Assessment and optimization of thermal and fluidity properties of high strength concrete via genetic algorithm
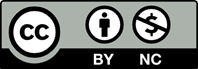
, This paper proposes a Response Surface Methodology (RSM) based Genetic Algorithm (GA) using MATLAB® to assess and optimize the thermal and fluidity of high strength concrete (HSC). The overall heat transfer coefficient, slump-spread flow and T50 time was defined as thermal and fluidity properties of high strength concrete. In addition to above mentioned properties, a 28-day compressive strength of HSC was also determined. Water to binder ratio, fine aggregate to total aggregate ratio and the percentage of super-plasticizer content was determined as effective factors on thermal and fluidity properties of HSC. GA based multi-objective optimization method was carried out by obtaining quadratic models using RSM. Having excessive or low ratio of water to binder provides lower overall heat transfer coefficient. Moreover, T50 time of high strength concrete decreased with the increasing of water to binder ratio and the percentage of superplasticizer content. Results show that RSM based GA is effective in determining optimal mixture ratios of HSC.
[1] Yan, S., Lin, H.-C. and Y.-C. Liu, Optimal schedule adjustments for supplying ready mixed concrete following incidents. Automation in Construction, 20 (8), 1041-1050 (2011).
[2] Chang, C.Y., Huang, R., Lee, P. C. and Weng, T.L., Application of a weighted Grey-Taguchi method for optimizing recycled aggregate concrete mixtures. Cement and Concrete Composites, 33(10), 1038- 1049 (2011)
[3] Şimşek, B., İç, Y.T. and Şimşek, E.H., A Full Factorial Design Based Desirability Function Approach for Optimization of Properties of C 40/50 Concrete Class. Mathematical and Computational Applications, 18(3), 330 (2013).
[4] Dvorkin, L., Dvorkin, O., Zhitkovsky, V. and Ribakov, Y., A method for optimal design of steel fiber reinforced concrete composition. Materials & Design, 32(6), 3254-3262 (2011).
[5] Lovato, P.S., Possan, E., Molin, D.C.C.D., Masuero, A.B. and Ribeiro, J.l.D., Modeling of mechanical properties and durability of recycled aggregate concretes. Construction and Building Materials, 26(1), 437-447 (2012).
[6] Mohammed, B.S., Fang, O.C., Anwar Hossain, K.M. and Lachemi, M., Mix proportioning of concrete containing paper mill residuals using response surface methodology. Construction and Building Materials, 35, 63-68 (2012).
[7] Olivia, M. and Nikraz, H., Properties of fly ash geopolymer concrete designed by Taguchi method. Materials & Design, 36, 191-198 (2012).
[8] Şimşek, B., İç,Y.T. and Şimşek, E.H., A TOPSISbased Taguchi optimization to determine optimal mixture proportions of the high strength selfcompacting concrete. Chemometrics and Intelligent Laboratory Systems, 125, 18-32 (2013).
[9] Sharma, N., Khanna, R. and Gupta, R.D., WEDM process variables investigation for HSLA by response surface methodology and genetic algorithm. Engineering Science and Technology, an International Journal, 18(2), 171-177 (2015).
[10] Subramanian, M., Sakthivel, M., Sooryaprakash, K., Sudhakaran, R., Optimization of Cutting Parameters for Cutting Force in Shoulder Milling of Al7075-T6 Using Response Surface Methodology and Genetic Algorithm. Procedia Engineering, 64, 690-700, 2013.
[11] Fayyazi, E., Ghobadian, B., Najafi, G., Hosseinzadeh, B., Mamat, R. and Hosseinzadeh, J., An ultrasound-assisted system for the optimization of biodiesel production from chicken fat oil using a genetic algorithm and response surface methodology. Ultrasonics Sonochemistry, 26, 312- 320 (2015).
[12] Kumar, A., Pathak, A.K. and Guria, C., NPK- 10:26:26 complex fertilizer assisted optimal cultivation of Dunaliella tertiolecta using response surface methodology and genetic algorithm. Bioresource Technology, 194, 117-129 (2015).
[13] Baş, D. and Boyacı, İ.H., Modeling and optimization II: Comparison of estimation capabilities of response surface methodology with artificial neural networks in a biochemical reaction. Journal of Food Engineering, 78(3), 846-854 (2007).
[14] Baş, D. and Boyacı, İ.H., Modeling and optimization I: Usability of response surface methodology. Journal of Food Engineering, 78(3), 836-845(2007).
[15] Lin, H.C., Su, C.T., Wang, C.C., Chang, B. H. and Juang, R.C., Parameter optimization of continuous sputtering process based on Taguchi methods, neural networks, desirability function, and genetic algorithms. Expert Systems with Applications, 39 (17), 12918-12925 (2012).
[16] Şimşek, B., İç, Y.T., Şimşek, E.H., Güvenç, A.B., Development of a graphical user interface for determining the optimal mixture parameters of normal weight concretes: A response surface methodology based quadratic programming approach. Chemometrics and Intelligent Laboratory Systems, 136, 1-9 (2014).
[17] Şimşek, B., İç, Y.T. and Şimşek, E.H. A RSMBased Multi-Response Optimization Application for Determining Optimal Mix Proportions of Standard Ready-Mixed Concrete. Arabian Journal for Science and Engineering, 41(4), 1435-1450 (2016).
[18] Basri, M., Rahman, R.N.Z.R.A., Ebrahimpour, A., Salleh, A.B., Gunawan, E.R. and Rahman, M.B.A., Comparison of estimation capabilities of response surface methodology (RSM) with artificial neural network (ANN) in lipase-catalyzed synthesis of palm-based wax ester. BMC Biotechnology, 7(1), 1- 14 (2007).
[19] Ortiz Jr, F., Simpson, J. R., Pignatiello, Jr, J.J., Heredia-Langner, A., A genetic algorithm approach to multiple-response optimization. Journal of Quality Technology, 36(4), 432 (2004).
[20] Chandwani, V., Agrawal, V. and Nagar, R., Modeling slump of ready mix concrete using genetic algorithms assisted training of Artificial Neural Networks. Expert Systems with Applications, 42(2), 885-893 (2015)
[21] Dombaycı, Ö.A., The prediction of heating energy consumption in a model house by using artificial neural networks in Denizli–Turkey. Advances in Engineering Software, 41(2), 141-147 (2010).
[22] Foucquier, A., Robert, S., Suard, F., Stephan, L. and Jay, A., State of the art in building modelling and energy performances prediction: A review. Renewable and Sustainable Energy Reviews, 23, 272-288 (2013).
[23] Lakatos, Á., Investigation Of The Effect Of Moisture In The Time Lag Of Building Walls With Different Insulating Materials. Environmental Engineering And Management Journal, 13(11), 2853-2858 (2014).
[24] Lee, Y., Choi, M.S., Yi, S.T. and Kim, J.K., Experimental study on the convective heat transfer coefficient of early-age concrete. Cement and Concrete Composites, 31(1), 60-71 (2009).
[25] Lennon, T., Designers' Guide to EN 1991-1-2, EN 1993-1-2 and EN 1994-1-2: Handbook for the Fire Design of Steel, Composite and Concrete Structures to the Eurocodes. Thomas Telford (2007).
[26] Simsek, B., Simsek, E.H. and Altunok, T. Empirical and Statistical Modeling of Heat Loss from Surface of a Cement Rotary Kiln System. Journal of the Faculty of Engineering and Architecture of Gazi University, 28(1), 59-66 (2013)