with reinforcement capacity for predicting the fate of an ART embryo. Syst Biol Reprod Med. 2021;67(1):64-78. doi: 10.1080/19396368.2020.1822953
- Kanakasabapathy MK, Thirumalaraju P, Bormann CL, et al. Development and evaluation of inexpensive automated deep learning-based imaging systems for embryology. Lab Chip. 2019;19(24):4139-4145. doi: 10.1039/C9LC00721K
- Pons MC, Carrasco B, Rives N, et al. Predicting the likelihood of live birth: An objective and user-friendly blastocyst grading system. Reprod Biomed Online. 2023;47(3):103243. doi: 10.1016/j.rbmo.2023.05.015
- Bori L, Gimenez C, Clark G, Babariya D, Wells D, Meseguer M. O-323 Sex-dependent discrepancies in automatic scoring: Implications for the performance of embryo evaluation and selection methods. Hum Reprod. 2024;39(Suppl 1):deae108.380. doi: 10.1093/humrep/deae108.380
- Valera MÁ, Conversa L, Murria L, Bori L, Garg A, Meseguer M. P-174 Do culture conditions alter the efficacy of embryo selection algorithms using time-lapse technology? Development of novel embryo selection model with embryos cultured in different conditions. Hum Reprod. 2023;38(Suppl 1):dead093.534. doi: 10.1093/humrep/dead093.534
- Sfontouris I, Nikiforaki D, Liarmakopoulou S, et al. P-280 Potential for improvement and current limitations of Artificial Intelligence (AI) for embryo selection: Analysis of external validation data. Hum Reprod. 2022;37(Suppl 1):deac107.269. doi: 10.1093/humrep/deac107.269
- Fauser BCJM, Braat DDM. Assisted reproductive technology. In: Textbook of Obstetrics and Gynaecology. Netherlands: Bohn Stafleu van Loghum; 2019. p. 263-282. doi: 10.1007/978-90-368-2131-5_14
- Bhandari HM, Choudhary MK, Stewart JA. An overview of assisted reproductive technology procedures. Obstet Gynaecol. 2018;20(3):167-176. doi: 10.1111/tog.12509
- Gwatkin RBL. Fertilization in vitro. In: Fertilization Mechanisms in Man and Mammals. Berlin: Springer US; 1977. p. 33-51. doi: 10.1007/978-1-4615-8804-7_5
- Jurisica I, Mylopoulos J, Glasgow J, Shapiro H, Casper RF. Case-based reasoning in IVF: Prediction and knowledge mining. Artif Intell Med. 1998;12(1):1-24. doi: 10.1016/S0933-3657(97)00037-7
- Bing Y, Ouellette RJ. Fertilization in vitro. Methods Mol Biol. 2009;550:251-266. doi: 10.1007/978-1-60327-009-0_16
- In Vitro Fertilization (IVF). In: The SAGE Encyclopedia of Children and Childhood Studies. London: SAGE Publications, Inc.; 2020. doi: 10.4135/9781529714388.n344
- Reljič M, Knez J, Kovač V, Kovačič B. Endometrial injury, the quality of embryos, and blastocyst transfer are the most important prognostic factors for in vitro fertilization success after previous repeated unsuccessful attempts. J Assist Reprod Genet. 2017;34(6):775-779. doi: 10.1007/s10815-017-0916-4
- Aflatoonian A, Asgharnia M. Factors affecting the successful embryo transfer. IJRM. 2006;4(2):45-50.
- Zheng D, Zeng L, Yang R, et al. Intracytoplasmic sperm injection (ICSI) versus conventional in vitro fertilisation (IVF) in couples with non-severe male infertility (NSMI-ICSI): Protocol for a multicentre randomised controlled trial. BMJ Open. 2019;9(9):e030366. doi: 10.1136/bmjopen-2019-030366
- Hardy K, Wright C, Rice S, et al. Future developments in assisted reproduction in humans. Reproduction. 2002;123(2):171-183. doi: 10.1530/rep.0.1230171
- Palermo GD, Sills ES, editors. Intracytoplasmic Sperm Injection. Berlin: Springer International Publishing; 2018. doi: 10.1007/978-3-319-70497-5
- Simopoulou M, Sfakianoudis K, Tsioulou P, et al. Risks in surrogacy considering the embryo: From the preimplantation to the gestational and neonatal period. Biomed Res Int. 2018;2018:1-9. doi: 10.1155/2018/6287507
- Igreja AR, Ricou M. Surrogacy: Challenges and ambiguities. New Bioethics. 2019;25(1):60-77. doi: 10.1080/20502877.2019.1564007
- van den Akker OBA. Introduction to surrogacy: Historical and present day context. In: Surrogate Motherhood Families. Berlin: Springer International Publishing; 2017. p. 5-37. doi: 10.1007/978-3-319-60453-4_1
- Patel N, Jadeja Y, Bhadarka H, Patel M, Patel N, Sodagar N. Insight into different aspects of surrogacy practices. J Hum Reprod Sci. 2018;11(3):212. doi: 10.4103/jhrs.JHRS_138_17
- Nakash A, Herdiman J. Surrogacy. J Obstet Gynaecol (Lahore). 2007;27(3):246-251. doi: 10.1080/01443610701194788
- Seidinova MA. Ethical issues of surrogacy. Bull Karagandy Univ Law Ser. 2023;110:139-146. doi: 10.31489/2023L2/139-146
- Bashiri A, Cherlow Y, Kresch-Jaffe T. Surrogacy: An important pathway to parenthood. A call for international standardization. J Reprod Immunol. 2024;163:104247. doi: 10.1016/j.jri.2024.104247
- Mulligan A. Surrogacy and the significance of gestation: Implications for law and policy. Bioethics. 2024;38(8):674-683. doi: 10.1111/bioe.13302
- Patil SN, Wali UV, Swamy MK. Selection of Single Potential Embryo to Improve the Success Rate of Implantation in IVF Procedure using Machine Learning Techniques. In: 2019 International Conference on Communication and Signal Processing (ICCSP). IEEE; 2019. p. 881-886. doi: 10.1109/ICCSP.2019.8697982
- Duffus JH, Schwenk M, Templeton DM. Gamete Intrafallopian Transfer (GIFT). United States: IUPAC Standards Online. doi: 10.1515/iupac.88.0833
- Tucker MJ, Leung CK, Leong MK, et al. Routine gamete intrafallopian transfer (GIFT): A highly successful option for treatment of non‐tubal infertility. Asia Oceania J Obstet Gynaecol. 1989;15(3):245-251. doi: 10.1111/j.1447-0756.1989.tb00184.x
- Tanaka A, Toeda M, Nagasawa I, Yamamoto T, Nakagata N. Development of gamete intrafallopian transfer with preincubated preovulatory oocytes (new GIFT) and analysis of clinical data. Nihon Sanka Fujinka Gakkai Zasshi. 1988;40(12):1859-1866.
- Meirow D, Schenker JG. Appraisal of gamete intrafallopian transfer. Eur J Obstet Gynecol Reprod Biol. 1995;58(1):59-65. doi: 10.1016/0028-2243(94)01940-9
- Pool TB, Ellsworth LR, Garza JR, Martin JE, Miller SS, Atiee SH. Zygote intrafallopian transfer as a treatment for nontubal infertility: A 2-year study. Fertil Steril. 1990;54(3):482-488. doi: 10.1016/S0015-0282(16)53766-2
- Tournay H, Camus M, Khan I, Staessen C, Van Steirteghem AC, Devroey P. In-vitro fertilization, gamete- or zygote intra-Fallopian transfer for the treatment of male infertility. Hum Reprod. 1991;6(2):263-266. doi: 10.1093/oxfordjournals.humrep.a137319
- Farhi J, Weissman A, Nahum H, Levran D. Zygote intrafallopian transfer in patients with tubal factor infertility after repeated failure of implantation with in vitro fertilization-embryo transfer. Fertil Steril. 2000;74(2):390-393. doi: 10.1016/S0015-0282(00)00610-5
- Levran D, Mashiach S, Dor J, Levron J, Farhi J. Zygote intrafallopian transfer may improve pregnancy rate in patients with repeated failure of implantation. Fertil Steril. 1998;69(1):26-30. doi: 10.1016/S0015-0282(97)00452-4
- Tournaye H, Devroey P, Camus M, Valkenburg M, Bollen N, Van Steirteghem AC. Zygote intrafallopian transfer or in vitro fertilization and embryo transfer for the treatment of male-factor infertility: A prospective randomized trial. Fertil Steril. 1992;58(2):344-350. doi: 10.1016/S0015-0282(16)55195-4
- Fluker MR, Zouves CG, Bebbington MW. A prospective randomized comparison of zygote intrafallopian transfer and in vitro fertilization-embryo transfer for nontubal factor infertility. Fertil Steril. 1993;60(3):515-519. doi: 10.1016/S0015-0282(16)56170-6
- Manna C, Nanni L, Lumini A, Pappalardo S. Artificial intelligence techniques for embryo and oocyte classification. Reprod Biomed Online. 2013;26(1):42-49. doi: 10.1016/j.rbmo.2012.09.015
- Góngora A, Miranda JA, Gordillo E, Dabbah J. Statistical analysis on artificial insemination human fertility Centre, Mexico City. Int Congr Ser. 2004;1271:3-6. doi: 10.1016/j.ics.2004.06.018
- Dalal RJ, Mishra AP, Gupta S. Artificial intelligence in assisted reproductive technology: Present and future. Int J Infertil Fetal Med. 2020;11(3):61-64. doi: 10.5005/jp-journals-10016-1208
- Jain K. Artificial intelligence in assisted reproductive technology-current scenario and future implications. Fertil Sci Res. 2019;6(2):57. doi: 10.4103/fsr.fsr_40_19
- Montag M, Toth B, Strowitzki T. New approaches to embryo selection. Reprod Biomed Online. 2013;27(5):539-546. doi: 10.1016/j.rbmo.2013.05.013
- Bulletti FM, Berrettini M, Sciorio R, Bulletti C. Artificial intelligence algorithms for optimizing assisted reproductive technology programs: A systematic review. Global Transl Med. 2023;2(2):0308. doi: 10.36922/gtm.0308
- Bhagwan KV, Kadam S. A comprehensive study on artificial intelligence and machine learning. Int J Adv Res Sci Commun Technol. 2024;4:276-279. doi: 10.48175/IJARSCT-19939
- Lin J, Sun XX. Predictive modeling in reproductive medicine. Reprod Dev Med. 2018;2(4):224-229. doi: 10.4103/2096-2924.249888
- Rosenwaks Z. Artificial intelligence in reproductive medicine: A fleeting concept or the wave of the future? Fertil Steril. 2020;114(5):905-907. doi: 10.1016/j.fertnstert.2020.10.002
- Raimundo J, Cabrita P. Artificial intelligence at assisted reproductive technology. Procedia Comput Sci. 2021;181:442-447. doi: 10.1016/j.procs.2021.01.189
- Dimitriadis I, Zaninovic N, Badiola AC, Bormann CL. Artificial intelligence in the embryology laboratory: A review. Reprod Biomed Online. 2022;44(3):435-448. doi: 10.1016/j.rbmo.2021.11.003
- Afnan MAM, Liu Y, Conitzer V, et al. Interpretable, not black-box, artificial intelligence should be used for embryo selection. Hum Reprod Open. 2021;2021(4):hoab040. doi: 10.1093/hropen/hoab040
- Benchaib M, Labrune E, Giscard d’Estaing S, Salle B, Lornage J. Shallow artificial networks with morphokinetic time‐lapse parameters coupled to ART data allow to predict live birth. Reprod Med Biol. 2022;21(1):e12486. doi: 10.1002/rmb2.12486
- Yu L, Lam KKW, Ng EHY, et al. Deep Learning-Based Embryo Assessment of Static Images can Reduce the Time to Live Birth in In Vitro Fertilization. medRxiv [Preprint]; 2024. doi: 10.1101/2024.10.28.24316259
- Liao Z, Yan C, Wang J, et al. A clinical consensus-compliant deep learning approach to quantitatively evaluate human in vitro fertilization early embryonic development with optical microscope images. Artif Intell Med. 2024;149:102773. doi: 10.1016/j.artmed.2024.102773
- Popa T, He C, Vasconcelos F, et al. P-168 Both artificial intelligence and manual embryo selection methods show sex-bias, favouring male embryos-insights from the largest embryo sex study using time-lapse and PGT-A. Hum Reprod. 2024;39(Suppl 1):deae108.539. doi: 10.1093/humrep/deae108.539
- Illingworth PJ, Venetis C, Gardner DK, et al. Deep learning versus manual morphology-based embryo selection in IVF: A randomized, double-blind noninferiority trial. Nat Med. 2024;30(11):3114-3120. doi: 10.1038/s41591-024-03166-5
- Huang JYJ, Rosenwaks Z. Assisted reproductive techniques. Methods Mol Biol. 2014;1154:171-231. doi: 10.1007/978-1-4939-0659-8_8
- Prostate pathophysiology. In: Atlas of Clinical Andrology. United States: CRC Press; 2005. p. 146-157. doi: 10.1201/b14619-17
- Kragh MF, Karstoft H. Embryo selection with artificial intelligence: How to evaluate and compare methods? J Assist Reprod Genet. 2021;38(7):1675-1689. doi: 10.1007/s10815-021-02254-6
- Merican ZZ, Yusof UK, Abdullah NL. Review on Embryo Selection Based on Morphology Using Machine Learning Methods; 2021. Available from: https://api.semanticscholar. org/corpusid:236880800 [Last accessed on 2025 Apr 28].
- Medenica S, Zivanovic D, Batkoska L, et al. The future is coming: Artificial intelligence in the treatment of infertility could improve assisted reproduction outcomes-the value of regulatory frameworks. Diagnostics. 2022;12(12):2979. doi: 10.3390/diagnostics12122979
- Curchoe CL. Meetings that matter: Time to put artificial intelligence on the ART roadmap. J Assist Reprod Genet. 2022;39(7):1493-1496. doi: 10.1007/s10815-022-02520-1
- Tran HP, Tran LNH, Dang HT, et al. A SWOT analysis of human- and machine learning- based embryo assessment. IEEE Access. 2020;8:227466-227481. doi: 10.1109/ACCESS.2020.3045772
- Kuo C, Zuo J, Han W, et al. Intelligent Assisted Reproduction: Innovative Applications of artificial Intelligence in Embryo Health Assessment. Authorea [Preprints]; 2025. doi: 10.22541/au.173639046.67662628/v1
- Presacan O, Dorobanțiu A, Thambawita V, et al. Embryo 2.0: Merging Synthetic and Real Data for Advanced AI Predictions [Preprint]; 2024. doi: 10.48550/arxiv.2412.01255
- Kaveh S, Ghafari A, Khedri Z, et al. Investigating the Artificial Intelligence in Prediction and Evaluation of Sperm and Embryo Quality in In Vitro Fertilization (IVF): A Systematic Review. [Preprint (Version 1)]; 2024. doi: 10.21203/rs.3.rs-5504223/v1
- Embryo Ranking Intelligent Classification Algorithm. C. to, “Embryo Ranking Intelligent Classification Algorithm; 2020. Available from: https://en.wikipedia.org/wiki/embryo_ ranking_intelligent_classification_algorithm? [Last accessed on 2025 Feb 19].
- Leahy B, Jang WD, Yang H, et al. Automated Measurements of Key Morphological Features of Human Embryos for IVF. Med Image Comput Comput Assist Interv. 2020:12265:25-35. doi: 10.1007/978-3-030-59722-1_3
- Mushtaq A, Mumtaz M, Raza A, Salem N, Yasir MN. Artificial intelligence-based detection of human embryo components for assisted reproduction by in vitro fertilization. Sensors. 2022;22(19):7418. doi: 10.3390/s22197418
- Allahbadia GN. Embryo transfer is the last frontier for deep machine learning artificial intelligence in medically assisted reproduction (MAR). J Reprod. 2023;2(1):18. doi: 10.58779/issn.2954-467X.tjor2023.v2.n1.18
- Liu R, Bai S, Jiang X, et al. Multifactor prediction of embryo transfer outcomes based on a machine learning algorithm. Front Endocrinol (Lausanne). 2021;12:745039. doi: 10.3389/fendo.2021.745039
- Curchoe CL, Bormann CL. Artificial intelligence and machine learning for human reproduction and embryology presented at ASRM and ESHRE 2018. J Assist Reprod Genet. 2019;36(4):591-600. doi: 10.1007/s10815-019-01408-x
- Mills C, Johnston M, Koplin J. O-220 Artificial intelligence for embryo selection: Ethical, social and regulatory issues. Hum Reprod. 2023;38(Suppl 1):dead093.266. doi: 10.1093/humrep/dead093.266
- Ahlström A, Berntsen J, Johansen M, et al. Correlations between a deep learning-based algorithm for embryo evaluation with cleavage-stage cell numbers and fragmentation. Reprod Biomed Online. 2023;47(6):103408. doi: 10.1016/j.rbmo.2023.103408
- Nasr M, Mohamed M, Shehata L. Artificial intelligence in assisted reproductive technology review. Int J Progress Sci Technol. 2021;25:507-11.
- Barrie A, Homburg R, McDowell G, Brown J, Kingsland C, Troup S. Examining the efficacy of six published time-lapse imaging embryo selection algorithms to predict implantation to demonstrate the need for the development of specific, in-house morphokinetic selection algorithms. Fertil Steril. 2017;107(3):613-621. doi: 10.1016/j.fertnstert.2016.11.014
- Fitz VW, Kanakasabapathy MK, Thirumalaraju P, et al. Should there be an “AI” in TEAM? Embryologists selection of high implantation potential embryos improves with the aid of an artificial intelligence algorithm. J Assist Reprod Genet. 2021;38(10):2663-2670. doi: 10.1007/s10815-021-02318-7