Optimal control of fractional integro-differential systems based on a spectral method and grey wolf optimizer
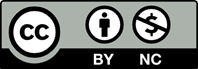
This paper elaborated an effective and robust metaheuristic algorithm with acceptable performance based on solution accuracy. The algorithm applied in solution of the optimal control of fractional Volterra integro-differential (FVID) equation which be substituted by nonlinear programming (NLP). Subsequently the FIVD convert the problem to a NLP by using spectral collocation techniques and thereafter we execute the grey wolf optimizer (GWO) to improve the speed and accuracy and find the solutions of the optimal control and state as well as the optimal value of the cost function. It is mentioned that the utilization of the GWO is simple, due to the fact that the GWO is global search algorithm, the method can be applied to find optimal solution of the NLP. The efficiency of the proposed scheme is shown by the results obtained in comparison with the local methods. Further, some illustrative examples introduced with their approximate solutions and the results of the present approach compared with those achieved using other methods.
[1] Diethelm, K., Ford, N.J. and Freed, A.D.(2004). Detailed error analysis for a fractional Adams method. Numerical Algorithms,36, 31–52.
[2] Huang, L., Li, X.F., Zhao, Y.L. and Duan, X.Y. (2011). Approximate solution of frac- tional integro-diferential equations by Taylor expansion method. Computers & Mathemat- ics with Applications, 62(3), 1127–1134.
[3] Tohidi, E. and Nik, H.S. (2015). A bessel col- location method for solving fractional optimal control problems. Applied Mathematical Mod- elling, 39(2), 455–465.
[4] Keshavarz, E., Ordokhani, Y. and Razzaghi, M. (2015). A numerical solution for fractional optimal control problems via Bernoulli poly- nomials. Journal of Vibration and Control, 22(18), 3889–3903.
[5] Zaky, M.A. and Machado, J.A.T. (2017). On the formulation and numerical simulation of distributed-order fractional optimal control problems. Communications in Nonlinear Sci- ence and Numerical Simulation, 52, 177–189.
[6] Salati, A.B., Shamsi, M., Torres, D.F.M.(2019). Direct transcription methods based on fractional integral approximation formulas for solving nonlinear fractional optimal con- trol problems. Communications in Nonlinear Science and Numerical Simulation, 67, 334– 350.
[7] Youssri, Y.H., and Abd-Elhameed, W.M.(2018). Spectral tau algorithm for solving a class of fractional optimal control prob- lems via Jacobi polynomials. An International Journal of Optimization and Control: Theo- ries & Applications (IJOCTA), 8(2), 152–160.
[8] Youssri, Y.H. and Hafez, R.M. (2019). Cheby- shev collocation treatment of VolterraFred- holm integral equation with error analysis. Arabian Journal of Mathematics, 1–10.
[9] Mirjalili, S.A., Mirjalili, S.M. and Lewis, A.(2014). Grey wolf optimizer. Advances in En- gineering Software, 69, 46–61.
[10] Baleanu, D., Diethelm, K., Scalas, E. and Trujillo, J.J. (2012). Fractional calculus models and numerical methods. Non- linearity and Chaos, Series on Complexity, World Scientific.
[11] Costabile, F., Dellaccio, F. and Gualtieri, M.I. (2006). A new approach to Bernoulli polynomials. Rendiconti di Matematica, Serie VII, 26, 1–12.
[12] Arfken G. (1985). Mathematical methods for physicists. 3rdedn. San Diego, CA: Academic Press.
[13] Kreyszig, E. (1978). Introductory functional analysis with applications. New York: John Wiley and Sons.
[14] Yuan, Y.X. (2000). A review of trust region algorithms for optimization. In Iciam, 99(1), 271–282.
[15] Sadjadi, S.J. and Ponnambalam, K. (1999). Advances in trust region algorithms for constrained optimization. Applied Numerical Mathematics, 29(3), 423–443.
[16] Faris, H., Aljarah, I., Al-Betar, M. A. and Mirjalili, S. (2018). Grey wolf optimizer: are- view of recent variants and applications. Neu- ral computing and applications, 30(2), 413– 435.
[17] Wang, J.S. and Li, S.X. (2019). An improved grey wolf optimizer based on diferential evo- lution and elimination mechanism. Scientific reports, 9(1), 71–81.
[18] Liu, H., Hua, G., Yin, H. and Xu, Y. (2018). An intelligent grey wolf optimizer algorithm for distributed compressed sensing. Computa- tional intelligence and neuroscience, 2018.
[19] Gupta, S. and Deep, K. (2018). An opposition-based chaotic grey wolf optimizer for global optimisation tasks. Journal of Ex- perimental & Theoretical Artificial Intelli- gence, 1–29.
[20] Abdo, M., Kamel, S., Ebeed, M., Yu, J. and Jurado, F. (2018). Solving non-smooth opti- mal power 丑ow problems using a developed grey wolf optimizer. Energies, 11(7), 1692.
[21] Pradhan, M., Roy, P. K. and Pal, T. (2016). Grey wolf optimization applied to economic load dispatch problems. International Jour- nal of Electrical Power & Energy Systems, 83, 325–334.
[22] Tung, N.S. and Chakravorty, S. (2015). Grey Wolf optimization for active power dispatch planning problem considering generator con- straints and valve point efect. International Journal of Hybrid Information Technology, 8(12), 117–134.
[23] Maleknejad, K., Ebrahimzadeh, A. (2014). Optimal control of volterra integro- diferential systems based on legendre wavelets and collocation method. Journal of Mathematical, Computational, Physical an Quantum Engineering,8(7), 1040–1044.