Innovative infrared imaging approach for breast cancer screening: Integrating rotational thermography and machine learning analysis
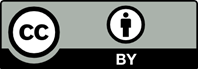
This paper presents a novel approach to breast cancer screening using infrared (IR) imaging. This work encompasses four phases: Refining data collection, advancing analysis methods, and enhancing feature extraction with machine learning. The developed system employed a temperature-controlled chamber with rotational thermography techniques to maintain consistent temperatures and capture high-quality IR images and all possible subject views. The paper describes four key experiments to detect breast cancer using IR imaging. The experiments involved the use of dynamic temperature-based data collection and a semi-circular arc movement to ensure precise imaging, keeping the object in focus. Initial experiments involved the use of dynamic temperature-based data collection and a semi-circular arc movement to ensure precise imaging focus. The final experiment incorporated a semi-circular arc movement. For each subject, 32 thermal IR images were acquired, targeting one breast at a time while isolating the other with an IR-proof barrier. The collected datasets were used for breast abnormality detection. The analyzed results revealed that support vector machine and neural network algorithms achieved an accuracy rate of 93.18%. The system’s installation at a hospital in India allowed for real-world application and validation. The final study, which introduced a new IR imaging protocol, demonstrated improved results compared to earlier pilot studies. This method enhances the accuracy of distinguishing malignant and benign tumors, supporting early breast cancer detection and treatment. The proposed methodology addresses data collection and analysis challenges, leading to improved screening efficiency and better patient outcomes.
- Sivanandam S, Anburajan M, Venkatraman B, Menaka M, Sharath D. Medical thermography: A diagnostic approach for type 2 diabetes based on non-contact infrared thermal imaging. Endocrine. 2012;42(2):343-351. doi: 10.1007/s12020-012-9645-8
- Nishide K, Nagase T, Oba M, et al. Ultrasonographic and thermographic screening for latent inflammation in diabetic foot callus. Diabetes Res Clin Pract. 2009;85(3):304-309. doi: 10.1016/j.diabres.2009.05.018
- Fujiwara Y, Inukai T, Aso Y, Takemura Y. Thermographic measurement of skin temperature recovery time of extremities in patients with type 2 diabetes mellitus. Exp Clin Endocrinol Diabetes. 2000;108(7):463-469. doi: 10.1055/s-2000-8142
- Fushimi H, Inoue T, Yamada Y, Matsuyama Y, Kubo M, Kameyama M. Abnormal vasoreaction of peripheral arteries to cold stimulus of both hands in diabetics. Diabetes Res Clin Pract. 1996;32(1-2):55-59. doi: 10.1016/0168-8227(96)01222-3
- Sodi A, Giambene B, Miranda P, Falaschi G, Corvi A, Menchini U. Ocular surface temperature in diabetic retinopathy: A pilot study by infrared thermography. Eur J Ophthalmol. 2009;19(6):1004-1008. doi: 10.1177/112067210901900617
- Ring EFJ, Ammer K. Infrared thermal imaging in medicine. Physiol Meas. 2012;33(3):R33-R46. doi: 10.1088/0967-3334/33/3/R33
- EtehadTavakol M, Sadri S, Ng EYK. Application of K- and Fuzzy c-means for color segmentation of thermal infrared breast images. J Med Syst. 2010;34(1):35-42. doi: 10.1007/s10916-008-9213-1
- Etehadtavakol M, Ng EYK. Color Segmentation of Breast Thermograms: A Comparative Study. Singapore: Springer; 2017. p. 69-77. doi: 10.1007/978-981-10-3147-2_6
- Pramanik S, Banik D, Bhattacharjee D, Nasipuri M, Bhowmik MK, Majumdar G. Suspicious-region segmentation from breast thermogram using DLPE-based level set method. IEEE Trans Med Imaging. 2019;38(2):572-584. doi: 10.1109/TMI.2018.2867620
- EtehadTavakol M, Chandran V, Ng EYK, Kafieh R. Breast cancer detection from thermal images using bispectral invariant features. Int J Therm Sci. 2013;69:21-36. doi: 10.1016/j.ijthermalsci.2013.03.001
- Garduño-Ramón MA, Vega-Mancilla SG, Morales- Henández LA, Osornio-Rios RA. Supportive noninvasive tool for the diagnosis of breast cancer using a thermographic camera as sensor. Sensors (Basel). 2017;17(3):497. doi: 10.3390/s17030497
- Prakash RM, Bhuvaneshwari K, Divya M, Sri KJ, Begum AS. Segmentation of Thermal Infrared Breast Images Using K-means, FCM and EM Algorithms for Breast Cancer Detection. In: 2017 International Conference on Innovations in Information, Embedded and Communication Systems (ICIIECS), IEEE; 2017. p. 1-4. doi: 10.1109/ICIIECS.2017.8276142
- Venkataramani K, Mestha LK, Ramachandra L, Prasad SS, Kumar V, Raja PJ. Semi-automated breast cancer tumor detection with thermographic video imaging. Annu Int Conf IEEE Eng Med Biol Soc. 2015;2015:2022-2025. doi: 10.1109/EMBC.2015.7318783
- Kandlikar SG, Perez-Raya I, Raghupathi PA, et al. Infrared imaging technology for breast cancer detection - Current status, protocols and new directions. Int J Heat Mass Transf. 2017;108:2303-2320. doi: 10.1016/j.ijheatmasstransfer.2017.01.086
- Bandyopadhyay A, Chaudhuri A, Mondal HS. IR based Intelligent Image Processing Techniques for Medical Applications. In: 2016 SAI Computing Conference (SAI), IEEE; 2016. p. 113-117. doi: 10.1109/SAI.2016.7555970
- Bandyopadhyay A, Mondal HS, Dam B, Patranabis DC. Efficient infrared image processing and machine learning algorithm for breast cancer screening. Comput Methods Biomech Biomed Eng Imaging Vis. 2023;11:2226-2238. doi: 10.1080/21681163.2023.2225639
- Bandyopadhyay A, Mondal HS, Pal B, Dam B, Patranabis DC. Exploring the Potential Use of Infrared Imaging in Medical Diagnosis: A Comprehensive Framework for Diabetes and Breast Cancer Screening. In: Proceedings of 4th International Conference on Image Processing and Capsule Networks (ICIPCN), Springer; 2023.
- Kapoor P, Prasad SVA. Image Processing for Early Diagnosis of Breast Cancer Using Infrared Images. In: 2010 the 2nd International Conference on Computer and Automation Engineering (ICCAE), IEEE; 2010. p. 564-566. doi: 10.1109/ICCAE.2010.5451827
- Gonzalez RC, Woods RE. Digital Image Processing. 4th ed. London: Pearson Education, Inc.; 2007.
- Chen GL, Lee CY. Iterative Morphology-based Segmentation of Breast Tumors in Ultrasound Images. In: 2014 International Symposium on Computer, Consumer and Control, IEEE; 2014. p. 1107-1110. doi: 10.1109/IS3C.2014.288
- Li C, Xu C, Gui C, Fox MD. Distance regularized level set evolution and its application to image segmentation. IEEE Trans Image Process. 2010;19(12):3243-3254. doi: 10.1109/TIP.2010.2069690
- Caselles V, Catté F, Coll T, Dibos F. A geometric model for active contours in image processing. Numer Math. 1993;66:1-3. doi: 10.1007/BF01385685
- Mambou SJ, Maresova P, Krejcar O, Selamat A, Kuca K. Breast cancer detection using infrared thermal imaging and a deep learning model. Sensors (Basel). 2018;18(9):2799. doi: 10.3390/s18092799
- Tsietso D, Yahya A, Samikannu R. A review on thermal imaging-based breast cancer detection using deep learning. Mob Inf Syst. 2022;2022:1-19. doi: 10.1155/2022/8952849
- Wu MN, Lin CC, Chang CC. Brain Tumor Detection Using Color-Based K-Means Clustering Segmentation. In: Third International Conference on Intelligent Information Hiding and Multimedia Signal Processing (IIH-MSP), IEEE; 2007. p. 245-250. doi: 10.1109/IIHMSP.2007.4457697
- Zhang Y, Wu X, He L, et al. Applications of hyperspectral imaging in the detection and diagnosis of solid tumours. Transl Cancer Res. 2020;9(2):1265-1277. doi: 10.21037/tcr.2019.12.53
- Lugano R, Ramachandran M, Dimberg A. Tumor angiogenesis: Causes, consequences, challenges and opportunities. Cell Mol Life Sci. 2020;77(9):1745-1770. doi: 10.1007/s00018-019-03351-7
- Houssein EH, Emam MM, Ali AA. An efficient multilevel thresholding segmentation method for thermography breast cancer imaging based on improved chimp optimization algorithm. Expert Syst Appl. 2021;185:115651. doi: 10.1016/j.eswa.2021.115651
- Houssein EH, Abdelkareem DA, Emam MM, Hameed MA, Younan M. An efficient image segmentation method for skin cancer imaging using improved golden jackal optimization algorithm. Comput Biol Med. 2022;149:106075. doi: 10.1016/j.compbiomed.2022.106075
- De Santana MA, Pereira JMS, da Silva FL, et al. Breast cancer diagnosis based on mammary thermography and extreme learning machines. Res Biomed Eng. 2018;34(1):45-53. doi: 10.1590/2446-4740.05217
- Gonçalves CB, Leles ACQ, Oliveira LE, Guimaraes G, Cunha JR, Fernandes H. Machine learning and infrared thermography for breast cancer detection. Proceedings. 2019;27:45. doi: 10.3390/proceedings2019027045
- Dixit S, Kumar A, Srinivasan K. A current review of machine learning and deep learning models in oral cancer diagnosis: Recent technologies, open challenges, and future research directions. Diagnostics (Basel). 2023;13(7):1353. doi: 10.3390/diagnostics13071353
- Cohen EE, Ahmed O, Kocherginsky M, et al. Study of functional infrared imaging for early detection of mucositis in locally advanced head and neck cancer treated with chemoradiotherapy. Oral Oncol. 2013;49(10):1025-1031. doi: 10.1016/j.oraloncology.2013.07.009
- Advanced Thermography and Preventive Education. Available from: https://thermogramcenter.com [Last accessed on 2023 Dec].
- Ng EYK. A review of thermography as promising non-invasive detection modality for breast tumor. Int J Therm Sci. 2009;48(5):849-859. doi: 10.1016/j.ijthermalsci.2008.06.015
- Wishart GC, Campisi M, Boswell M, et al. The accuracy of digital infrared imaging for breast cancer detection in women undergoing breast biopsy. Eur J Surg Oncol. 2010;36(6):535-540. doi: 10.1016/j.ejso.2010.04.003
- Kakileti ST, Madhu H, Subramoni T, Manjunath G. Thermalytix: Using AI to save lives. XRDS Crossroads ACM Mag Stud. 2020;26(3):38-41. doi: 10.1145/3383384