Dental cavity analysis, prediction, localization, and quantification using computer vision
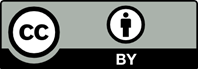
Dental health assessment is a critical component of overall well-being, and advancements in computer vision and deep learning have opened new avenues for automating and enhancing this process. In this study, we present a comprehensive approach to dental cavity analysis, spanning localization, quantification, and visualization. Our methodology leveraged a diverse dataset of colored dental images that had been meticulously augmented and annotated. The You Only Look Once model was employed for precise dental cavity localization, providing bounding box predictions. Remarkably, these results were obtained based on images from standard device cameras. Subsequently, we introduced the use of the segment anything model segmentation model, known for its zero-shot generalization capabilities, to focus on the exact areas of dental cavities. This approach enhanced the granularity of our analysis, providing dental professionals with detailed visualizations for precise diagnosis. During the quantification phase, we extracted cavity areas from bounding box coordinates, enabling accurate measurement of cavity sizes. The model achieved a notable mean average precision of 0.732, an accuracy of 0.789, and a recall of 0.701. Moreover, the model converged quickly, with most metrics achieving near-optimal results after 100 iterations. This quantitative data augments traditional diagnosis methods, facilitating more informed treatment decisions.
- Pruthi S. A Window to Your Overall Health, Oral Health. United States: Mayo Clinic; 2024.
- Global Burden of Disease (GBD). Institute for Health Metrics and Evaluation, IHME; 2019.
- Kim SM, Jang WM, Ahn HA, Park HJ, Ahn HS. Korean National Health Insurance value incentive program: Achievements and future directions. J Prev Med Public Health. 2012;45:148-155. doi: 10.3961/jpmph.2012.45.3.148
- Maru AM, Narendran S. Epidemiology of dental caries among adults in a rural area in India. J Contemp Dent Pract. 2012;13(3):382-388. doi: 10.5005/jp-journals-10024-1155
- Shankar V, Narang U. Emerging market innovations: Unique and differential drivers, practitioner implications, and research agenda. J Acad Mark Sci. 2020;48:1030-1052. doi: 10.1007/s11747-019-00685-3
- Silvertown JD, Wong BP, Abrams SH, Sivagurunathan KS, Mathews SM, Amaechi BT. Comparison of the canary system and DIAGNOdent for the in vitro detection of caries under opaque dental sealants. J Investig Clin Dent. 2017;8(4). doi: 10.1111/jicd.12239
- Almalki YE, Imam Din A, Ramzan M, et al. Deep learning models for classification of dental diseases using orthopantomography X-ray OPG images. Sensors (Basel). 2022;22(19):7370. doi: 10.3390/s22197370
- Retrouvey JM, Conley RS. Decoding deep learning applications for diagnosis and treatment planning. Dent Press J Orthod. 2023;27. doi: 10.1590/2177-6709.27.5.e22spe5
- Kumar S, Kumar H. Analysis of image segmentation techniques for dental radiography. Element Educ Online. 2021;20(4):3868-3875. doi: 10.17051/ilkonline.2021.04.422
- Tareq A, Faisal MI, Islam S, et al. Visual diagnostics of dental caries through deep learning of non-standardised photographs using a hybrid YOLO ensemble and transfer learning model. Int J Environ Res Public Health. 2023;20:5351. doi: 10.3390/ijerph20075351
- Thanh MT, Van Toan N, Ngoc VT, Tra NT, Giap CN, Nguyen DM. Deep learning application in dental caries detection using intraoral photos taken by smartphones. Appl Sci. 2022;12(11):5504. doi: 10.3390/app12115504
- Rizzoli A. 6 Innovative Artificial Intelligence Applications in Dentistry; 2021. Available from: https://www.v7labs.com/ blog/ai-in-dentistry [Last accessed on 2021 Oct 26].
- Nirzu. Dental Disease Detection from Panoramic Dental X-ray. Available from: https://github.com/nirzu97/project-dental-disease-detection [Last accessed on 2021 Oct 26].
- Nakai K, Wei L. Recent advances in the prediction of subcellular localization of proteins and related topics. Front Bioinform. 2022;2:910531. doi: 10.3389/fbinf.2022.910531
- Acharya A. Guide to Image Segmentation in Computer Vision: Best Practices; 2022. Available from: https://encord. com/blog/image-segmentation-for-computer-vision-best-practice-guide [Last accessed on 2022 Nov 07].
- Brownlee J. A Gentle Introduction to Object Recognition with Deep Learning. Vol. 5. Machine Learning Mastery; 2019. p. 10. Available from: https://machinelearningmastery.com/ object-recognition-with-deep-learning [Last accessed on 2022 Nov 07].
- Fernandes AF, Dórea JR, Rosa GJ. Image analysis and computer vision applications in animal sciences: An overview. Front Vet Sci. 2020;7:551269. doi: 10.3389/fvets.2020.551269
- Chen B, Chen C, Hu J, et al. Computer vision and machine learning-based gait pattern recognition for flat fall prediction. Sensors (Basel). 2022;22:7960. doi: 10.3390/s22207960
- ForouzeshFar P, Safaei AA, Ghaderi F, Hashemikamangar SS. Dental caries diagnosis from bitewing images using convolutional neural networks. BMC Oral Health. 2024;24(1):211. doi: 10.1186/s12903-024-03973-9
- Park EY, Jeong S, Kang S, Cho J, Cho JY, Kim EK. Tooth caries classification with quantitative light-induced fluorescence (QLF) images using convolutional neural network for permanent teeth in vivo. BMC Oral Health. 2023;23(1):981. doi: 10.1186/s12903-023-03669-6
- Prados-Privado M, Garc Villalón JC, Martínez-Martínez CH, Ivorra C, Prados-Frutos JC. Dental caries diagnosis and detection using neural networks: A systematic review. J Clin Med. 2020;9(11):3579. doi: 10.3390/jcm9113579
- Kang IA, Ngnamsie Njimbouom S, Lee KO, Kim JD. DCP: Prediction of dental caries using machine learning in personalized medicine. Appl Sci. 2022;12(6):3043. doi: 10.3390/app12063043
- Albano D, Galiano V, Basile M, et al. Artificial intelligence for radiographic imaging detection of caries lesions: A systematic review. BMC Oral Health. 2024;24(1):274. doi: 10.1186/s12903-024-04046-7
- Park EY, Cho H, Kang S, Jeong S, Kim EK. Caries detection with tooth surface segmentation on intraoral photographic images using deep learning. BMC Oral Health. 2022;22(1):573. doi: 10.1186/s12903-022-02589-1
- Lian L, Zhu T, Zhu F, Zhu H. Deep learning for caries detection and classification. Diagnostics (Basel), 2021;11(9):1672. doi: 10.3390/diagnostics11091672
- Alharbi SS, AlRugaibah AA, Alhasson HF, Khan RU. Detection of cavities from dental panoramic x-ray images using nested u-net models. Appl Sci. 2023;13(23):12771. doi: 10.3390/app132312771
- Sikri A, Sikri J, Piplani V, Thakur Y. Applications of artificial intelligence in dentistry: A narrative review. South Asian Res J Oral Dent Sci. 2024;6(1):1-10. doi: 10.36346/sarjods.2024.v06i01.001
- Zhou X, Yu G, Yin Q, Liu Y, Zhang Z, Sun J. Context aware convolutional neural network for children caries diagnosis on dental panoramic radiographs. Comput Math Methods Med. 2022;2022:6029245. doi: 10.1155/2022/6029245