New complex-valued activation functions
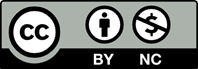
We present a new type of activation functions for a complex-valued neural network (CVNN). A proposed activation function is constructed such that it fixes a given ellipse. We obtain an application to a complex-valued Hopfield neural network (CVHNN) using a special form of the introduced complex functions as an activation function. Considering the interesting geometric properties of the plane curve ellipse such as focusing property, we emphasize that these properties may have possible applications in various neural networks.
[1] Ceylan, M., O(¨)zbay, Y., U>can, O.N., Y1ld1r1m,E. (2010). A novel method for lung segmenta- tion on chest CT images: complex-valued ar- tificial neural network with complex wavelet transform. Turk. J. Elec. Eng. and Comp. Sci., 18(4), 613-623.
[2] Ceylan, M., Ya>sar, H. (2016). A novel ap- proach for automatic blood vessel extraction in retinal images: complex ripplet-I transform and complex valued artificial neural network. Turk. J. Elec. Eng. and Comp. Sci., 24(4), 3212-3227.
[3] Gandal, A.S., Kalra, P.K., Chauhan, D.S.(2007). Performance evaluation of complex valued neural networks using various error functions. International Journal of Electrical, Computer, Energetic, Electronic and Com- munication Engineering, 1(5), 732-737.
[4] Hirose, A. (2009). Complex-valued neural net- works: The merits and their origins. Proceed- ings of the Internatinal Joint Conference on Neural Networks (IJCNN), Atlanta, June 14- 19, 1237-1244.
[5] Jalab, H.A., Ibrahim, R. W. (2011). New ac- tivation functions for complex-valued neural network. International Journal of the Physi- cal Sciences, 6(7), 1766-1772.
[6] Oladipo, A.T., Gbolagade, M. (2014). Some subordination results for logisticsigmoid acti- vation function in the space of univalent func- tions. Advances in Computer Science and En- gineering, 12(2), 61-79.
[7] Singh, R.G., Singh, A.P. (2015). Multi- ple complex extreme learning machine using holomorphic mapping for prediction of wind power generation system. International Jour- nal of Computer Applications, 123(18), 24-33.
[8] Zimmermann, H.G., Minin, A., Kusherbaeva, V. (2011). Comparison of the complex val- ued and real valued neural networks trained with gradient descent and random search al- gorithms. ESANN 2011 proceedings, Euro- pean Symposium on Artificial Neural Net- works, Computational Intelligence and Ma- chine Learning. Bruges (Belgium) 27-29 April 2011.
[9] Kim, T., Adal1, T. (2002) Fully complex multi-layer perceptron network for nonlinear signal processing. J. VLSI Sig. Process., 32, 29-43.
[10] Frantz, M. (1994). A focusing property of the ellipse. Amer. Math. Monthly, 101(3), 250- 258.
[11] Wilker, J.B. (1995). Further thoughts on a focusing property of the ellipse. Bull. Belg. Math. Soc., 2, 153-159.
[12] Connett, J.E. (1992). Trapped re丑ections?. Amer. Math. Monthly, 99, 178-179.
[13] Di Concilio, A., Guadagni, C., Peters, J.F., Ramanna, S. (2018). Descriptive proximi- ties. Properties and interplay between clas- sical proximities and overlap. Math. Com- put. Sci., 12(1), 91-106. arXiv:1609.06246. MR3767897.
[14] Ferrer, S., HanBmann, H., Palaci/an, J., Yan- guas, P. (2002). On perturbed oscillators in 1-1-1 resonance: the case of axially symmet- ric cubic potentials. J. Geom. Phys., 40(3-4), 320-369.
[15] Grandon, J., Derpich, I. (2011). A Heuris- tic for the Multi-knapsack Problem. WSEAS Transactions on Mathematics, 10(3), 95-104.
[16] Kanan, H.R., Faez, K., Ezoji, M. (2006). An e伍cient face recognition system using a new optimized localization method, In Pat- tern Recognition. ICPR 2006, 18th Interna- tional Conference on Vol. 3, 564-567.
[17] Kellner, M.A., Hanning, T., Farr, H. (2002). Real-time analysis of the grain on wooden planks, Machine Vision Applications in In- dustrial Inspection X. Vol. 4664. Interna- tional Society for Optics and Photonics.
[18] Kobayashi, M., (2013). Hyperbolic Hopfield neural networks. IEEE Trans. Neural Netw. Learn Syst. 24(2), 335-341.
[19] Li, J., Zhang, J. (2004). Bifurcations oftrav- elling wave solutions for the generalization form of the modified KdV equation. Chaos Solitons Fractals 21(4), 889-913.
[20] Peters, J.F. (2018). Proximal Vortex Cycles and Vortex Nerves. Non-Concentric, Nesting, Possibly Overlapping Homology Cell Com- plexes. Journal of Mathematical Sciences and Modelling, 1 (2), 80-85. arXiv:1805.03998.
[21] Zhang, G., Jayas, D.S., White, N.D. (2005). Separation of touching grain kernels in an im- age by ellipse fitting algorithm. Biosystems engineering, 92(2), 135-142.
[22] Wolfram Research. (2019). Inc., Mathemat- ica, Version 12.0, Champaign, IL.
[23] Beardon, A.F. (1983). The geometry of dis- crete groups. Graduate texts in mathematics, vol 91. Springer, New York.
[24] Jones, G.A., Singerman, D. (1987). Com- plex functions. An algebraic and geometric viewpoint, Cambridge University Press, Cam- bridge.
[25] Kwok, Y.K. (2010). Applied complex vari- ables for scientists and engineers. Cambridge University Press, New York.
[26] Mandic, D.P. (2000). The use of M¨obius transformations in neural networks and signal processing. Neural Networks for Signal Pro- cessing X, 1, 185-194.
[27] O(¨)zdemir, N., I(˙)skender, B.B., O(¨)zg¨ur, N.Y.(2011). Complex valued neural network with M¨obius activation function. Commun. Non- linear Sci. Numer. Simul., 16(12), 4698-4703. [28] Hopfield, J.J. (1982). Neural networks and physical systems with emergent collective computational abilities. Proc. Nat. Acad. Sci. United States Amer., 79(8), 2554-2558.
[29] Kobayashi, M. (2017). Symmetric complex- valued Hopfield neural networks. IEEE Trans. Neural Netw. Learn Syst., 28(4), 1011-1015.
[30] Khalil, H.K. (1996). Nonlinear systems. 2nd ed. United States of America: Prentice Hall.
[31] Kuroe, Y., Yoshida, M., Mori, T. (2003). On activation functions for complex-valued neural networks - existence of energy func- tions. In: Kaynak O et al., editors. ICANN/ICONIP 2003. LNCS 2714 . Berlin Heidelberg: Springer-Verlag, 985-992.