Optimizing electronic health records to support artificial intelligence
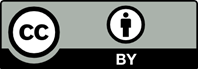
Electronic health records (EHRs) provide the most important data sources for artificial intelligence (AI). Gaining access to quality data suitable for advanced analytics continues to be challenging. This rapid review documents the current state of available data; identifies foundational AI data/information needs; and explores the benefits of adopting new and emerging technologies to design and implement next-generation EHRs. Opportunities to optimize EHRs for AI purposes are identified. This review was informed by expert knowledge and shared experiences supported by the literature, including technical standards. Main findings include poor ecosystem-wide infrastructures due to the lack of adopting the right set of standards, and current data and knowledge governance no longer fit for purpose. While many jurisdictions are continuing the use of legacy systems, some forward-looking national health systems and health-care facilities are adopting transformational strategies by adopting a strong data and digital focus to transition to new-generation systems. New foundational-level national infrastructures with strong leadership and governance are essential to enhance the governance and quality of available data, from collection at source throughout the entire data supply chain. Secure and ubiquitous access to high-quality EHR data at scale will foster the evolution of more intelligent and trustworthy AI. Key characteristics of next-generation EHRs supported by currently available technologies and standards that are able to meet digital era demands are provided in this paper. We conclude that the use of generative AI in clinical settings can only be reliably achieved when EHRs are optimized throughout the entire global digital health ecosystem.
- De Mello BH, Rigo SJ, Da Costa CA, et al. Semantic interoperability in health records standards: A systematic literature review. Health Technol (Berl). 2022;12(2):255-272. doi: 10.1007/s12553-022-00639-w
- Ingram D. Health Care in the Information Society. United Kingdom: OpenBook Publishers; 2023. doi: 10.11647/OBP.0335
- Hovenga E, Hullin C. Global collaborative leadership challenges and economic drivers. In: Hovenga E, Grain H, editors. Roadmap to Successful Digital Health Ecosystems. Ch. 3. United States: Academic Press; 2022. p. 35-63. doi: 10.1016/B978-0-12-823413-6.00001-X
- Heeks R. Health information systems: Failure, success and improvisation. Int J Med Inform. 2006;75(2):125-137. doi: 10.1016/j.ijmedinf.2005.07.024
- Justinia T. The UK’s National programme for IT: Why was it dismantled? Health Serv Manage Res. 2017;30(1):2-9. doi: 10.1177/0951484816662492
- Sadoughi F, Kimiafar K, Ahmadi M, Shakeri MT. Determining of factors influencing the success and failure of hospital information system and their evaluation methods: A systematic review. Iran Red Crescent Med J. 2013;15(12):e11716. doi: 10.5812/ircmj.11716
- Southon G, Sauer C, Dampney K. Lessons from a failed information systems initiative: Issues for complex organisations. Int J Med Inform. 1999;55(1):33-46. doi: 10.1016/s1386-5056(99)00018-0
- Johnstone R. Global Report Reveals Senior Officials Lack Understanding to Drive Digital Transformation of Government. Singapore: Global Government Forum; 2022 Available from: https://www.globalgovernmentforum.com/ global-report-reveals-senior-officials-lack-understanding-to-drive-digital-transformation-of-government [Last accessed on 2024 Mar 09].
- Wallace M, Sharfstein JM. The patchwork U.S. Public health system. N Engl J Med. 2022;386(1):1-4. doi: 10.1056/NEJMp2104881
- Steinkamp J, Kantrowitz JJ, Airan-Javia S. Prevalence and sources of duplicate information in the electronic medical record. JAMA Netw Open. 2022;5(9):e2233348. doi: 10.1001/jamanetworkopen.2022.33348
- Wang EC, Wright A. Characterizing outpatient problem list completeness and duplications in the electronic health record. J Am Med Inform Assoc. 2020;27(8):1190-1197. doi: 10.1093/jamia/ocaa125
- Madden JM, Lakoma MD, Rusinak D, Lu CY, Soumerai SB. Missing clinical and behavioral health data in a large electronic health record (EHR) system. J Am Med Inform Assoc. 2016;23(6):1143-1149. doi: 10.1093/jamia/ocw021
- Weiskopf NG, Hripcsak G, Swaminathan S, Weng C. Defining and measuring completeness of electronic health records for secondary use. J Biomed Inform. 2013;46(5):830-836. doi: 10.1016/j.jbi.2013.06.010
- Downing NL, Bates DW, Longhurst CA. Physician burnout in the electronic health record era: Are we ignoring the real cause? Ann Intern Med. 2018;169(1):50-51. doi: 10.7326/M18-0139
- Zhang J, Symons J, Agapow P, et al. Best practices in the real-world data life cycle. PLoS Digit Health. 2022;1(1):e0000003. doi: 10.1371/journal.pdig.0000003
- Zhang J, Morley J, Gallifant J, et al. Mapping and evaluating national data flows: Transparency, privacy, and guiding infrastructural transformation. Lancet Digit Health. 2023;5(10):e737-e748. doi: 10.1016/S2589-7500(23)00157-7
- HL7. Health Level Seven International. Available from: https://www.hl7.org [Last accessed on 2019 Feb 06].
- SNOMED-International. Leading Healthcare Terminology, Worldwide. Available from: https://www.snomed.org [Last accessed on 2024 Mar 10].
- DICOM. Digital Imaging and Communications in Medicine. Available from: https://www.dicomstandard.org [Last accessed on 2019 May 23].
- Beale T, Hovenga E. The knowledge-driven platform: Strategic technologies for a platform ecosystem approach. In: Hovenga E, Grain H, editors. Roadmap to Successful Digital Health Ecosystems. Ch. 6. United States: Academic Press; 2022. p. 115-141. doi: 10.1016/B978-0-12-823413-6.00006-9
- Hovenga E, Grain H, Beale T. Fragmented global standards development organisations. In: Hovenga E, Grain H, editors. Roadmap to Successful Digital Health Ecosystems. Ch. 4. United States: Academic Press; 2022. p. 65-96. doi: 10.1016/B978-0-12-823413-6.00025-2
- CDISC. Clinical Data Interchange Standards Consortium. Available from: https://www.cdisc.org [Last accessed on 2019 May 23].
- JIC. Joint Initiative Council for Global Health Informatics Standardisation. Available from: https://www. jointinitiativecouncil.org [Last accessed on 2024 Mar 10].
- ISO/TS_21564. 2019 Health Informatics-Terminology Resource Map Quality Measures (MapQual). Switzerland: Intenational Organisation for Standardisation; 2019. Available from: https://www.iso.org/standard/71088.html [Last accessed on 2019 Dec 06].
- OpenEHR. Archetype Technology Overview. London: OpenEHR Foundation. Available from: https:// specifications.openehr.org/releases/am/latest/overview. html [Last accessed on 2021 Jun 11].
- HL7-International. HL7 Version 3 Clinical Document Architecture (CDA) Release 2. Available from: http://www. hl7.org/implement/standards/product_brief.cfm?product_ id=7 [Last accessed on 2018 Sep 26].
- HL7-International. Fast Healthcare Interoperability Resources Specification (FHIR). Available from: https://www.hl7.org/ fhir/overview.html [Last accessed on 2024 Feb 28].
- Atalag K, Yang HY, Tempero E, Warren J. Model driven development of clinical information sytems using openEHR. Stud Health Technol Inform. 2011;169:849-853.
- Weeks J, Pardee R. Learning to share health care data: A brief timeline of influential common data models and distributed health data networks in U.S. health care research. EGEMS (Wash DC). 2019;7(1):4. doi: 10.5334/egems.279
- OpenEHR/CKM. Clinical Knowledge Manager. Available from: https://ckm.openehr.org/ckm/# [Last accessed on 2019 Feb 21].
- OpenEHR-Foundation. OpenEHR Reference Model (RM)- Latest. London: OpenEHR Foundation; 2017. Avaialble from: https://openehr.org [Last accessed on 2024 Jul 23].
- Cresswell KM, Mozaffar H, Lee L, Williams R, Sheikh A. Safety risks associated with the lack of integration and interfacing of hospital health information technologies: A qualitative study of hospital electronic prescribing systems in England. BMJ Qual Saf. 2017;26(7):530-541. doi: 10.1136/bmjqs-2015-004925
- Guo M, Wang Y, Yang Q, et al. Normal workflow and key strategies for data cleaning toward real-world data: Viewpoint. Interact J Med Res. 2023;12:e44310. doi: 10.2196/44310
- Stöger K, Schneeberger D, Kieseberg P, Holzinger A. Legal aspects of data cleansing in medical AI. Comput Law Secur Rev. 2021;42:105587. doi: 10.1016/j.clsr.2021.105587
- Scott P, Dunscombe R, Evans D, Mukherjee M, Wyatt J. Learning health systems need to bridge the “two cultures” of clinical informatics and data science. J Innov Health Inform. 2018;25:126-131. doi: 10.14236/jhi.v25i2.1062
- Cresswell K, Domínguez Hernández A, Williams R, Sheikh A. Key challenges and opportunities for cloud technology in health care: Semistructured interview study. JMIR Hum Factors. 2022;9(1):e31246. doi: 10.2196/31246
- HL7. Argonaut Project 2019. Available from: http://docs. smarthealthit.org/argonaut [Last accessed on 2019 Feb 06].
- CSIRO-eHealth-Research-Centre. SPARKED-AU FHIR Accelerator Brisbane; 2024. Available from: https://confluence.csiro.au/display/fhir/sparked+- +au+fhir+accelerator [Last accessed on 2024 Feb 17].
- ISO-13606-2. Health Informatics--Electronic Health Record Communication--Part 2: Archetype Interchange Specification: International Organsiation for Standardisation; 2008. Available from: https://www.iso.org/standard/50119.html [Last accessed on 2019 May 25].
- LOINC. Logical Observation Identifiers Names and Codes from Regenstrief. Indiana: Regenstrief. Available from: https://loinc.org [Last accessed on 2019 Feb 25].
- NSW-Government DN. AI Guidelines: Using Artifical Intelligence in the NSW Public Sector; 2023. Available from: https://www.education.gov.au/schooling/announcements/ australian-framework-generative-artificial-intelligence-ai-schools [Last accessed on 2024 Feb 16].
- NSW-Government. AI Assurance Framework; 2022. Available from: https://www.digital.nsw.gov.au/policy/ artificial-intelligence/nsw-artificial-intelligence-assurance-framework [Last accessed on 2024 Feb 16].
- AMA. Position Statement: Artifical Intelligence in Healthcare; 2023. Available from: https://www.ama.com.au/sites/ default/files/2023-08/artificial%20intelligence%20in%20 healthcare%20-%20ama.pdf [Last accessed on 2024 Feb 16].
- World Health Organisation. Regulatory Considerations on Artificial Intelligence for health. Geneva: World Health Organization; 2023. Available from: https://iris.who.int/ handle/10665/373421 [Last accessed on 2024 Feb 16].
- Australian-Government DoI, Science and Resources. Australia’s AI Ethics Principles. Available from: https:// www.industry.gov.au/publications/australias-artificial-intelligence-ethics-framework/australias-ai-ethics-principles [Last accessed on 2024 Feb 16].
- Australian-Government SDA. Guidelines for Secure AI System Development; 2023. Available from: https://www. cyber.gov.au/about-us/view-all-content/advice-and-guidance/guidelines-secure-ai-system-development [Last accessed on 2024 Feb 17].
- Australian-Government-DoH. National Health Reform Agreement (NHRA) 2020-25. Available from: https://www. health.gov.au/initiatives-and-programs/2020-25-national-health-reform-agreement-nhra [Last accessed on 2024 Feb 17].
- Data-Governance-Institute. The DGI-Data Governance Framework. Available from: https://datagovernance.com [Last accessed on 2024 Feb 16].
- The-White-House. Delivering on the Promise of AI to Improve Health Outcomes; 2023. Available from: https://www. whitehouse.gov/briefing-room/blog/2023/12/14/delivering-on-the-promise-of-ai-to-improve-health-outcomes [Last accessed on 2024 Feb 28].
- Government-of-the-Netherlands. Dutch Government Presents Vision on Generative AI; 2024. Available from: https://www.government.nl/latest/news/2024/01/18/ dutch-government-dutch-government-presents-vision-on-generative-ai#:~:text=the%20dutch%20government%20 has%20announced,via%20the%20dutch%20ai%20coalition [Last accessed on 2024 Feb 28].
- Elkin P, editor. Terminology, Ontology and their Implementations. 2nd ed. Berlin: Springer; 2023.
- ISO17115. Health Informatics-Representation of Categorial Structures of Terminology (CatStructure); 2020. Available from: https://www.iso.org/standard/73695.html [Last accessed on 2023 Dec 05].
- ISO18104. Health Informatics-Categorial Structures for Representation of Nursing Practice in Terminological Systems. Switzerland: International Organisation of Standards; 2023. Available from: https://www.iso.org/standard/81132.html [Last accessed on 2023 Dec 05].
- Hovenga E. Integrating a categorial structure for clinical practice into EHRs. Stud Health Technol Inform. 2024;310:74-78. doi: 10.3233/SHTI230930
- El-Sappagh S, Franda F, Ali F, Kwak KS. SNOMED CT standard ontology based on the ontology for general medical science. BMC Med Inform Decis Mak. 2018;18(1):76. doi: 10.1186/s12911-018-0651-5
- Rodrigues JM, Kumar A, Bousquet C, Trombert B. Using the CEN/ISO standard for categorial structure to harmonise the development of WHO international terminologies. Stud Health Technol Inform. 2009;150:255-259.
- ONC. Common Data Model Harmonisation. Available from: https://www.healthit.gov/topic/scientific-initiatives/pcor/ common-data-model-harmonization-cdm [Last accessed on 2024 Feb 21].
- Aldoseri A, Al-Khalifa KN, Hamouda AM. Re-thinking data strategy and integration for artificial intelligence: Concepts, opportunities, and challenges. Appl Sci. 2023;13(12):7082. doi: 10.3390/app13127082
- McCormick KA. The digital needs of genomics resulting from pandemics. In: Hovenga E, Grain H, editors. Roadmap to Successful Digital Health Ecosystems. Ch. 15. United States: Academic Press; 2022. p. 363-374. doi: 10.1016/B978-0-12-823413-6.00018-5
- Hassan M, Awan FM, Naz A, et al. Innovations in genomics and big data analytics for personalized medicine and health care: A review. Int J Mol Sci. 2022;23(9):4645. doi: 10.3390/ijms23094645
- Viceconti M, Hunter P, Hose R. Big data, big knowledge: Big data for personalized healthcare. IEEE J Biomed Health Inform. 2015;19(4):1209-1215. doi: 10.1109/JBHI.2015.2406883
- OpenEHR. An Open Domain-Driven Platform for Developing Flexible e-Health systems. London: OpenEHR Foundation. Available from: https://www.openehr.org [Last accessed on 2019 2019 Feb 06].
- Blobel B, Kalra D, Koehn M, et al. The role of ontologies for sustainable, semantically interoperable and trustworthy EHR solutions. Stud Health Technol Inform. 2009;150:953-957.
- Rector AL, Rogers J, Taweel A. Models and inference methods for clinical systems: A principled approach. Stud Health Technol Inform. 2004;107(Pt 1):79-83.
- Rector A, Schulz S, Rodrigues JM, Chute CG, Solbrig H. On beyond gruber: “Ontologies” in today’s biomedical information systems and the limits of OWL. J Biomed Inform. 2019;100:100002. doi: 10.1016/j.yjbinx.2019.100002
- Rector A, Johnson P, Tu SW, Wroe C, Rogers J, editors. Interface of Inference Models with Concept and Medical Record Models. In: AIME’01: Proceedings of the 8th Conference on AI in Medicine in Europe: Artifical Intelligence Medicine; 2001. doi: 10.1007/3-540-48229-6_43
- Rector AL. The interface between information, terminology, and inference models. Stud Health Technol Inform. 2001;84(Pt 1):246-250.
- Rector A, Marley T, Qamar R. Models of use and Model of Meaning Slideplayer; 2017. Available from: https:// slideplayer.com/slide/11210854 [Last accessed on 2024 Feb 29].
- ISO-23903. Health Informatics-Interoperability and Integration Reference Architecture-Model and Framework. Geneva: International Organization for Standards (ISO); 2021. Available from: https://www.iso.org/standard/77337. html [Last accessed on 2024 Jun 11].
- NHS-England. Digital Transformation. Available from: https://www.england.nhs.uk/digitaltechnology [Last accessed on 2024 Feb 28].
- Pedrera-Jiménez M, García-Barrio N, Frid S, et al. Can OpenEHR, ISO 13606, and HL7 FHIR work together? An agnostic approach for the selection and application of electronic health record standards to the next-generation health data spaces. J Med Internet Res. 2023;25:e48702. doi: 10.2196/48702
- OECD. Towards an Integrated Health Information System in the Netherlands. Paris: OECD Publishing; 2022. doi: 10.1787/a1568975-en
- Eit-Health E. Implementing the European Health Data Space in Sweden; 2023. Available from: https://eithealth.eu/wp-content/ uploads/2023/10/implementing-the-european-health-data-space-in-sweden.pdf [Last accessed on 2024 Feb 28].
- Government-of-Jamaica. Jamaica Poursues Digital Transformation of Health Sector: Ministry of Health and Welness; 2024 Available from: https://www.moh.gov.jm/ jamaica-pursues-digital-transformation-of-health-sector [Last accessed on 2024 Feb 21].
- Jones M. Caribbean/PAHO-Jamaican case study. In: Hovenga E, Grain H, editors. Roadmap to Successful Digital Health Ecosystems. Ch. 4. United States: Academic Press; 2022. p. 523-535. doi: 10.1016/B978-0-12-823413-6.00026-4
- eHAction. Common Semantic Strategy for Health in the European Union; 2019 Available from: http://ehaction.eu/ eu-common-semantic-strategy-open-consultation [Last accessed on 2024 Feb 28].
- CODE-Center-for-Open-Data-Enterprise. Sharing and Utilizing Health Data for AI Applications-Round Table Report; 2019. Available from: https://www.hhs.gov/sites/ default/files/sharing-and-utilizing-health-data-for-ai-applications.pdf [Last accessed on 2024 Feb 22].
- ISO13606. The ISO 13606 Standard Explained; 2019. Available from: http://www.en13606.org/information.html [Last accessed on 2024 Feb 18].
- OpenEHR. Specifications. Available from: https:// specifications.openehr.org [Last accessed on 2021 Apr 25].
- Atalag K, Beale T, Chen R, Gornik T, Heard S, McNicoll I. OpenEHR-A Semantically-Enabled, Vendor-Independent Health Computing Platform-White Paper; 2017. Avaialble from: https://www.openehr.org/resources/white_paper_docs/openEHR_vendor_independent_platform.pdf [Last accessed on 2017 Feb 17].
- ISO-13606-1. Health Informatics--Electronic Health Record Communication--Part 1: Reference Model. Switzerland: ISO; 2019. Available from https://www.iso.org/standard/67868. html [Last accessed on 2017 Feb 18].
- Beale T. Archetypes: Constraint-based domain models for future-proof information systems. In: Baclawski K, Kilov H, editors. Eleventh OOPSLA Workshop on Behavioral Semantics: Serving the Customer. Boston: Northeastern University; 2002.
- Ma C, Frankel H, Beale T, Heard S. EHR query language (EQL)--a query language for archetype-based health records. Stud Health Technol Inform. 2007;129(Pt 1):397-401.
- OpenEHR. Archetype Query Language (AQL). Available from: https://specifications.openehr.org/releases/query/ latest/aql.html [Last accessed on 2019 Feb 12].
- Chen R, Valladares C, Corbal I, Anani N, Koch S. Early experiences from a guideline-based computerized clinical decision support for stroke prevention in atrial fibrillation. Stud Health Technol Inform. 2013;192:244-247.
- Garde S. Clinical knowledge governance: The international perspective. Stud Health Technol Inform. 2013;193:269-281.
- Atalag K, Bilgen S. Multi-level Modeling and the Role of Archetypes in the Design of Health Information Systems: A Modeling Example in Endoscopy. In: Conference proceedings of the 2007 International Symposium on Health Informatics and Bioinformatics HIBIT07, Atalya, Turkey. doi: 10.13140/2.1.4762.2727
- Viceconti M, De Vos M, Mellone S, Geris L. Position paper from the digital twins in healthcare to the virtual human twin: A moon-shot project for digital health research. IEEE J Biomed Health Inform. 2023;28:491-501. doi: 10.1109/JBHI.2023.3323688
- Nickerson D, Atalag K, De Bono B, et al. The human physiome: How standards, software and innovative service infrastructures are providing the building blocks to make it achievable. Interface Focus. 2016;6(2):20150103. doi: 10.1098/rsfs.2015.0103
- Teno JM. Garbage in, Garbage out-words of caution on big data and machine learning in medical practice. JAMA Health Forum. 2023;4(2):e230397. doi: 10.1001/jamahealthforum.2023.0397
- Institute of Medicine Committee on Quality of Health Care in America. Crossing the Quality Chasm: A New Health System for the 21st Century. Washington, DC: National Academies Press.
- ISO/TR14639. Briefing Note: Strengthening National Health Systems through a Capacity-Based eHealth Architecture. Switzerland: International Organization of Standards ISO; 2014. Available from: https://www.iso.org/files/live/sites/ isoorg/files/archive/pdf/en/14639-brochureversionv7.pdf [Last accessed on 2020 Jul 18].
- PATH. Data Use partnerships: Theory of Change; 2016. Available from: https://www.path.org/our-impact/ resources/data-use-partnership-theory-of-change [Last accessed on 2021 Jul 22].
- Castro A, Machado J, Roggendorf M, Soller H. How to build a data architecture to Drive Innovation-Today and Tomorrow. McKinsey Technology; 2021. Available from: https://www.mckinsey.com/business-functions/mckinsey-digital/our-insights/how-to-build-a-data-architecture-to-drive-innovation-today-and-tomorrow [Last accessed on 2021 Apr 12].