Manufacturing multi-organs database: A comprehensive, predictive, and analytical biofabrication database
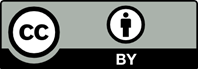
Biofabrication, broadly described as “a process that results in a defined product with biological function,” has undergone a revolution in recent years. This revolution has led to an explosion of literature containing valuable data and insights on bioactive materials and machine learning-aided design. However, the accessibility and comprehension of this rich data source remain a challenge, necessitating the creation of a comprehensive database. Herein, we present the manufacturing multi-organs database (MMDB), a real-time updating database developed to foster an all-inclusive understanding of biofabrication by leveraging machine learning for standardized analysis of material properties and manufacturing processes. The MMDB aids in identifying commonly used cells, materials, and culture strategies in biofabrication by analyzing over 5000 papers related to 37 human organs. Leveraging machine learning models, it predicts optimal printing parameters and organ functionality metrics, thereby streamlining experimental designs and reducing costs. In addition, MMDB offers knowledge services that encompass hotspot analysis, trend identification, international collaboration analysis, and comprehensive knowledge maps of organ functions and biomaterials. We believe that the MMDB, serving as a crucial and readily accessible knowledge base, will fundamentally facilitate the design and optimization of biofabrication experiments. Moreover, by accelerating the discovery of optimal parameters, the MMDB has the potential to offer invaluable insights into organ function, propelling the field of biofabrication toward more efficient and effective organ manufacturing.
- Mironov V, Boland T, Trusk T, Forgacs G, Markwald RR. Organ printing: Computer-aided jet-based 3D tissue engineering. Trends Biotechnol. 2003;21(4):157-161. doi: 10.1016/S0167-7799(03)00033-7
- Murphy SV, Atala A. 3D bioprinting of tissues and organs. Nat Biotechnol. 2014;32(8):773-785. doi: 10.1038/nbt.2958
- Ozbolat IT, Hospodiuk M. Current advances and future perspectives in extrusion-based bioprinting. Biomaterials. 2016;76:321-343. doi: 10.1016/j.biomaterials.2015.10.076
- Zhang YS, Yue K, Aleman J, et al. 3D bioprinting for tissue and organ fabrication. Ann Biomed Eng. 2017;45(1):148-163. doi: 10.1007/s10439-016-1612-8
- Celikkin N, Presutti D, Maiullari F, et al. Tackling current biomedical challenges with frontier biofabrication and organ-on-a-chip technologies. Front Bioeng Biotechnol. 2021;9:732130. doi: 10.3389/fbioe.2021.732130
- Peng W, Datta P, Wu Y, et al. Challenges in bio-fabrication of organoid cultures. In: Cell Biology and Translational Medicine, Volume 3: Stem Cells, Bio-materials and Tissue Engineering. Springer, Cham, 2018, p53-71. doi: 10.1007/5584_2018_216
- Paxton N, Smolan W, Böck T, Melchels F, Groll J, Jungst T. Proposal to assess printability of bioinks for extrusion-based bioprinting and evaluation of rheological properties governing bioprintability. Biofabrication. 2017;9(4):044107. doi: 10.1088/1758-5090/aa8dd8
- Pagac M, Hajnys J, Ma QP, et al. A review of vat photopolymerization technology: Materials, applications, challenges, and future trends of 3d printing. Polymers (Basel). 2021;13(4):598. doi: 10.3390/polym13040598
- Bertassoni LE. Bioprinting of complex multicellular organs with advanced functionality-recent progress and challenges ahead. Adv Mater. 2022;34(3):e2101321. doi: 10.1002/adma.202101321
- Mandrycky C, Wang Z, Kim K, Kim DH. 3D bioprinting for engineering complex tissues. Biotechnol Adv. 2016;34(4):422-434. doi: 10.1016/j.biotechadv.2015.12.011
- Hutmacher DW. Scaffolds in tissue engineering bone and cartilage. Biomaterials. 2000;21(24):2529-2543. doi: 10.1016/s0142-9612(00)00121-6
- Ng WL, Lee JM, Zhou M, et al. Vat polymerization-based bioprinting-process, materials, applications and regulatory challenges. Biofabrication. 2020;12(2):022001. doi: 10.1088/1758-5090/ab6034
- Griffith LG, Naughton G. Tissue engineering--current challenges and expanding opportunities. Science. 2002;295(5557):1009-1014. doi: 10.1126/science.1069210
- Langer R, Vacanti JP. Tissue engineering. Science. 1993;260(5110):920-926. doi: 10.1126/science.8493529
- Atala A, Kasper FK, Mikos AG. Engineering complex tissues. Sci Transl Med. 2012;4(160):160rv12. doi: 10.1126/scitranslmed.3004890
- Zhang B, Radisic M. Organ-on-a-chip devices advance to market. Lab Chip. 2017;17(14):2395-2420. doi: 10.1039/C6LC01554A
- Huh D, Hamilton GA, Ingber DE. From 3D cell culture to organs-on-chips. Trends Cell Biol. 2011;21(12):745-754. doi: 10.1016/j.tcb.2011.09.005
- Li J, Liang W, Chen Z, et al. OOCDB: A comprehensive, systematic, and real-time organs-on-a-chip database. Genomics Proteomics Bioinformatics. 2023;21(2):243-258. doi: 10.1016/j.gpb.2023.01.001
- Mahadik B, Margolis R, McLoughlin S, et al. An open-source bioink database for microextrusion 3D printing. Biofabrication. 2022;15(1):015008. doi: 10.1088/1758-5090/ac933a
- Hemphill EE, Dharia AP, Lee C, et al. SCLD: A stem cell lineage database for the annotation of cell types and developmental lineages. Nucleic Acids Res. 2011;39(suppl_1):D525-D533. doi: 10.1093/nar/gkq941
- Hinton GE, Salakhutdinov RR. Reducing the dimensionality of data with neural networks. Science. 2006;313(5786):504-507. doi: 10.1126/science.1127647
- Skardal A, Atala A. Biomaterials for integration with 3-D bioprinting. Ann Biomed Eng. 2015;43(3):730-746. doi: 10.1007/s10439-014-1207-1
- Murphy SV, Skardal A, Atala A. Evaluation of hydrogels for bio‐printing applications. J Biomed Mater Res A. 2013;101(1):272-284. doi: 10.1002/jbm.a.34326
- White J. PubMed 2.0. Med Ref Serv Q. 2020;39(4):382-387. doi: 10.1080/02763869.2020.1826228
- Burnham JF. Scopus database: A review. Biomed Digit Libr. 2006;3(1):1-8. doi: 10.1186/1742-5581-3-1
- Yao T, Zhang Y, Lv M, Zang G, Ng SS, Chen X. Advances in 3D cell culture for liver preclinical studies. Acta Biochim Biophys Sin (Shanghai). 2021;53(6):643-651. doi: 10.1093/abbs/gmab046
- Deng J, Wei W, Chen Z, et al. Engineered liver-on-a-chip platform to mimic liver functions and its biomedical applications: A review. Micromachines (Basel). 2019;10(10):676. doi: 10.3390/mi10100676
- Choudhury D, Anand S, Naing MW. The arrival of commercial bioprinters-towards 3D bioprinting revolution! Int J Bioprint. 2018;4(2):139. doi: 10.18063/IJB.v4i2.139
- Pedregosa F, Varoquaux G, Gramfort A, et al. Scikit-learn: Machine learning in Python. J Mach Learn Res. 2011;12:2825-2830.
- Chen T, Guestrin C. Xgboost: A Scalable Tree Boosting System. In: Proceedings of the 22nd Acm Sigkdd International Conference on Knowledge Discovery and Data Mining, 2016.
- Sharma P, Li Y. Self-Supervised Contextual Keyword and Keyphrase Retrieval with Self-Labelling, 2019.
- MacQueen J. Some Methods for Classification and Analysis of Multivariate Observations. In: Proceedings of the Fifth Berkeley Symposium on Mathematical Statistics and Probability. Oakland, CA, USA, 1967.