Additive manufacturing: Application and validation of machine learning-based process-structure-property linkages in Ti-6Al-4V
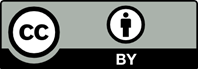
In the field of metal additive manufacturing (AM), various processes and heat treatments can yield unique grain morphologies, thereby influencing material properties and machining behavior. In this study, a novel workflow using a machine learning-based approach that combines statistical descriptors of textured AM-process induced microstructure, cutting force model (as a material response), and a data-mining method is established. It is proven to be a valid method for creating process-structure-property linkages for metal AM. This study focuses on two highly varied metal AM processes: Powder bed fusion (PBF, e.g., laser PBF and electron beam PBF) and directed energy deposition (DED, e.g., wire-fed plasma-directed energy deposition). The study also accounted for the effects of post-AM heat treatment and build orientation. It was found that the accuracy of material behavior predictions is highly correlated with AM processing conditions, building orientations, and machining conditions. Specifically, while initially applying PBF training data to DED samples resulted in a 15% root mean square prediction error, this error was subsequently reduced to <1% through cross-training using combined microstructure training data sets. This discrepancy could be attributed to the significantly different thermal cycling conditions in L-PBF and DED, which resulted in highly varied textured microstructures. Residual stresses generated during AM processing and the selection of machining parameters exert the highest impact on the machining behavior. The implications of these findings extend to the use of statistically descriptive microstructures for various AM processing conditions and build orientations in computational methods and other machining learning approaches.
- Conner JW, Manogharan BP, Martof GP, et al., 2014, Making sense of 3-D printing: Creating a map of additive manufacturing products and services. Addit Manuf, 1: 64–76. https://doi.org/10.1016/j.addma.2014.08.005
- Dutta B, (Sam) Froes FH, 2017, The additive manufacturing (AM) of titanium alloys. Metal Powder Rep, 72: 96–106. https://doi.org/10.1016/j.mprp.2016.12.062
- Arthur NK, 2019, Laser based manufacturing of ti6al4v: A comparison of lens and selective laser melting. Mater Sci Forum, 950: 44–49. https://doi.org/10.4028/www.scientific.net/MSF.950.44
- Frazier WE, 2014, Metal additive manufacturing: A review. J Mater Eng Perform, 23: 1917–1928. https://doi.org/10.1007/s11665-014-0958-z
- Baufeld B, Brandl E, Van Der Biest O, 2011, Wire based additive layer manufacturing: Comparison of microstructure and mechanical properties of Ti-6Al-4V components fabricated by laser-beam deposition and shaped metal deposition. J Mater Process Technol, 211: 1146–1158. https://doi.org/10.1016/j.jmatprotec.2011.01.018
- Kruth JP, Leu MC, Nakagawa T, 1998, Progress in additive manufacturing and rapid prototyping. Ann CIRP, 47: 525–540. https://doi.org/10.1016/S0007-8506(07)63240-5
- Gong X, Zeng D, Groeneveld-Meijer W, et al., 2022, Additive manufacturing: A machine learning model of process-structure-property linkages for machining behavior of Ti-6Al-4V. Mater Sci Addit Manuf, 1: 6. https://doi.org/10.18063/msam.v1i1.6
- Liu Q, Wu H, Paul MJ, et al., 2020, Machine-learning assisted laser powder bed fusion process optimization for AlSi10Mg: New microstructure description indices and fracture mechanisms. Acta Mater, 201: 316–328. https://doi.org/10.1016/J.ACTAMAT.2020.10.010
- Wang C, Chandra S, Huang S, et al., 2023, Unraveling process-microstructure-property correlations in powder-bed fusion additive manufacturing through information-rich surface features with deep learning. J Mater Process Technol, 311: 117804. https://doi.org/10.1016/J.JMATPROTEC.2022.117804
- Goh GD, Huang X, Huang S, et al., 2023, Data imputation strategies for process optimization of laser powder bed fusion of Ti6Al4V using machine learning. Mater Sci Addit Manuf, 2: 50. https://doi.org/10.36922/msam.50
- Mondal B, Mukherjee T, DebRoy T, 2022, Crack free metal printing using physics informed machine learning. Acta Mater, 226: 117612. https://doi.org/10.1016/J.ACTAMAT.2021.117612
- Galati M, Iuliano L, 2018, A literature review of powder-based electron beam melting focusing on numerical simulations. Addit Manuf, 19: 1–20. https://doi.org/10.1016/J.ADDMA.2017.11.001
- Biamino S, Penna A, Ackelid U, et al., 2011, Electron beam melting of Ti-48Al-2Cr-2Nb alloy: Microstructure and mechanical properties investigation. Intermetallics (Barking), 19: 776–781. https://doi.org/10.1016/J.INTERMET.2010.11.017
- Vayre B, Vignat F, Villeneuve F, 2012, Metallic additive manufacturing: State-of-the-art review and prospects. Mech Ind, 13: 89–96. https://doi.org/10.1051/MECA/2012003
- Bhavar V, Kattire P, Patil V, et al., 2018, A review on powder bed fusion technology of metal additive manufacturing. In: Additive Manufacturing Handbook. United States: CRC Press. p. 251–253. https://doi.org/10.1201/9781315119106-15
- Saboori A, Gallo D, Biamino S, et al., 2017, An overview of additive manufacturing of titanium components by directed energy deposition: Microstructure and mechanical properties. Appl Sci 7: 883. https://doi.org/10.3390/APP7090883
- Bordin A, Sartori S, Bruschi S, et al., 2017, Experimental investigation on the feasibility of dry and cryogenic machining as sustainable strategies when turning Ti6Al4V produced by Additive Manufacturing. J Clean Prod, 142: 4142–4151. https://doi.org/10.1016/J.JCLEPRO.2016.09.209
- Ding R, Guo ZX, Wilson A, 2002, Microstructural evolution of a Ti-6Al-4V alloy during thermomechanical processing. Mater Sci Eng A, 327: 233–245. https://doi.org/10.1016/S0921-5093(01)01531-3
- Baufeld B, Van der Biest O, Gault R, 2010, Additive manufacturing of Ti–6Al–4V components by shaped metal deposition: Microstructure and mechanical properties. Mater Des, 31: S106–S111. https://doi.org/10.1016/J.MATDES.2009.11.032
- Anwar S, Ahmed N, Abdo BM, et al., 2018, Electron beam melting of gamma titanium aluminide and investigating the effect of EBM layer orientation on milling performance. Int J Adv Manuf Technol, 96: 3093–3107. https://doi.org/10.1007/s00170-018-1802-7
- Vayre B, Vignat F, Villeneuve F, 2013, Identification on some design key parameters for additive manufacturing: Application on electron beam melting. Proc CIRP, 7: 264–269. https://doi.org/10.1016/j.procir.2013.05.045
- Murr LE, Johnson WL, 2017, 3D metal droplet printing development and advanced materials additive manufacturing. J Mat Res Technol, 6: 77–89. https://doi.org/10.1016/j.jmrt.2016.11.002
- Antonysamy AA, Meyer J, Prangnell PB, 2013, Effect of build geometry on the β-grain structure and texture in additive manufacture of Ti6Al4V by selective electron beam melting. Mater Charact, 84: 153–168. https://doi.org/10.1016/J.MATCHAR.2013.07.012
- Al-Bermani SS, Blackmore ML, Zhang W, et al., 2010, The origin of microstructural diversity, texture, and mechanical properties in electron beam melted Ti-6Al-4V. Metall Mater Trans A Phys Metall Mater Sci, 41: 3422–3434. https://doi.org/10.1007/s11661-010-0397-x
- Murr LE, Gaytan SM, Medina F, et al., 2010, Characterization of Ti-6Al-4V open cellular foams fabricated by additive manufacturing using electron beam melting. Mater Sci Eng A, 527: 1861–1868. https://doi.org/10.1016/j.msea.2009.11.015
- Cheng B, Price S, Lydon J, et al., 2014, On process temperature in powder-bed electron beam additive manufacturing: Model development and validation. J Manuf Sci Eng Trans ASME, 136: 061019. https://doi.org/10.1115/1.4028484
- King WE, Anderson AT, Ferencz RM, et al., 2015, Laser powder bed fusion additive manufacturing of metals; physics, computational, and materials challenges. Appl Phys Rev, 2: 041304. https://doi.org/10.1063/1.4937809
- Loh LE, Chua CK, Yeong WY, et al., 2015, Numerical investigation and an effective modelling on the selective laser melting (SLM) process with aluminium alloy 6061. Int J Heat Mass Transf, 80: 288–300. https://doi.org/10.1016/J.IJHEATMASSTRANSFER.2014.09.014
- Vilardell AM, Fredriksson G, Yadroitsev I, et al., 2019, Fracture mechanisms in the as-built and stress-relieved laser powder bed fusion Ti6Al4V ELI alloy. Opt Laser Technol, 109: 608–615. https://doi.org/10.1016/j.optlastec.2018.08.042
- Heigel JC, Phan TQ, Fox JC, et al., 2018, Experimental investigation of residual stress and its impact on machining in hybrid additive/subtractive manufacturing. Proc Manuf, 26: 929–940. https://doi.org/10.1016/J.PROMFG.2018.07.120
- Ming W, Chen J, An Q, et al., 2019, Dynamic mechanical properties and machinability characteristics of selective laser melted and forged Ti6Al4V. J Mater Process Technol, 271: 284–292. https://doi.org/10.1016/j.jmatprotec.2019.04.015
- Vrancken B, Thijs L, Kruth JP, et al., 2012, Heat treatment of Ti6Al4V produced by selective laser melting: Microstructure and mechanical properties. J Alloys Compd, 541: 177–185. https://doi.org/10.1016/j.jallcom.2012.07.022
- Ren S, Chen Y, Liu T, et al., 2019, Effect of build orientation on mechanical properties and microstructure of Ti-6Al-4V manufactured by selective laser melting. Metall Mater Trans A Phys Metall Mater Sci, 50: 4388–4409. https://doi.org/10.1007/s11661-019-05322-w
- Thijs L, Vrancken B, Kruth JP, et al., 2013, The Influence of Process Parameters and Scanning Strategy on the Texture in Ti6Al4V Part Produced by Selective Laser Melting. In: Proceedings of the Advanced Materials, Processes and Applications for Additive Manufacturing, Vol. 1. p. 21–28.
- Wanjara P, Brochu M, Jahazi M, 2007, Electron beam freeforming of stainless steel using solid wire feed. Mater Des, 28: 2278–2286. https://doi.org/10.1016/j.matdes.2006.08.008
- Taminger K, Hafley R, 2003, Electron Beam Freeform Fabrication: A Rapid Metal Deposition Process. Proceedings of the 3rd Annual Automotive Composites Conference, 3, p. 9–10. Available from: https://ntrs.nasa.gov/ citations/20040042496 [Last accessed on 2023 Aug 25].
- Hofmann DC, Roberts S, Otis R, et al., 2014, Developing gradient metal alloys through radial deposition additive manufacturing. Sci Rep, 4: 5357. https://doi.org/10.1038/srep05357
- Sun G, Zhou R, Lu J, et al., 2015, Mazumder, ‘Evaluation of defect density, microstructure, residual stress, elastic modulus, hardness and strength of laser-deposited AISI 4340 steel. Acta Mater, 84: 172–189. https://doi.org/10.1016/j.actamat.2014.09.028
- Zhang YN, Cao X, Wanjara P, et al., 2015, Tensile properties of laser additive manufactured Inconel 718 using filler wire. J Mater Res, 30: 2006–2020. https://doi.org/10.1557/jmr.2014.199
- Derekar KS, 2018, A review of wire arc additive manufacturing and advances in wire arc additive manufacturing of aluminium. Mater Sci Technol, 34: 895–916. https://doi.org/10.1080/02670836.2018.1455012
- McAndrew AR, Rosales MA, Colegrove PA, et al., 2018, Interpass rolling of Ti-6Al-4V wire + arc additively manufactured features for microstructural refinement. Addit Manuf, 21: 340–349. https://doi.org/10.1016/j.addma.2018.03.006
- Guzanová A, Ižaríková G, Brezinová J, et al., 2017, Influence of build orientation, heat treatment, and laser power on the hardness of Ti6Al4V manufactured using the DMLS process. Metals (Basel), 7: 318. https://doi.org/10.3390/met7080318
- Gorsse S, Hutchinson C, Gouné M, et al., 2017, Additive manufacturing of metals: a brief review of the characteristic microstructures and properties of steels, Ti-6Al-4V and high-entropy alloys. Sci Technol Adv Mater, 18: 584–610. https://doi.org/10.1080/14686996.2017.1361305
- Neikter M, Åkerfeldt P, Pederson R, et al., 2018, Microstructural characterization and comparison of Ti-6Al-4V manufactured with different additive manufacturing processes. Mater Charact, 143: 68–75. https://doi.org/10.1016/j.matchar.2018.02.003
- Li GC, Li J, Tian XJ, et al., 2017, Microstructure and properties of a novel titanium alloy Ti-6Al-2V-1.5Mo- 0.5Zr-0.3Si manufactured by laser additive manufacturing. Mater Sci Eng A, 684: 233–238. https://doi.org/10.1016/j.msea.2016.11.084
- Lin JJ, Lv YH, Liu YX, 2016, Microstructural evolution and mechanical properties of Ti-6Al-4V wall deposited by pulsed plasma arc additive manufacturing. Mater Des, 102: 30–40. https://doi.org/10.1016/j.matdes.2016.04.018
- Zhai Y, Galarraga H, Lados DA, 2016, Microstructure, static properties, and fatigue crack growth mechanisms in Ti-6Al-4V fabricated by additive manufacturing: LENS and EBM. Eng Fail Anal, 69: 3–14. https://doi.org/10.1016/j.engfailanal.2016.05.036
- Liu S, Shin YC, 2019, Additive manufacturing of Ti6Al4V alloy : A review. Mater Des, 164: 107552. https://doi.org/10.1016/j.matdes.2018.107552
- Bonaiti G, Parenti P, Annoni M, et al., 2017, Micro-milling machinability of DED additive titanium Ti-6Al-4V. Proc Manuf, 10: 497–509. https://doi.org/10.1016/j.promfg.2017.07.104
- Oyelola O, Crawforth P, M’Saoubi R, et al., 2018, Machining of functionally graded Ti6Al4V/WC produced by directed energy deposition. Addit Manuf, 24: 20–29. https://doi.org/10.1016/j.addma.2018.09.007
- Acharya R, Sharon JA, Staroselsky A, 2017, Prediction of microstructure in laser powder bed fusion process. Acta Mater, 124: 360–371. https://doi.org/10.1016/j.actamat.2016.11.018
- Fergani O, Berto F, Welo T, et al., 2017, Analytical modelling of residual stress in additive manufacturing. Fatigue Fract Eng Mater Struct, 40: 971–978. https://doi.org/10.1111/ffe.12560
- Chen Q, Guillemot G, Gandin CA, et al., 2017, Three-dimensional finite element thermomechanical modeling of additive manufacturing by selective laser melting for ceramic materials. Addit Manuf, 16: 124–137. https://doi.org/10.1016/j.addma.2017.02.005
- Bostanabad R, Zhang Y, Li X, et al., 2018, Computational microstructure characterization and reconstruction: Review of the state-of-the-art techniques. Prog Mater Sci, 95: 1–41. https://doi.org/10.1016/j.pmatsci.2018.01.005
- Popova E, Rodgers TM, Gong X, et al., 2017, Process-structure linkages using a data science approach: Application to simulated additive manufacturing data. Integr Mater Manuf Innov, 6: 54–68. https://doi.org/10.1007/s40192-017-0088-1
- Pilania G, Wang C, Jiang X, et al., 2013, Accelerating materials property predictions using machine learning. Sci Rep, 3: 1–6. https://doi.org/10.1038/srep02810
- Ko H, Witherell P, Ndiaye NY, et al., 2019, Machine learning based continuous knowledge engineering for additive manufacturing. In: IEEE International Conference on Automation Science and Engineering, p. 648–654. https://doi.org/10.1109/COASE.2019.8843316
- Baumann FW, Sekulla A, Hassler M, et al., 2018, Trends of machine learning in additive manufacturing. Int J Rapid Manuf, 7: 310. https://doi.org/10.1504/IJRAPIDM.2018.095788
- Delli U, Chang S, 2018, Automated process monitoring in 3D printing using supervised machine learning. Proc Manuf, 26: 865–870. https://doi.org/10.1016/j.promfg.2018.07.111
- Yao X, Moon SK, Bi G, 2017, A hybrid machine learning approach for additive manufacturing design feature recommendation. Rapid Prototype J, 23: 983–997.
- ASME, 2019, ASME Y14.41-Digital Product Definition Data Practices: ASME Digital Standards. New York City: ASME, p. 128. Available: https://www.asme.org/codes-standards/ find-codes-standards/y14-5-dimensioning-tolerancing [Last accessed on 2023 Sep 21].
- Tilton M, Borjali A, Isaacson A, et al., 2021, On structure and mechanics of biomimetic meta-biomaterials fabricated via metal additive manufacturing. Mater Des, 201: 109498. https://doi.org/10.1016/J.MATDES.2021.109498
- Wang SQ, Liu JH, Chen DL, 2014, Effect of strain rate and temperature on strain hardening behavior of a dissimilar joint between Ti-6Al-4V and Ti17 alloys. Mater Des, 56: 174–184. https://doi.org/10.1016/j.matdes.2013.11.003
- Rao PP, Tangri K, 1991, Yielding and work hardening behaviour of titanium aluminides at different temperatures. Mater Sci Eng A, 132: 49–59. https://doi.org/10.1016/0921-5093(91)90360-Y
- Bystrzanowski S, Bartels A, Clemens H, et al., 2008, Characteristics of the tensile flow behavior of Ti-46Al-9Nb sheet material-analysis of thermally activated processes of plastic deformation. Intermetallics (Barking), 16: 717–726. https://doi.org/10.1016/j.intermet.2008.02.008
- Lu W, Li X, Lei Y, et al., 2012, Study on the mechanical heterogeneity of electron beam welded thick TC4-DT joints. Mater Sci Eng A, 540: 135–141. https://doi.org/10.1016/j.msea.2012.01.117
- Chiou ST, Tsai HL, Lee WS, 2007, Effects of strain rate and temperature on the deformation and fracture behaviour of titanium alloy. Mater Trans, 48: 2525–2533. https://doi.org/10.2320/MATERTRANS.MRA2007607
- E. Committee of C, 2001, Aeronautical Materials, ‘中国航空材料手册 (China Aeronautical Materials Handbook). 2nd ed. Beijing: Standing Press China, p. 874.
- Rafi HK, Karthik NV, Gong H, et al., 2013, Microstructures and mechanical properties of Ti6Al4V parts fabricated by selective laser melting and electron beam melting. J Mater Eng Perform, 22: 3872–3883. https://doi.org/10.1007/S11665-013-0658-0/FIGURES/14
- Liu Q, Wang Y, Zheng H, et al., 2016, Microstructure and mechanical properties of LMD-SLM hybrid forming Ti6Al4V alloy. Mater Sci Eng A, 660: 24–33. https://doi.org/10.1016/j.msea.2016.02.069
- Shi X, Ma S, Liu C, et al., 2017, Selective laser melting-wire arc additive manufacturing hybrid fabrication of Ti-6Al-4V alloy: Microstructure and mechanical properties. Mater Sci Eng A, 684: 196–204. https://doi.org/10.1016/j.msea.2016.12.065
- Simonelli M, Tse YY, Tuck C, 2014, Effect of the build orientation on the mechanical properties and fracture modes of SLM Ti-6Al-4V. Mater Sci Eng A, 616: 1–11. https://doi.org/10.1016/j.msea.2014.07.086
- Tan X, Kok Y, Tan YJ, et al., 2015, Graded microstructure and mechanical properties of additive manufactured Ti-6Al-4V via electron beam melting. Acta Mater, 97: 1–16. https://doi.org/10.1016/j.actamat.2015.06.036
- Murr LE, Esquivel EV, Quinones SA, et al., 2009, Microstructures and mechanical properties of electron beam-rapid manufactured Ti-6Al-4V biomedical prototypes compared to wrought Ti-6Al-4V. Mater Charact, 60: 96–105. https://doi.org/10.1016/j.matchar.2008.07.006
- Wolff S, Lee T, Faierson E, et al., 2016, Anisotropic properties of directed energy deposition (DED)-processed Ti-6Al-4V. J Manuf Process, 24: 397–405. https://doi.org/10.1016/j.jmapro.2016.06.020
- Qiu C, Adkins NJ, Attallah MM, 2013, Microstructure and tensile properties of selectively laser-melted and of HIPed laser-melted Ti-6Al-4V. Mater Sci Eng A, 578: 230–239. https://doi.org/10.1016/j.msea.2013.04.099
- Bordin A, Bruschi S, Ghiotti A, et al., 2015, Analysis of tool wear in cryogenic machining of additive manufactured Ti6Al4V alloy. Wear, 328–329: 89–99. https://doi.org/10.1016/j.wear.2015.01.030
- Shunmugavel M, Polishetty A, Goldberg M, et al., 2017, A comparative study of mechanical properties and machinability of wrought and additive manufactured (selective laser melting) titanium alloy-Ti-6Al-4V. Rapid Prototype J, 23: 1051–1056. https://doi.org/10.1108/RPJ-08-2015-0105
- Polishetty A, Shunmugavel M, Goldberg M, et al., 2017, Cutting force and surface finish analysis of machining additive manufactured titanium alloy Ti-6Al-4V. Proc Manuf, 7: 284–289. https://doi.org/10.1016/j.promfg.2016.12.071
- Wu X, Jiang P, Chen L, et al., 2014, Extraordinary strain hardening by gradient structure. Proc Natl Acad Sci U S A, 111: 7197–7201. https://doi.org/10.1073/pnas.1324069111
- Jiao Y, Stillinger FH, Torquato S, 2007, Modeling heterogeneous materials via two-point correlation functions: Basic principles. Phys Rev E Stat Nonlin Soft Matter Phys, 76: 1–15. https://doi.org/10.1103/PhysRevE.76.031110
- Berryman JG, Blair SC, 1986, Use of digital image analysis to estimate fluid permeability of porous materials: Application of two-point correlation functions. J Appl Phys, 60: 1930–1938. https://doi.org/10.1063/1.337245
- Corson PB, 1974, Correlation functions for predicting properties of heterogeneous materials. I. Experimental measurement of spatial correlation functions in multiphase solids. J Appl Phys, 45: 3159–3164. https://doi.org/10.1063/1.1663741
- Turner DM, Niezgoda SR, Kalidindi SR, 2016, Efficient computation of the angularly resolved chord length distributions and lineal path functions in large microstructure datasets. Model Simul Mat Sci Eng, 24: 075002. https://doi.org/10.1088/0965-0393/24/7/075002
- Fitzpatrick ME, Fry AT, Holdway P, et al., 2005, Determination of residual stresses by X-ray diffraction-issue 2. In: A National Measurement Good Practice Guide. United Kingdom: National Physical Laboratory. https://doi.org/10.1063/1.3525214
- Luo Q, Yang S, 2017, Uncertainty of the X-ray diffraction (XRD) sin2 Ψ technique in measuring residual stresses of physical vapor deposition (PVD) hard coatings. Coatings, 7: 128. https://doi.org/10.3390/coatings7080128
- Priddy MW, Paulson NH, Kalidindi SR, et al., 2017, Strategies for rapid parametric assessment of microstructure-sensitive fatigue for HCP polycrystals. Int J Fatigue, 104: 231–242. https://doi.org/10.1016/j.ijfatigue.2017.07.015
- Bieler TR, Semiatin SL, 2002, The origins of heterogeneous deformation during primary hot working of Ti-6Al-4V. Int J Plast, 18: 1165–1189. https://doi.org/10.1016/S0749-6419(01)00057-2
- Hémery S, Villechaise P, 2018, Investigation of size effects in slip strength of titanium alloys: α Nodule size dependence of the critical resolved shear stress. Metall Mater Trans A Phys Metall Mater Sci, 49: 4394–4397. https://doi.org/10.1007/s11661-018-4678-0
- Gong G, 1986, Cross-validation, the jack knife, and the bootstrap: Excess error estimation in forward logistic regression. J Am Stat Assoc, 81: 108–113. https://doi.org/10.1080/01621459.1986.10478245
- Chen T, Guestrin C, 2016, XGBoost: A Scalable Tree Boosting System. KDD ’16, August 13-17, San Francisco, CA, USA, 2016.
- Sundararaghavan V, Zabaras N, 2005, Classification and reconstruction of three-dimensional microstructures using support vector machines. Comput Mater Sci, 32: 223–239. https://doi.org/10.1016/j.commatsci.2004.07.004
- Sun J, Guo YB, 2009, Material flow stress and failure in multiscale machining titanium alloy Ti-6Al-4V. Int J Adv Manuf Technol, 41: 651–659. https://doi.org/10.1007/s00170-008-1521-6
- Ginting A, Nouari M, 2009, Surface integrity of dry machined titanium alloys. Int J Mach Tools Manuf, 49: 325– 332. https://doi.org/10.1016/j.ijmachtools.2008.10.011
- Pramanik A, 2014, Problems and solutions in machining of titanium alloys. Int J Adv Manuf Technol, 70: 919–928. https://doi.org/10.1007/s00170-013-5326-x