Screening and early detection of cervical intraepithelial neoplasia and cervicitis using a hemoglobin absorption map-derived machine learning algorithm
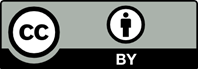
Early and non-invasive detection of cervical malignancy holds great clinical significance. Diffuse reflectance (DR) spectroscopy has the capability to map tissue transformation at the biochemical, morphological, and cellular levels. We have developed a non-invasive, multimodal imaging system to map changes in tissue autofluorescence using DR for the screening and early detection of cervical cancer and cervical inflammation (cervicitis). The developed multispectral imaging device consists of light-emitting diodes (LED) emitting at 375, 545, 575, and 610 nm wavelengths, along with a 5-megapixel monochrome camera for image acquisition. Camera operation and image analysis are controlled using proprietary software installed on a Windows tablet. The 375 nm LED-excited autofluorescence, and the elastically backscattered light at 545, 575, and 610 nm originating from the cervix tissue are captured by the camera and processed to assess tissue abnormalities. A machine learning (ML) algorithm based on DR image intensity ratio values was developed for tissue classification. It was observed that the R610/R545 image ratio could discriminate malignant cervical sites from normal tissues, achieving a sensitivity of 100% and specificity of 93%. In comparison, cervicitis could be discriminated from normal tissues using the R610/R575 ratio, with a sensitivity of 91.6% and specificity of 94.4%. The study demonstrates the potential of DR imaging in conjunction with ML algorithm to non-invasively screen and detect cervical intraepithelial neoplasia and cervicitis in real time. As compared to the existing practice of Pap smear and colposcopy-directed biopsy, which are subjective and require a waiting period for results, objective screening using CerviScan would help reduce patient anxiety, unnecessary biopsies, and treatment costs. With increased patient screening, the accuracy of the ML algorithm would improve. When integrated into a cloud server, the system could address the needs of multiple users in a field setting.
- Ferlay J, Soerjomataram I, Dikshit R, et al. Cancer incidence and mortality worldwide: Sources, methods and major patterns in GLOBOCAN 2012. Int J Cancer. 2015;136(5):E359-E386. doi: 10.1002/ijc.29210
- Denny L. Cervical cancer: Prevention and treatment. Discov Med. 2012;14(75):125-131.
- Sankaranarayanan R, Ramadas K. Socioeconomic factors and cancer prevention in India: Diverse interventions are needed. In: World Cancer Report Cancer Research for Cancer Prevention. Vol. 4.4. France: International Agency for Research on Cancer; 2020. p. 258-265.
- Leyden WA, Manos MM, Geiger AM, et al. Cervical cancer in women with comprehensive health care access: Attributable factors in the screening process. J Natl Cancer Inst. 2005;97(9):675-683. doi: 10.1093/jnci/dji115
- Barut MU, Kale A, Kuyumcuoğlu U, et al. Analysis of sensitivity, specificity, and positive and negative predictive values of smear and colposcopy in diagnosis of premalignant and malignant cervical lesions. Med Sci Monit. 2015;21: 3860-3867. doi: 10.12659/MSM.895227
- Rahmadwati G, Naghdy G, Ross M, Todd C, Norachmawati E. Classification of Cervical Cancer Using Histology Images. In: 2010 Second International Conference on Computer Engineering and Applications. Bali, Indonesia: IEEE; 2010. p. 515-519. doi: 10.1109/ICCEA.2010.105
- Bosch FX, Burchell AN, Schiffman M, et al. Epidemiology and natural history of human papillomavirus infections and type-specific implications in cervical neoplasia. Vaccine. 2008;26(Suppl 10):K1-K16. doi: 10.1016/j.vaccine.2008.05.064
- Narayanan S, Anand S, Prasanna R, et al. Bimodal multispectral imaging system with cloud-based machine learning algorithm for real-time screening and detection of oral potentially malignant lesions and biopsy guidance. J Biomed Opt. 2021;26(8):086003. doi: 10.1117/1.JBO.26.8.086003
- Prasad PN. Introduction to Biophotonics. 1st ed. United States: John Wiley and Sons; 2003.
- Prabitha VG, Suchetha S, Jayanthi JL, et al. Detection of cervical lesions by multivariate analysis of diffuse reflectance spectra: A clinical study. Lasers Med Sci. 2016;31(1):67-75. doi: 10.1007/s10103-015-1829-z
- Mirabal YN, Chang SK, Atkinson EN, Malpica A, Follen M, Richards-Kortum R. Reflectance spectroscopy for in vivo detection of cervical precancer. J Biomed Opt. 2002;7(4): 587-594. doi: 10.1117/1.1502675
- Shaikh R, Prabitha VG, Dora TK, et al. A comparative evaluation of diffuse reflectance and Raman spectroscopy in the detection of cervical cancer. J Biophotonics. 2016;10(2):242-252. doi: 10.1002/jbio.201500248
- Prabitha VG, Suchetha S, Jayanthi JL, et al. Multi-spectral diffuse reflectance imaging for detection of cervical lesions: A pilot study. Int J Eng Sci Innov Technol. 2014;3(6):169-177.
- Messadi DV, Younai FS, Liu HH, Guo G, Wang CY. The clinical effectiveness of reflectance optical spectroscopy for the in vivo diagnosis of oral lesions. Int J Oral Sci. 2014;6: 162-167. doi: 10.1038/ijos.2014.39
- Stephen MM, Jayanthi JL, Unni NG, et al. Diagnostic accuracy of diffuse reflectance imaging for early detection of pre-malignant and malignant changes in the oral cavity: A feasibility study. BMC Cancer. 2013;13:278. doi: 10.1186/1471-2407-13-278
- Prasanth CS, Stephen MM, Dora TK, Maheshwari A, Subhash N. Non-invasive detection of periodontal disease using diffuse reflectance spectroscopy: A clinical study. In: Biomedical Applications of Light Scattering VI. Vol. 8230. Bellingham: SPIE; 2012. p. 117-127.
- Prasanth CS, Betsy J, Jayanthi JL, Nisha UG, Prasantila J, Subhash N. In vivo inflammation mapping of periodontal disease based on diffuse reflectance spectral imaging: A clinical study. J Biomed Opt. 2013;18(2):026019. doi: 10.1117/1.JBO.18.2.026019
- Chandra Sekhar P, Betsy J, Presanthila J, Subhash N. Discrimination of periodontal diseases using diffuse reflectance spectral intensity ratios. J Biomed Opt. 2012;17(2):027001. doi: 10.1117/1.JBO.17.2.027001
- Barik AK, Pavithran MS, Nelliat M, et al. Laser induced fluorescence of cervical tissues: An in vitro study for the diagnosis of cervical cancer from the cervicitis. J Opt. 2022;24(5):054002. doi: 10.1088/2040-8986/ac59e2
- Zhang H, Chen C, Ma C, et al. Feature fusion combined with Raman spectroscopy for early diagnosis of cervical cancer. IEEE Photonics J. 2021;13(3):3900311. doi: 10.1109/JPHOT.2021.3075958
- Huang W, Sun S, Yu Z, Lu S, Feng H. Chronic cervicitis and cervical cancer detection based on deep learning of colposcopy images toward translational pharmacology. Front Pharmacol. 2022;13:911962. doi: 10.3389/fphar.2022.911962
- Dong B, Lu Z, Yang T, et al. Development, validation, and clinical application of a machine learning model for risk stratification and management of cervical cancer screening based on full-genotyping hrHPV test (SMART-HPV): A modelling study. Lancet Reg Health West Pac. 2025;55:101480. doi: 10.1016/j.lanwpc.2025.101480
- Wekalao J, Kumaresan MS, Mallan S, et al. Metasurface based surface plasmon resonance (SPR) biosensor for cervical cancer detection with behaviour prediction using machine learning optimization based on support vector regression. Plasmonics. 2024:1-24. doi: 10.1007/s11468-024-02623-8
- Sarhangi HA, Beigifard D, Farmani E, Bolhasani H. Deep learning techniques for cervical cancer diagnosis based on pathology and colposcopy images. Inform Med Unlocked. 2024;47:101503. doi: 10.1016/j.imu.2024.101503
- El-Nashar SA, Mariani A. Uterine carcinosarcoma. Clin Obstet Gynecol. 2011;54(2):292-304. doi: 10.1097/GRF.0b013e31821ac635
- Gupta N, Dudding N, Smith JHF. Eight cases of malignant mixed Müllerian tumor (carcinosarcoma) of the uterus: Findings in SurePath™ cervical cytology. Diagn Cytopathol. 2014;42(2):165-169. doi: 10.1002/dc.22910
- Arbyn M, Weiderpass E, Bruni L, et al. Estimates of incidence and mortality of cervical cancer in 2018: A worldwide analysis. Lancet Glob Health. 2020;8(2):e191-e203. doi: 10.1016/S2214-109X(19)30482-6
- Nordstrom RJ, Burke L, Niloff JM, Myrtle JF. Identification of cervical intraepithelial neoplasia (CIN) using UV‐excited fluorescence and diffuse‐reflectance tissue spectroscopy. Lasers Surg Med. 2001;29(2):118-127. doi: 10.1002/lsm.1097
- Najib FS, Hashemi M, Shiravani Z, Poordast T, Sharifi S, Askary E. Diagnostic accuracy of cervical pap smear and colposcopy in detecting premalignant and malignant lesions of cervix. Indian J Surg Oncol. 2020;11(3):453-458. doi: 10.1007/s13193-020-01118-2