Dislocation hyperbolic augmented Lagrangian algorithm in convex programming
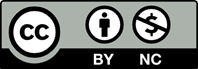
The dislocation hyperbolic augmented Lagrangian algorithm (DHALA) is a new approach to the hyperbolic augmented Lagrangian algorithm (HALA). DHALA is designed to solve convex nonlinear programming problems. We guarantee that the sequence generated by DHALA converges towards a Karush-Kuhn-Tucker point. We are going to observe that DHALA has a slight computational advantage in solving the problems over HALA. Finally, we will computationally illustrate our theoretical results.
[1] Tseng, P., & Bertsekas, D.P. (1993). On the con- vergence of the exponential multiplier method for convex programming. Mathematical Program- ming, 60, 1-19. https://doi.org/10.1007/BF01 580598
[2] Polyak, R.A. (2001). Log-sigmoid multipliers method in constrained optimization. Annals of Operations Research, 101, 427-460. https://do i.org/10.1023/A:1010938423538
[3] Polyak, R., & Teboulle, M. (1997). Nonlinear rescaling and proximal-like methods in convex op- timization. Mathematical Programming, 76, 265-284. https://doi.org/10.1007/BF02614440
[4] Kort, B.W., & Bertsekas, D.P. (1976). Combined primal-dual and penalty methods for convex pro- gramming. SIAM Journal on Control and Opti- mization, 14, 268-294. https://doi.org/10.1137/0314020
[5] Rockafellar, R.T. (1976). Augmented Lagrangians and applications of the proximal point algorithm in convex programming. Mathematics of Opera- tions Research, 1, 97-116. https://doi.org/10 .1287/moor.1.2.97
[6] Mallma-Ramirez, L. (2022). The Hyperbolic Aug- mented Lagrangian Algorithm. Ph.D. Thesis. Fed- eral University of Rio de Janeiro/COPPE, Rio de Janeiro, Brazil. https://www.cos.ufrj.br/up loadfile/publicacao/3038.pdf
[7] Xavier, A.E. (1982a). Penaliza¸c˜ao Hiperb´olica- Um Novo M´etodo para Resolu¸c˜ao de Problemas de Otimiza¸c˜ao . MSc Thesis. Federal University of Rio de Janeiro/COPPE, Rio de Janeiro, Brazil.
[8] Xavier, A.E. (1992). Penaliza¸c˜ao Hiperb´olica. Ph.D. Thesis. Federal University of Rio de Janeiro/COPPE, Rio de Janeiro, Brazil.
[9] Kanzow, C., & Steck, D. (2017). An example com-paring the standard and safeguarded augmented Lagrangian methods. Operations Research Let- ters, 45, 598-603. https://doi.org/10.1016/ j.orl.2017.09.005
[10] Xavier, A. E. (2001). Hyperbolic penalty: a new method for nonlinear programming with inequal- ities. International Transactions in Operational Research, 8, 659-671. https://doi.org/10.1 111/1475-3995.t01-1-00330
[11] Zangwill, W.I. (1967). Non-linear programming via penalty function. Management Science, 13, 344-358. https://doi.org/10.1287/mnsc.1 3.5.344
[12] Auslender, A., Teboulle, M., & Ben-Tiba, S.(1999). Interior proximal and multiplier meth- ods based on second order homogeneous kernels. Mathematics of Operations Research, 24, 645-668. https://doi.org/10.1287/moor.24.3.645
[13] Polyak, R.A. (2002). Nonlinear rescaling vs. smoothing technique in convex optimization. Mathematical Programming, 92, 197-235. https: //doi.org/10.1007/s101070100293
[14] Rockafellar, R.T. (1970). Convex Analysis. Princeton University Press, Princeton, NJ.
[15] Hartman, J.K. (1975). Iterative determination of parameters for an exact penalty function. Jour- nal of Optimization Theory and Applications, 16, 49-66. https://doi.org/10.1007/BF00935623
[16] Harwell Subroutine Library (2002). A collection of Fortran codes for large scale scientific compu- tation, https://www.hsl.rl.ac.uk/
[17] Hock, W., & Schittkowski, K. (1981). Test Exam- ples for Nonlinear Programming Codes. Lecture Notes in Economics and Mathematical Systems 187, Springer-Verlag, Berlin, Germany. https: //doi.org/10.1007/978-3-642-48320-2
[18] Tirkolaee, E.B., Goli, A., G¨(u)tmen, S., Weber,G.W., & Szwedzka, K. (2023). A novel model for sustainable waste collection arc routing prob- lem: Pareto-based algorithms. Annals of Opera- tions Research, 324, 189-214. https://doi.org/ 10.1007/s10479-021-04486-2
[19] Grapiglia, G.N. (2021). Worst-case evaluation complexity of a quadratic penalty method for nonconvex optimization. Optimization Methods and Software, 38, 781-803. https://doi.org/ 10.1080/10556788.2023.2189711
[20] Belloufi, M., Benzine, R., & Laskri, Y. (2013). Modification of the Armijo line search to satisfy the convergence properties of HS method. An In- ternational Journal of Optimization and Control: Theories & Applications (IJOCTA), 3, 145-152. https://doi.org/10.11121/ijocta.01.2013. 00141