Accelerating hybrid lattice structures design with machine learning
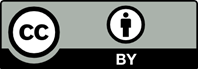
Lattice structures inspired by triply periodic minimal surfaces (TPMS) have attracted increasing attention due to their lightweight properties and high mechanical performance. Recent research showed that hybrid structures based on the topology of two or more types of TPMS can present interesting multifunctional properties. However, the complexity of TPMS-based lattice designs presents challenges in both design and evaluation. To address these challenges, this study was designed to explore the integration of the machine learning method to predict the mechanical properties of hybrid lattice structures inspired by TPMS based on their patterns. A back propagation neural network (BPNN) was designed and trained on a dataset generated through finite element (FE) simulations and homogenization methods. The BPNN demonstrated robustness in predicting elastic modulus and Poisson’s ratio of TPMS hybrid lattice structures, offering rapid and efficient predictions. Validation against FE simulations confirmed the accuracy and reliability of the BPNN predictions, proving its potential as a valuable tool for accelerating the design and evaluation of complex hybrid lattice structures.
Phuong Tran serves as the Editorial Board Member of the journal, but did not in any way involve in the editorial and peer-review process conducted for this paper, directly or indirectly.
- Ashby MF, Evans AG, Fleck NA, Gibson LJ, Hutchinson JW, Wadley HN. Metal Foams: A Design Guide. London: Butterworth-Heinemann; 2000.
- Peng C, Tran P, Nguyen-Xuan H, Ferreira AJ. Mechanical performance and fatigue life prediction of lattice structures: Parametric computational approach. Compos Struct. 2020;235:111821. doi: 10.1016/j.compstruct.2019.111821
- Wong M, Owen I, Sutcliffe CJ, Puri A. Convective heat transfer and pressure losses across novel heat sinks fabricated by Selective Laser Melting. Int J Heat Mass Tran. 2009;52(1- 2):281-288. doi: 10.1016/j.ijheatmasstransfer.2008.06.002
- Zhang XY, Fang G, Zhou J. Additively manufactured scaffolds for bone tissue engineering and the prediction of their mechanical behavior: A review. Materials (Basel). 2017;10(1)50. doi: 10.3390/ma10010050
- Tran P, Peng C. Triply periodic minimal surfaces sandwich structures subjected to shock impact. J Sandwich Struct Mater. 2020;23(6):2146-2175. doi: 10.1177/1099636220905551
- Peng C, Tran P. Bioinspired functionally graded gyroid sandwich panel subjected to impulsive loadings. Compos Part B Eng. 2020;188:107773. doi: 10.1016/j.compositesb.2020.107773
- Nguyen‐Van V, Peng C, Hazell PJ, Lee J, Nguyen‐Xuan H, Tran P. Performance of meta concrete panels subjected to explosive load: Numerical investigations. Struct Concr. 2023;25:1590-1619. doi: 10.1002/suco.202200749
- Catchpole-Smith S, Sélo RR, Davis AW, Ashcroft IA, Tuck CJ, Clare A. Thermal conductivity of TPMS lattice structures manufactured via laser powder bed fusion. Addit Manuf. 2019;30:100846. doi: 10.1016/j.addma.2019.100846
- Aremu AO, Brennan-Craddock JP, Panesar A, et al. A voxel-based method of constructing and skinning conformal and functionally graded lattice structures suitable for additive manufacturing. Addit Manuf. 2017;13:1-13. doi: 10.1016/j.addma.2016.10.006
- Peng C, Fox K, Qian M, Nguyen-Xuan H, Tran P. 3D printed sandwich beams with bioinspired cores: Mechanical performance and modelling. Thin Walled Struct. 2021;161:107471. doi: 10.1016/j.tws.2021.107471
- Wickramasinghe S, Peng C, Ladani R, Tran P. Analysing fracture properties of bio-inspired 3D printed suture structures. Thin Walled Struct. 2022;176:109317. doi: 10.1016/j.tws.2022.109317
- Wu C, Peng C, Le TC, Das R, Tran P. Tunable 3D printed composite metamaterials with negative stiffness. Smart Mater Struct. 2023;32(12):125010. doi: 10.1088/1361-665X/ad06df
- Ashby MF. The properties of foams and lattices. Philos Trans A Math Phys Eng Sci. 2006;364(1838):15-30. doi: 10.1098/rsta.2005.1678
- Rashed MG, Ashraf M, Mines RA, Hazell PJ. Metallic microlattice materials: A current state of the art on manufacturing, mechanical properties and applications. Mater Des. 2016;95:518-533. doi: 10.1016/j.matdes.2016.01.146
- Wang Y, Zhang L, Daynes S, Zhang H, Feih S, Wang MY. Design of graded lattice structure with optimized mesostructures for additive manufacturing. Mater Des. 2018;142:114-123. doi: 10.1016/j.matdes.2018.01.011
- Wang P, Yang F, Li P, Zheng B, Fan H. Design and additive manufacturing of a modified face-centered cubic lattice with enhanced energy absorption capability. Extreme Mech Lett. 2021;47:101358. doi: 10.1016/j.eml.2021.101358
- Daynes S, Feih S, Lu WF, Wei J. Optimisation of functionally graded lattice structures using isostatic lines. Mater Des. 2017;127:215-223. doi: 10.1016/j.matdes.2017.04.082
- Cao X, Zhang D, Liao B, et al. Numerical analysis of the mechanical behavior and energy absorption of a novel P-lattice. Thin Walled Struct. 2020;157:107147. doi: 10.1016/j.tws.2020.107147
- Lan T, Tran P. Multiscale topology optimization of lattice structure using 3D moving hollow morphable bars. JOM. 2021;73(12):4141-4153. doi: 10.1007/s11837-021-04917-2
- Al-Ketan O, Abu Al-Rub RK. Multifunctional mechanical metamaterials based on triply periodic minimal surface lattices. Adv Eng Mater. 2019;21(10):1900524. doi: 10.1002/adem.201900524
- Han L, Che S. An overview of materials with triply periodic minimal surfaces and related geometry: From biological structures to self-assembled systems. Adv Mater. 2018;30(17):e1705708. doi: 10.1002/adma.201705708
- Lan T, Peng C, Fox K, Do T, Tran P. Triply periodic minimal surfaces lattice structures: Functional graded and hybrid designs for engineering applications. Mater Sci Addit Manuf. 2023;2(3):1753. doi: 10.36922/msam.1753
- Maskery I, Aboulkhair NT, Aremu AO, Tuck CJ, Ashcroft IA. Compressive failure modes and energy absorption in additively manufactured double gyroid lattices. Addit Manuf. 2017;16:24-29. doi: 10.1016/j.addma.2017.04.003
- Li X, Xiao L, Song W. Compressive behavior of selective laser melting printed Gyroid structures under dynamic loading. Addit Manuf. 2021;46:102054. doi: 10.1016/j.addma.2021.102054
- Yan C, Hao L, Hussein A, Young P, Huang J, Zhu W. Microstructure and mechanical properties of aluminium alloy cellular lattice structures manufactured by direct metal laser sintering. Mater Sci Eng A. 2015;628:238-246. doi: 10.1016/j.msea.2015.01.063
- Cao X, Yang H, Ren X, et al. Mechanical performance and defect analysis of the imperfect micro smooth gyroid cylinder shell structure. Compos Struct. 2021;273:114320. doi: 10.1016/j.compstruct.2021.114320
- Peng C, Tran P, Mouritz AP. Compression and buckling analysis of 3D printed carbon fibre-reinforced polymer cellular composite structures. Compos Struct. 2022;300:116167. doi: 10.1016/j.compstruct.2022.116167
- Nguyen-Van V, Liu J, Peng C, Zhang G, Nguyen-Xuan H, Tran P. Dynamic responses of bioinspired plastic-reinforced cementitious beams. Cement Concr Compos. 2022;133:104682. doi: 10.1016/j.cemconcomp.2022.104682
- Nguyen-Van V, Tran P, Peng C, Pham L, Zhang G, Nguyen- Xuan H. Bioinspired cellular cementitious structures for prefabricated construction: Hybrid design and performance evaluations. Autom Construct. 2020;119:103324. doi: 10.1016/j.autcon.2020.103324
- Plocher J, Panesar A. Effect of density and unit cell size grading on the stiffness and energy absorption of short fibre-reinforced functionally graded lattice structures. Addit Manuf. 2020;33:101171. doi: 10.1016/j.addma.2020.101171
- Al-Ketan O, Lee DW, Abu Al-Rub RK. Mechanical properties of additively-manufactured sheet-based gyroidal stochastic cellular materials. Addit Manuf. 2021;48:102418. doi: 10.1016/j.addma.2021.102418
- Maskery I, Aremu AO, Parry L, Wildman RD, Tuck CJ, Ashcroft IA. Effective design and simulation of surface-based lattice structures featuring volume fraction and cell type grading. Mater Des. 2018;155:220-232. doi: 10.1016/j.matdes.2018.05.058
- Maskery I, Ashcroft IA. The deformation and elastic anisotropy of a new gyroid-based honeycomb made by laser sintering. Addit Manuf. 2020;36:101548. doi: 10.1016/j.addma.2020.101548
- Peng C, Marzocca P, Tran P. Triply periodic minimal surfaces based honeycomb structures with tuneable mechanical responses. Virtual Phys Prototyp. 2022;18(1):e2125879. doi: 10.1080/17452759.2022.2125879
- Cerniauskas G, Sadia H, Alam P. Machine intelligence in metamaterials design: A review. Oxford Open Mater Sci. 2024;4(1):itae001. doi: 10.1093/oxfmat/itae001
- Meng L, McWilliams B, Jarosinski W, et al. Machine learning in additive manufacturing: A review. JOM. 2020;72: 2363-2377. doi: 10.1007/s11837-020-04155-y
- Jiao P, Alavi AH. Artificial intelligence-enabled smart mechanical metamaterials: Advent and future trends. Int Mater Rev. 2021;66(6):365-393. doi: 10.1080/09506608.2020.1815394
- Bastek JH, Kochmann DM. Inverse design of nonlinear mechanical metamaterials via video denoising diffusion models. Nat Mach Intell. 2023;5(12):1466-1475. doi: 10.1038/s42256-023-00762-x
- Nadell CC, Huang B, Malof JM, Padilla WJ. Deep learning for accelerated all-dielectric metasurface design. Opt Express. 2019;27(20):27523-27535. doi: 10.1364/OE.27.027523
- Liu TW, Chan CT, Wu RT. Deep-learning-based acoustic metamaterial design for attenuating structure-borne noise in auditory frequency bands. Materials (Basel). 2023;16(5):1879. doi: 10.3390/ma16051879
- Li W, Chen P, Xiong B, et al. Deep learning modeling strategy for material science: From natural materials to metamaterials. J Phys Mater. 2022;5(1):014003. doi: 10.1088/2515-7639/ac5914
- Peng H, Liu A, Huang J, Cao L, Liu J, Lu L. PH-Net: Parallelepiped microstructure homogenization via 3D convolutional neural networks. Addit Manuf. 2022;60:103237. doi: 10.1016/j.addma.2022.103237
- Gu GX, Chen CT, Richmond DJ, Buehler MJ. Bioinspired hierarchical composite design using machine learning: Simulation, additive manufacturing, and experiment. Mater Horizons. 2018;5(5):939-945. doi: 10.1039/C8MH00653A
- Zheng X, Chen TT, Guo X, Samitsu S, Watanabe I. Controllable inverse design of auxetic metamaterials using deep learning. Mater Des. 2021;211:110178. doi: 10.1016/j.matdes.2021.110178
- Sui F, Guo R, Zhang Z, Gu GX, Lin L. Deep reinforcement learning for digital materials design. ACS Mater Lett. 2021;3(10):1433-1439. doi: 10.1021/acsmaterialslett.1c00390
- Wilt JK, Yang C, Gu GX. Accelerating auxetic metamaterial design with deep learning. Adv Eng Mater. 2020;22(5):1901266. doi: 10.1002/adem.201901266
- Do Carmo MP. Differential Geometry of Curves and Surfaces. 2nd ed. New York: Springer Cham; 2016.
- Schoen AH. NASA Technical Note D-5541; 1970.
- Matsen MW, Bates FS. Origins of complex self-assembly in block copolymers. Macromolecules. 1996;29(23):7641-7644. doi: 10.1021/ma960744q
- Pouya C, Overvelde JT, Kolle M, et al. Characterization of a mechanically tunable gyroid photonic crystal inspired by the butterfly parides sesostris. Adv Opt Mater. 2015;4(1): 99-105. doi: 10.1002/adom.201500436
- Michielsen K, Stavenga DG. Gyroid cuticular structures in butterfly wing scales: Biological photonic crystals. J R Soc Interface. 2008;5(18):85-94. doi: 10.1098/rsif.2007.1065
- Lai M, Kulak AN, Law D, Zhang Z, Meldrum FC, Riley DJ. Profiting from nature: Macroporous copper with superior mechanical properties. Chem Commun (Camb). 2007;34:3547-3549. doi: 10.1039/b707469g
- Brakke KA. The surface evolver. Exp Math. 1992;1(2): 141-165. doi: 10.1080/10586458.1992.10504253
- Dong G, Tang Y, Zhao YF. A 149 line homogenization code for three-dimensional cellular materials written in matlab. J Eng Mater Technol. 2019;141(1):11. doi: 10.1115/1.4040555
- Abadi M, Barham P, Chen J, et al. TensorFlow: A System for Large-Scale Machine Learning. In: 12th USENIX Symposium on Operating Systems Design and Implementation. 2016. p. 265-283.
- Yan H, Yu H, Zhu S, Wang Z, Zhang Y, Guo L. Nonlinear properties prediction and inverse design of a porous auxetic metamaterial based on neural networks. Thin Walled Struct. 2024;197:111717. doi: 10.1016/j.tws.2024.111717