Exploring constraint qualification-free optimality conditions for linear second-order cone programming
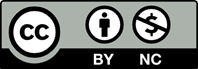
Linear second-order cone programming (SOCP) deals with optimization problems characterized by a linear objective function and a feasible region defined by linear equalities and second-order cone constraints. These constraints involve the norm of a linear combination of variables, enabling the representation of a wide range of convex sets. The SOCP serves as a potent tool for addressing optimization challenges across engineering, finance, machine learning, and various other domains. In this paper, we introduce new optimality conditions tailored for {SOCP} problems. These conditions have the form of two optimality criteria that are obtained without the requirement of any constraint qualifications and are defined explicitly. The first criterion utilizes the concept of immobile indices of constraints. The second criterion, without relying explicitly on immobile indices, introduces a special finite vector set for assessing optimality. To demonstrate the effectiveness of these criteria, we present two illustrative examples highlighting their applicability. We compare the obtained criteria with other known optimality conditions and show the advantage of the former ones.
[1] Nesterov, Y., & Nemirovski, A.S. (1992). Conic formulation of a convex programming problem and duality. Optimization Methods & Software, 1(2), 95-115. https://doi.org/10.1080/1055 6789208805510
[2] Glineur, F. (2001). Conic optimization: an elegant framework for convex optimization. Belgian Journal of Operations Research, Statistics and Computer Science, 41(1-2), 5-28.
[3] D¨ur, M. & Rendl, F. (2021). Conic optimization: A survey with special focus on copositive optimization and binary quadratic problems. EURO Journal on Computational Optimization, v.9, 100021. https://doi.org/10.1016/j.ejco .2021.100021
[4] Hanasusanto, G. A., & Kuhn, D. (2018). Conic programming reformulations of two stage distributionally robust linear programs over Wasserstein balls. Operations Research, 66(3), 849-869. https://doi.org/10.1287/opre.2 017.1698
[5] Kocuk, B. (2021) Conic reformulations for Kullback-Leibler divergence constrained distributionally robust optimization and applications. An International Journal of Optimization and Control: Theories and Applications, 11(2), pp.139-151. h t t p s : //doi.org/10.11121/ijocta.01.2021.001001
[6] Pataki, G. (2000). The geometry of semidefinite programming. In: H. Wolkowicz, R. Saigal, & L. Vandenberghe (eds.) Handbook of Semidefinite Programming. International Series in Operations Research & Management Science, vol 27. Springer, Boston, MA, 29-65. https://doi.or g/10.1007/978-1-4615-4381-7_3
[7] Vandenberghe, L. & Boyd, S. (1996). Semidefinite programming. SIAM Review, 38(1), 49-95. https://doi.org/10.1137/1038003
[8] Anjos, M.F. & Lasserre, J.B. (2012) Handbook on Semidefinite, Conic and Polynomial Optimization. International Series in Operations Research & Management Science, Springer New York, NY. https: //doi.org/10.1007/978-1-4614-0769-0
[9] Alizadeh, F. & Goldfarb, D. (2003). Second-order cone programming. Mathematical Programming, Ser. B, 95, 3-51. https://doi.org/10.1007/s1 0107-002-0339-5
[10] Lobo, M.S., Vandenberghe, L., Boyd, S., & Lebret, H. (1998). Applications of second order cone programming. Linear Algebra and Its Applications, 284, 193-228. https://doi.org/ 10.1016/S0024-3795(98)10032-0
[11] Luo, Z.-Q. & Sturm, J. F. (2000). Error analysis. In: H. Wolkowicz, R. Saigal, L. Vandenberghe (eds) Handbook of Semidefinite Programming. International Series in Operations Research & Management Science, vol 27. Springer, Boston, MA, 163-190.
[12] Meng, J., Cao, P., Huang, J., Lin, H., Chen, Y., & Cao, R. (2019) Second-order cone programming formulation of discontinuous deformation analysis. IJNME- International Journal for Numerical Methods in Engineering. 118(5), 243-57. https://doi.org/10.1002/nme.6006
[13] Wang, D., Chen, X., Lyu, Y., & Tang, C.(2019) Geotechnical localization analysis based on Cosserat continuum theory and second-order cone programming optimized finite element method. Computers and Geotechnics. 114, 103-118. https://doi.org/10.1016/j.compgeo.2019.103118
[14] Zhang, Y., & Zhang, L. (2019). New constraint qualifications and optimality conditions for second order cone programs. Set-Valued and Variational Analysis. 27, 693-712. https://do i.org/10.1007/s11228-018-0487-2
[15] Bonnans, J.F., & Shapiro, A. (2000). Perturbation analysis of optimization problems. Springer, New York.
[16] Kostyukova, O. I., Tchemisova, T. V., & Dudina, O. S. (2020). Immobile indices and CQ-free optimality criteria for linear copositive programming problems. Set-Valued and Variational Analysis. 28, 89-107. https: //doi.org/10.1007/s11228-019-00527-y
[17] Kapoor, M., Suneja, S. K., & Sharma, S. (2016). Generalized (Φ,ρ)-convexity in nonsmooth vector optimization over cones. An International Journal of Optimization and Control: Theories & Applications, 6(1), 1-7. https://doi.org/10.11121/ijocta.01.2016.00247 https://doi.org/10.11121/ijocta.01.2016. 00247
[18] Andreani, R., Martinez, J.M., Silva, P.S., & Ramos, A.( 2018). Strict constraint qualifications and sequential optimality conditions for constrained optimization. Mathematics of Operations Research online, 43, 693-717. https://doi.org/10.1287/moor.2017.0879
[19] Jeyakumar, V. (2008). Constraint qualifications characterizing Lagrangian duality in convex optimization. Journal of Optimization Theory and Applications, 136, 31-41. https://doi.or g/10.1007/s10957-007-9294-x
[20] Andreani, R., Fukuda, E. H., Haeser, G., Santos, D.O., & Secchin, L.D. (2024) Optimality conditions for nonlinear second-order cone programming and symmetric cone programming. Journal of Optimization Theory and Applications, 200, 1-13. https: //doi.org/10.1007/s10957-023-02338-6
[21] Gorokhovik, V.V. (2021). Step-affine functions, halfspaces, and separation of convex sets with applications to convex optimization problems. In: Proceedings of the Steklov Institute of Mathematics, 313(1), S83-S99. https://doi.or g/10.1134/S008154382103010X
[22] Kortanek, K. O., Yu, G., & Zhang, Q. (2021). Strong duality for standard convex programs. Mathematical Methods of Operations Research, 94(3), 413-436. https://doi.org/10.1007/s0 0186-021-00761-x
[23] Cochran, J.J., Cox, L.A., Jr., Keskinocak, P., Kharoufeh, J.P., Smith, J.C., & Solodov, M.V. (2011). Constraint qualifications. In: J.J. Cochran, L.A. Cox, P. Keskinocak,J.P. Kharoufeh, & J.C. Smith (eds.) Wiley Encyclopedia of Operations Research and Management Science. Wiley. h t t p s : //doi.org/10.1002/9780470400531
[24] Bonnans, J.F., & Ramirez, H. (2005) Perturbation analysis of second-order cone programming problems. Mathematical Programming, 104(2), 205-227. h t t p s : //doi.org/10.1007/s10107-005-0613-4
[25] Kruger, A.Y., Minchenko, L., Outrata, J.V.(2013) On relaxing the Mangasarian-Fromovitz constraint qualification. Positivity, 18, 171-189.https://doi.org/10.1007/s11117-013-023 8-4
[26] Andreani, R., Haeser, G., Mito, L.M., Ramirez, H., Santos, D.O., & Silveira, T.P. (2022) Naive constant rank-type constraint qualifications for multifold second-order cone programming and semidefinite programming. Optimization Letters, 16, 589-610. https://doi.org/10.1007/s11590 -021-01737-w
[27] Andersen, E. D., Roos, C., & Terlaky, T. (2002). Notes on duality in second order and p-order cone optimization. Optimization, 51(4), 627-643. http s://doi.org/10.1080/0233193021000030751
[28] Kostyukova, O., & Tchemisova, T. (2015). Convex SIP problems with finitely representable compact index sets: immobile indices and the properties of the auxiliary NLP problem, Set-Valued and Variational Analysis, 23(3), 519-546. https://doi.org/10.1007/s11228 -015-0320-0
[29] Tun¸cel, L., & Wolkowicz, H. (2013). Strong duality and minimal representations for cone optimization. Computational Optimization and Applications. 53, 619-648. https://doi.org/10.1007/s10589-012-9480-0
[30] Kostyukova, O., & Tchemisova, T. (2021).Structural properties of faces of the cone of copositive matrices. Mathematics, 9(21), 2698.https://doi.org/10.3390/math9212698
[31] Golshtein, E. G. (1971). Theory of duality in mathematical programming and its applications. Nauka. Moscow. (in Russian)
[32] Liu, M., & Pataki, G. (2018). Exact duals and short certificates of infeasibility and weak infeasibility in conic linear programming. Mathematical Programming, 167, 435-480. https://doi.org/10.1007/s10107-017-1136-5
[33] Ramana, M. V., Tun¸cel, L., & Wolkowicz, H. (1997). Strong duality for semidefinite programming. SIAM Journal on Optimization, 7(3), 641-662. https://doi.org/10.1137/S1 052623495288350
[34] Kostyukova, O. I., & Tchemisova, T. V.(2022). On strong duality in linear copositive programming. Journal of Global Optimization,83(3), 457-480. https://doi.org/10.1007/s10898-021-00995-3
[35] Kostyukova, O.I.,& Tchemisova,T.V. (2023) An exact explicit dual for the linear copositive programming problem. Optimization Letters, 17,107-120. https://doi.org/10.1007/s11590-022-01870-0