Machine learning-generated compression modulus database for 3D printing of gelatin methacryloyl
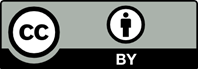
3D bioprinting enables the fabrication of printable tissues, including those for neural, cartilage, and skin repair. The mechanical properties, especially stiffness, of 3D-bioprinted scaffolds are crucial for modulating cell adhesion, growth, migration, and differentiation. The stiffness of a scaffold can be adjusted post-printing by modifying the hydrogel concentration, crosslinker concentration, light intensity during photocrosslinking, and duration of crosslinking. The optimization of these conditions to produce the desired scaffold stiffness for a particular cell type or application is a time-consuming and rigorous process. This study developed an innovative approach to predict the compression modulus of 3D-printed gelatin methacryloyl (GelMA) scaffolds using the Bayesian optimization (BO) algorithm. Through just 10 iterations (75 experimental data points), the model was able to predict > 13,000 possible compression modulus values in a search space comprising four independent variables (GelMA concentration, crosslinker concentration, ultraviolet light [UV] distance, and UV exposure time). This approach can be utilized in other photocrosslinkable bioinks for 3D printing that have a myriad of pre- or post-printing parameters that can affect scaffold stiffness.
- Hashizume R, Fujimoto KL, Hong Y, et al. Morphological and mechanical characteristics of the reconstructed rat abdominal wall following use of a wet electrospun biodegradable polyurethane elastomer scaffold. Biomaterials. 2010;31(12):3253-3265. doi: 10.1016/j.biomaterials.2010.01.051
- Simmons CA, Alsberg E, Hsiong S, Kim WJ, Mooney DJ. Dual growth factor delivery and controlled scaffold degradation enhance in vivo bone formation by transplanted bone marrow stromal cells. Bone. 2004;35(2): 562-569. doi: 10.1016/j.bone.2004.02.027
- Chung JHY, Kade JC, Jeiranikhameneh A, et al. 3D hybrid printing platform for auricular cartilage reconstruction. Biomed Phys Eng Express. 2020;6(3):035003. doi: 10.1088/2057-1976/ab54a7
- Daikuara LY, Yue Z, Skropeta D, Wallace GG. In vitro characterisation of 3D printed platelet lysate-based bioink for potential application in skin tissue engineering. Acta Biomater. 2021;123:286-297. doi: 10.1016/j.actbio.2021.01.021
- Hospodiuk M, Dey M, Sosnoski D, Ozbolat IT. The bioink: a comprehensive review on bioprintable materials. Biotechnol Adv. 2017;35(2):217-239. doi: 10.1016/j.biotechadv.2016.12.006
- Floren M, Bonani W, Dharmarajan A, Motta A, Migliaresi C, Tan W. Human mesenchymal stem cells cultured on silk hydrogels with variable stiffness and growth factor differentiate into mature smooth muscle cell phenotype. Acta Biomater. 2016;31:156-166. doi: 10.1016/j.actbio.2015.11.051
- Macri-Pellizzeri L, De-Juan-Pardo EM, Prosper F, Pelacho B. Role of substrate biomechanics in controlling (stem) cell fate: implications in regenerative medicine. J Tissue Eng Regene Med. 2018;12(4):1012-1019. doi: 10.1002/term.2586
- Yi B, Xu Q, Liu W. An overview of substrate stiffness guided cellular response and its applications in tissue regeneration. Bioact Mater. 2022;15:82-102. doi: 10.1016/j.bioactmat.2021.12.005
- Lv H, Wang H, Zhang Z, et al. Biomaterial stiffness determines stem cell fate. Life Sci. 2017;178:42-48. doi: 10.1016/j.lfs.2017.04.014
- Querceto S, Santoro R, Gowran A, et al. The harder the climb the better the view: the impact of substrate stiffness on cardiomyocyte fate. J Mol Cell Cardiol. 2022;166:36-49. doi: 10.1016/j.yjmcc.2022.02.001
- Huang Y, Xu K, Liu J, Dai G, Yin J, Wei P. Promotion of adrenal pheochromocytoma (PC-12) cell proliferation and outgrowth using Schwann cell-laden gelatin methacrylate substrate. Gels. 2022;8(2):84. doi: 10.3390/gels8020084
- Nichol JW, Koshy ST, Bae H, Hwang CM, Yamanlar S, Khademhosseini A. Cell-laden microengineered gelatin methacrylate hydrogels. Biomaterials. 2010;31(21):5536-5544. doi: 10.1016/j.biomaterials.2010.03.064
- Fan Y, Yue Z, Lucarelli E, Wallace GG. Hybrid printing using cellulose nanocrystals reinforced GelMA/HAMA hydrogels for improved structural integration. Adv Healthc Mater. 2020;9(24):2001410. doi: 10.1002/adhm.202001410
- Sharifi S, Sharifi H, Akbari A, Chodosh J. Systematic optimization of visible light-induced crosslinking conditions of gelatin methacryloyl (GelMA). Sci Rep. 2021;11(1):23276. doi: 10.1038/s41598-021-02830-x
- Wu Y, Xiang Y, Fang J, et al. The influence of the stiffness of GelMA substrate on the outgrowth of PC12 cells. Biosci Rep. 2019;39(1): BSR20181748. doi: 10.1042/BSR20181748
- Yin J, Yan M, Wang Y, Fu J, Suo H. 3D bioprinting of low-concentration cell-laden gelatin methacrylate (GelMA) bioinks with a two-step cross-linking strategy. ACS Appl Mater Interfaces. 2018;10(8):6849-6857. doi: 10.1021/acsami.7b16059
- Chung JHY, Naficy S, Yue Z, et al. Bio-ink properties and printability for extrusion printing living cells. Biomater Sci. 2013;1(7):763-773. doi: 10.1039/c3bm00012e
- Her GJ, Wu H-C, Chen M-H, Chen M-Y, Chang S-C, Wang T-W. Control of three-dimensional substrate stiffness to manipulate mesenchymal stem cell fate toward neuronal or glial lineages. Acta Biomater. 2013;9(2):5170-5180. doi: 10.1016/j.actbio.2012.10.012
- O’Connell CD, Zhang B, Onofrillo C, et al. Tailoring the mechanical properties of gelatin methacryloyl hydrogels through manipulation of the photocrosslinking conditions. Soft Matter. 2018;14(11):2142-2151. doi: 10.1039/c7sm02187a
- Freeman S, Calabro S, Williams R, Jin S, Ye K. Bioink formulation and machine learning-empowered bioprinting optimization. Front Bioeng Biotechnol. 2022;10:913579. doi: 10.3389/fbioe.2022.913579
- Ruberu K, Senadeera M, Rana S, et al. Coupling machine learning with 3D bioprinting to fast track optimisation of extrusion printing. Appl Mater Today. 2021;22:100914. doi: 10.1016/j.apmt.2020.100914
- Shin J, Lee Y, Li Z, Hu J, Park SS, Kim K. Optimized 3D bioprinting technology based on machine learning: a review of recent trends and advances. Micromachines. 2022;13(3):363. doi: 10.3390/mi13030363
- Sun J, Yao K, An J, Jing L, Huang K, Huang D. Machine learning and 3D bioprinting. Int J Bioprint. 2023;9(4):717. doi: 10.18063/ijb.717
- Yu C, Jiang J. A perspective on using machine learning in 3D bioprinting. Int J Bioprint. 2020;6(1):253. doi: 10.18063/ijb.v6i1.253
- An J, Chua CK, Mironov V. Application of machine learning in 3D bioprinting: focus on development of big data and digital twin. Int J Bioprint. 2021;7(1):342. doi: 10.18063/ijb.v7i1.342
- Tian S, Stevens R, McInnes BT, Lewinski NA. Machine assisted experimentation of extrusion-based bioprinting systems. Micromachines. 2021;12(7):780. doi: 10.3390/mi12070780
- Brochu E, Cora VM, Freitas ND. A tutorial on Bayesian optimization of expensive cost functions, with application to active user modeling and hierarchical reinforcement learning. ArXiv. 2010;1012:2599 doi: 10.48550/arXiv.1012.2599
- Bishop CM, Nasrabadi NM. Pattern recognition and machine learning. J Electronic Imaging. 2007;16(4): 049901. doi: 10.1117/1.2819119
- Engler AJ, Sen S, Sweeney HL, Discher DE. Matrix elasticity directs stem cell lineage specification. Cell. 2006;126(4):677-689. doi: 10.1016/j.cell.2006.06.044
- Aregueta-Robles UA, Martens PJ, Poole-Warren LA, Green RA. Tissue engineered hydrogels supporting 3D neural networks. Acta Biomater. 2019;95: 269-284. doi: 10.1016/j.actbio.2018.11.044
- Chatterjee K, Lin-Gibson S, Wallace WE, et al. The effect of 3D hydrogel scaffold modulus on osteoblast differentiation and mineralization revealed by combinatorial screening. Biomaterials. 2010;31(19):5051-5062. doi: 10.1016/j.biomaterials.2010.03.024