Qualitative behavior of stiff ODEs through a stochastic approach
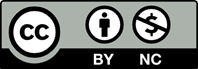
In the last few decades, stiff differential equations have attracted a great deal of interest from academic society, because much of the real life is covered by stiff behavior. In addition to importance of producing model equations, capturing an exact behavior of the problem by dealing with a solution method is also handling issue. Although there are many explicit and implicit numerical methods for solving them, those methods cannot be properly applied due to their computational time, computational error or effort spent for construction of a structure. Therefore, simulation techniques can be taken into account in capturing the stiff behavior. In this respect, this study aims at analyzing stiff processes through stochastic approaches. Thus, a Monte Carlo based algorithm has been presented for solving some stiff ordinary differential equations and system of stiff linear ordinary differential equations. The produced results have been qualitatively and quantitatively discussed.
[1] Curtis, C.F. & Hirschfelder, J.O. (1950). Integration of Stiff Equations. Proceedings of the National Academy of Sciences of the United States of America, 38(3), 235-243.
[2] Shampine, L.F. & Gear, C.W. (1976). A User’s View of Solving Ordinary Differential Equations.Department of Computer Science University of Illinois at Urbana.
[3] Hairer, E. & Wanner, G. (2000). Solving Ordinary Differential Equations II. Springer, Second Edition.
[4] Birge, J.R. & Louveaux, F. (2011). Introduction to Stochastic Programming. Springer Science and Business Media.
[5] Kroese, D.P. & Chan, J.C. (2016). Statistical Modeling and Computation. Springer.
[6] Rubinstein, R.Y. & Kroese, D.P. (2016). Simulation and the Monte Carlo Method (Vol. 10). John Wiley & Sons.
[7] Fishman, G. (2013). Monte Carlo: Concepts, Algorithms, and Applications. Springer Science and Business Media.
[8] Kroese, D.P., Taimre, T., & Botev, Z.I.(2013). Handbook of Monte Carlo Methods (Vol. 706). John Wiley and Sons.
[9] Chapra, C.S. (2017). Applied Numerical Methods with MATLAB for Engineers and Scientists.McGraw Hill.
[10] Dekking, F.M. (2005). A Modern Introduction to Probability and Statistics: Understanding why and how. Springer Science and Business Media.
[11] Uslu, H. (2018). Behavior of First Order Differential Equations Through aMonte Carlo Based Algorithm. MSc Thesis, Yildiz Technical University, Istanbul, Turkey.
[12] Sari, M., Uslu, H. & Cosgun, T. (2018). The Qualitative Behavior of Some Stiff ODEs Through Stochastic Methods. The International Conference on Applied Mathematics in Engineering (ICAME'18), Balikesir, Turkey.
[13] Uslu, H. & Sari, M. (2019). Monte Carlo based stochastic approach for first order nonlinear ODE systems. Pamukkale Journal of Engineering Sciences, 26(1), 133-139.