Artificial intelligence in cardiac rhythm diagnostics and management: Challenges and opportunities
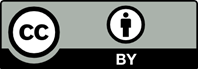
Each day, one million people undergo electrocardiogram diagnostics. The diagnostic process is time-consuming and often yields incomplete or inconclusive results, placing significant strain on physicians. Artificial intelligence (AI)-assisted diagnosis can significantly alleviate this burden by enhancing diagnostic accuracy and efficiency, and its application is gaining traction across various fields. With the increasing number of patients and a growing backlog of diagnostic appointments, AI can offer physicians benefits such as accurate, timely, and reliable assistance in reviewing vital signs and conducting physical examinations for individual patients. As physicians face mounting pressure from insurance companies and government guidelines for consultation time, AI can help streamline the diagnostic process. In particular, with the growing global attention on cardiac health (and the overall decline thereof), the range of automated diagnostic opportunities is expanding rapidly. Additional mathematical processing tools can provide probabilistic assessments of various cardiac conditions, reducing physicians’ workload while enhancing treatment options. AI has already demonstrated success in expediting the detection of pathological cardiac depolarization abnormalities and shortening diagnostic time frames. However, AI-based diagnostics requires further validation and safeguards to minimize diagnostic inaccuracies, ensuring its reliability and safety in clinical practice.
- Empana JP, Lerner I, Valentin E, et al. Incidence of Sudden cardiac death in the European Union. J Am Coll Cardiol. 2022;79(18):1818-1827. doi: 10.1016/j.jacc.2022.02.041
- Zwietering PJ, Knottnerus JA, Rinkens PE, Kleijne MA, Gorgels AP. Arrhythmias in general practice: Diagnostic value of patient characteristics, medical history and symptoms. Fam Pract. 1998;15:343-353. doi: 10.1093/fampra/15.4.343
- Hoefman E, Van Weert HCPM, Reisma JB, Koster RW, Bindels PJE. Diagnostic yield of patient-activated loop recorders for detecting heart rhythm abnormalities in general practice: A randomised clinical trial. Fam Pract. 2005;22:478-484. doi: 10.1093/fampra/cmi048
- Hoefman E, Bindels PJE, Van Weert HCPM. Efficacy of diagnostic tools for detecting cardiac arrhythmias: Systemic literature search. Neth Heart J. 2010;18:543-551. doi: 10.1007/s12471-010-0831-0
- Auer R, Bauer DC, Marques-Vidal P, Butler J, Min LJ, Cornuz J. Association of major and minor ECG abnormalities with coronary heart disease events. JAMA. 2012;307:1497-1505. doi: 10.1001/jama.2012.434
- Mai VT, Alattas KA, Bouteraa Y, Ghaderpour E, Mohammadzadeh A. Personalized blood pressure control by machine learning for remote patient monitoring. IEEE Access. 2024;12:83994-84004. doi: 10.1109/ACCESS.2024.3413572
- Cheng CH, Wong KW, Chin JW, Chan TT, So RHY. Deep learning methods for remote heart rate measurement: A review and future research agenda. Sensors. 2021;21(18):6296. doi: 10.3390/s21186296
- Androulakis E, Fielder C. Artificial Intelligence in ECG Diagnostics-where are we now? European Society of Cardiology; 2024. Available from: https://www.escardio. org/councils/council-for-cardiology-practice-(ccp)/ cardiopractice/artificial-intelligence-in-ecg-diagnostics-where-are-we-now [Last accessed on 2025 Mar 18].
- Sau A, Pastika L, Sieliwonczyk E, et al. Artificial intelligence-enabled electrocardiogram for mortality and cardiovascular risk estimation: A model development and validation study. Lancet Digit Health. 2024;6(11):e791-e802. doi: 10.1016/S2589-7500(24)00172-9
- Siontis KC, Noseworthy PA, Attia ZI, Friedman PA. Artificial intelligence-enhanced electrocardiography in cardiovascular disease management. Nat Rev Cardiol. 2021;18(7):465-478. doi: 10.1038/s41569-020-00503-2
- Najarian K, Splinter R. Biomedical Signal and Image Processing. 2nd ed. Boca Raton, FL: CRC Press; 2012. doi: 10.1201/b11978
- Einthoven W. Galvanometrische registratie van het menschelijk electrocardiogram. In: Herinneringsbundel Prof. S.S. Rosenstern. Leiden: Eduard Ijdo; 1902. p. 101-106.
- Einthoven W. Het tele-cardiogram. Ned Tijdschr Geneeskd. 1906;22:1517-1547.
- Attia ZI, Noseworthy PA, Lopez-Jimenez F, et al. An artificial intelligence-enabled ECG algorithm for the identification of patients with atrial fibrillation during sinus rhythm: A retrospective analysis of outcome prediction. Lancet. 2019;394(10201):861-867.
- Yusuf SW, Durand JB, Lenihan DJ, Swafford J. Dextrocardia: An incidental finding. Tex Heart Inst J. 2009;36(4):358-359.
- Bernasconi A, Azancot A, Simpson JM, Jones A, Sharland GK. Fetal dextrocardia: Diagnosis and outcome in two tertiary centres. Heart. 2005;91(12):1590-1594. doi: 10.1136/hrt.2004.048330
- Garg N, Agarwal BL, Modi N, Radhakrishnan S, Sinha N. Dextrocardia: An analysis of cardiac structures in 125 patients. Int J Cardiol. 2003;88(2-3):143-156. doi: 10.1016/s0167-5273(02)00539-9
- Wiener N. The Interpolation, Extrapolation and Smoothing of Stationary Time Series’, Report of the Services 19, Research Project DIC-6037 MIT; 1942.
- Luengo-Fernandez R, Walli-Attaei M, Gray A, et al. Economic burden of cardiovascular diseases in the European Union: A population-based cost study. Eur Heart J. 2023;44(45):4752-4767. doi: 10.1093/eurheartj/ehad583
- Brown RG, Hwang PYC. Introduction to Random Signals and Applied Kalman Filtering. 3rd ed. New York, NY: John Wiley & Sons; 1996.
- Welch LR. Wiener-Hopf Theory 2006-11-25. Available from: https://csi.usc.edu/PDF/wienerhopf.pdf [Last accessed on 2025 Mar 18].
- Wiener N, Hopf E. Ueber Eine Klasse Singulärer Integralgleichungen. Berlin: Sitzungber. Akad. Wiss; 1931. p. 696-706.
- Wiener N. Extrapolation, Interpolation, and Smoothing of Stationary Time Series. New York NY: John Wiley & Sons; 1949.
- Kolmogorov AN. Stationary sequences in Hilbert space’, (In Russian) Bull. Moscow Univ. 1941;2(6):1-40. English translation In: Kailath T, editor. Linear Least Squares Estimation. Stroudsburg, PA; Dowden, Hutchinson & Ross; 1977.
- Tison GH, Zhang J, Delling FN, Deo RC. Automated and interpretable patient ECG profiles for disease detection, tracking, and discovery. Circ Cardiovasc Qual Outcomes. 2019;12(9):e005289. doi: 10.1161/CIRCOUTCOMES.118.005289
- Christopoulos G, Graff-Radford J, Lopez CL, et al. Artificial intelligence-electrocardiography to predict incident atrial fibrillation: A population-based study. Circ Arrhythm Electrophysiol. 2020;13(12):e009355. doi: 10.1161/CIRCEP.120.009355
- Yamashita R, Nishio M, Do RKG, Togashi K. Convolutional neural networks: An overview and application in radiology. Insights Imaging. 2018;9(4):611-629. doi: 10.1007/s13244-018-0639-9
- Rudin C. Stop explaining black box machine learning models for high stakes decisions and use interpretable models instead. Nat Mach Intell. 2019;1(5):206-215. doi: 10.1038/s42256-019-0048-x
- Ashok A, Babburi A, Ardra T, Gayathri KS, Indu RJ, Narayanan G. Performance Comparison of Matched Filter, Wavelet Denoising and Wiener Filter Technique in Communication Receivers. In: 2018 3rd IEEE International Conference on Recent Trends in Electronics, Information and Communication Technology (RTEICT). doi: 10.1109/rteict42901.2018.9012
- Proakis JG, Manolakis DG. Digital Signal Processing. Available from: https://uvceee.wordpress.com/wp-content/ uploads/2016/09/digital_signal_processing_principles_ algorithms_and_applications_third_edition.pdf [Last accessed on 2025 Mar 18].
- Bhattacharyya SS, Deprettere EF, Leupers R, Takala J. Handbook of Signal Processing Systems. 3rd ed. New York, NY: Springer; 2019. Available from: https://link.springer.com/ book/10.1007/978-3-319-91734-4 [Last accessed on 2025 Mar 18].
- Lyons RG. Understanding Digital Signal Processing. Hoboken, NJ: Prentics Hall; 2010.
- Casasent D, Chang WT. Correlation synthetic discriminant functions. Appl Optics. 1986;25(14):2343-2350. doi: 10.1364/AO.25.002343
- Holton T. Digital Signal Processing, Principles and Applications. Cambridge, UK: Cambridge University Press; 2021. doi: 10.1017/9781108290050
- Alizadehsani R, Roshanzamir M, Hussain S, et al. Handling of uncertainty in medical data using machine learning and probability theory techniques: A review of 30 years (1991- 2020). Ann Oper Res. 2021;21:1-42. doi: 10.1007/s10479-021-04006-2
- Hannun AY, Rajpurkar P, Haghpanahi M, et al. Cardiologist-level arrhythmia detection and classification in ambulatory electrocardiograms using a deep neural network. Nat Med. 2019;25(1):65-69. doi: 10.1038/s41591-018-0268-3
- Turin GL. An introduction to matched filters. IRE Trans Inform Theory. 1960;6(3):311-332. doi: 10.1109/TIT.1960.1057571
- Davenpoort MA, Boufounos P, Wakin M, Baraniuk R. Signal processing with compressive measurements. IEEE J Select Top Signal Process. 2010;4(2):445-460. doi: 10.1109/JSTSP.2009.2039178
- Wimalajeewa T, Varshney PK. Sparse signal detection with compressive measurements via partial support set estimation. IEEE Trans Signal Inform Process Over Netw. 2017;3(1):46-60. doi: 10.1109/TSIPN.2016.2601025
- Fornasier M, Rauhut H. Compressive sensing. In: Scherzer O, editor. Handbook of Mathematical Methods in Imaging. New York, NY: Springer; 2011. doi: 10.1007/978-0-387-92920-0_6
- Cauchy AL. Extrait du Mémoire sur Quelques Séries Analogues À La Série de Lagrange, sur les Fonctions Symétriques, et sur la Formation Directe des Équations Que Produit L’élimination des Inconnues Entre des Équations Algébriques Données. Mémoires de l’Académie des Sciences, t. IX; 1830. p. 73-78. Available from: https://gallica.bnf.fr/ark:/12148/bpt6k901828/f79 [Last accessed on 2025 Mar 18].
- Steele JM. The Cauchy-Schwarz Master Class: An Introduction to the Art of Mathematical Inequalities. The Mathematical Association of America. Cambridge, UK: Cambridge University Press; 2004.
- Steiger WL. On a generalization of the Cauchy-Schwarz in equality. Am Math Monthly. 1969;76:815-816. doi: 10.1080/00029890.1969.12000339
- Pishro-Nik H. Introduction to Probability, Statistics, and Random Processes. Sunderland MA: Kappa Research LLC; 2014. Available from: https://www.probabilitycourse.com [Last accessed on 2025 Mar 18].
- Shamloo AS. Risk assessment in cardiac arrhythmias. Eur Heart J. 2020;41(47):4455-4457. doi: 10.1093/eurheartj/ehaa808
- Takase B, Ikeda T, Shimizu W, et al. JCS/JHRS 2022 guideline on diagnosis and risk assessment of arrhythmia. J Arrhythm. 2024;40(4):655-752. doi: 10.1002/joa3.13052
- Redfield MM, Jacobsen SJ, Burnett JC Jr., Mahoney DW, Bailey KR, Rodeheffer RJ. Burden of systolic and diastolic ventricular dysfunction in the community: Appreciating the scope of the heart failure epidemic. JAMA. 2003;289(2):194-202. doi: 10.1001/jama.289.2.194
- McDonagh TA, Metra M, Adamo M, et al. 2023 Focused Update of the 2021 ESC Guidelines for the diagnosis and treatment of acute and chronic heart failure. Eur Heart J. 2023;44(37):3627-3639. doi: 10.1093/eurheartj/ehad195
- Attia ZI, Kapa S, Lopez-Jimenez F, et al. Screening for cardiac contractile dysfunction using an artificial intelligence-enabled electrocardiogram. Nat Med. 2019;25(1):70-74. doi: 10.1038/s41591-018-0240-2
- Ribeiro AH, Ribeiro MH, Paixão GMM, et al. Automatic diagnosis of the 12-lead ECG using a deep neural network. Nat Commun. 2020;11(1):1760. doi: 10.1038/s41467-020-15432-4
- Rawi AA, Elbashir MK, Ahme AM. Classification of 27 heart abnormalities using 12-lead ECG signals with combined deep learning techniques. Bull Electrical Eng Inform. 2023;12(4):2220-2235. doi: 10.11591/eei.v12i4.4668
- Attia ZI, Kapa S, Yao X, et al. Prospective validation of a deep learning electrocardiogram algorithm for the detection of left ventricular systolic dysfunction. J Cardiovasc Electrophysiol. 2019;30(5):668-674. doi: 10.1111/jce.13889
- Kwon JM, Kim KH, Jeon KH, et al. Development and validation of deep-learning algorithm for electrocardiography-based heart failure identification. Korean Circ J. 2019;49(7):629-639. doi: 10.1097/mat.0000000000001218
- Cho J, Lee B, Kwon JM, et al. Artificial intelligence algorithm for screening heart failure with reduced ejection fraction using electrocardiography. ASAIO J. 2021;67(3):314-321. doi: 10.1097/mat.0000000000001218
- Pickham D, Zarafshar S, Sani D, Kumar N, Froelicher V. Comparison of three ECG criteria for athlete pre-participation screening. J Electrocardiol. 2014;47(6):769-774. doi: 10.1016/j.jelectrocard.2014.07.019
- Sheikh N, Papadakis M, Ghani S, et al. Comparison of electrocardiographic criteria for the detection of cardiac abnormalities in elite black and white athletes. Circulation. 2014;129(16):1637-1649. doi: 10.1161/CIRCULATIONAHA.113.006179
- Ko WY, Siontis KC, Attia ZI, et al. Detection of hypertrophic cardiomyopathy using a convolutional neural network-enabled electrocardiogram. J Am Coll Cardiol. 2020;75(7):722-733. doi: 10.1016/j.jacc.2019.12.030
- Kwon JM, Jeon KH, Kim HM, et al. Comparing the performance of artificial intelligence and conventional diagnosis criteria for detecting left ventricular hypertrophy using electrocardiography. Europace. 2020;22(3):412-419. doi: 10.1093/europace/euz324
- Han L, Askari M, Altman RB, et al. Atrial fibrillation burden signature and near-term prediction of stroke: A machine learning analysis. Circ Cardiovasc Qual Outcomes. 2019;12(10):e005595. doi: 10.1161/CIRCOUTCOMES.118.005595
- Bos JM, Attia ZI, Albert DE, Noseworthy PA, Friedman PA, Ackerman MJ. Use of artificial intelligence and deep neural networks in evaluation of patients with electrocardiographically concealed long QT syndrome from the surface 12-lead electrocardiogram. JAMA Cardiol. 2021;6(5):532-538. doi: 10.1001/jamacardio.2020.7422
- Aufiero S, Bleijendaal H, Robyns T, et al. A deep learning approach identifies new ECG features in congenital long QT syndrome. BMC Med. 2022;20(1):162. doi: 10.1186/s12916-022-02350-z
- Available from: https://www.biopac.com/knowledge-base/ecg-analysis [Last accessed on 2025 Mar 18].
- Vahanian A, Beyersdorf F, Praz F, et al. 2021 ESC/EACTS guidelines for the management of valvular heart disease. Eur Heart J. 2022;43(7):561-632. doi: 10.1093/eurheartj/ehab395
- Kwon JM, Kim KH, Akkus Z, Jeon KH, Park J, Oh BH. Artificial intelligence for detecting mitral regurgitation using electrocardiography. J Electrocardiol. 2020;59:151-157. doi: 10.1016/j.jelectrocard.2020.02.008
- Elias P, Poterucha TJ, Rajaram V, et al. Deep learning electrocardiographic analysis for detection of left-sided valvular heart disease. J Am Coll Cardiol. 2022;80(6): 613-626. doi: 10.1016/j.jacc.2022.05.029
- Splinter R, editor. Handbook of Physics in Medicine and Biology. 1st ed. Baco Raton, FL: CRC Press; 2010. doi: 10.1201/9781420075250
- How are Arrhythmias Treated? National Heart, Lung, and Blood Institute; 2011. Available from: https://www.nhlbi.nih. gov/health/arrhythmias/treatment [Last accessed on 2025 Mar 18].
- Saljic A, Heijman J, Dobrev D. Recent advances in antiarrhythmic drug therapy. Drugs. 2023;83(13):1147-1160. doi: 10.1007/s40265-023-01923-3
- Tettey-Engmann FA, Parupelli SK, Desai SS. A review of biomedical devices: Classification, regulatory guidelines, human factors, software as a medical device, and cybersecurity. Biomed Mater Dev Biomed Mater Dev. 2024;2:316-341. doi: 10.1007/s44174-023-00113-9
- Diagnosing Arrhythmias; Arrhythmia Alliance. Available from: https://www.lhch.nhs.uk/media/. resources/64a7ecda3bd825.77246728.pdf [Last accessed on 2025 Mar 18].
- Desai DS, Hajouli S. Arrhythmias. St. Petersburg, FL, USA: StatPearls Publishing; 2025. Available from: https://www. ncbi.nlm.nih.gov/books/NBK558923 [Last accessed on 2025 Mar 18].
- Classification of cardiac arrhythmias and conduction disturbances. Am Heart J. 1979;98(2):263-267. doi: 10.1016/0002-8703(79)90230-8
- Antzelevitch C, Burashnikov A. Overview of basic mechanisms of cardiac arrhythmia. Card Electrophysiol Clin. 2011;3(1):23-45. doi: 10.1016/j.ccep.2010.10.012
- Kiladze MR, Lyakhova UA, Lyakhov PA, Nagornov NN, Vahabi M. Multimodal neural network for recognition of cardiac arrhythmias based on 12-load electrocardiogram signals. IEEE Access. 2023;11:133744-133754. doi: 10.1109/ACCESS.2023.3335176