Advances in liquid biopsy: Computational and artificial intelligence approaches in cancer research
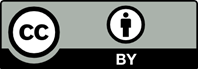
Liquid biopsy analysis has emerged as a promising approach for non-invasive cancer monitoring and diagnosis. This review provides an overview of the current landscape and future potential of liquid biopsy in cancer research, with a particular focus on the computational methodologies and techniques utilized for liquid biopsy data analysis and interpretation. The challenges and opportunities in extracting meaningful insights from the vast array of genomic, epigenomic, transcriptomic, and proteomic data are discussed, as well as the possibilities and current pitfalls of artificial intelligence approaches. In addition, the benefits and limitations of integrating multimodal cancer research data are covered, with a focus on advancing precision oncology and personalized medicine. By providing a critical assessment of the field, this review aims to foster knowledge about the available computational approaches and facilitate the choice of the most appropriate methodology for in silico investigation of liquid biopsy data, ultimately enhancing research endeavors and disease management strategies. Most of the works discussed in this review have emerged within the past 5 years, indicating a rapidly growing interest in this technique.
- Domínguez-Vigil IG, Moreno-Martínez AK, Wang JY, Roehrl MHA, Barrera-Saldaña HA. The dawn of the liquid biopsy in the fight against cancer. Oncotarget. 2018;9(2):2912-2922. doi: 10.18632/oncotarget.23131
- Louis DN, Perry A, Reifenberger G, et al. The 2016 World Health Organization classification of tumors of the central nervous system: A summary. Acta Neuropathol (Berl). 2016;131(6):803-820. doi: 10.1007/s00401-016-1545-1
- Eibl RH, Schneemann M. Liquid biopsy and primary brain tumors. Cancers (Basel). 2021;13(21):5429. doi: 10.3390/cancers13215429
- Mittal K, Koon H, Elson P, et al. Dual VEGF/VEGFR inhibition in advanced solid malignancies: Clinical effects and pharmacodynamic biomarkers. Cancer Biol Ther. 2014;15(8):975-981. doi: 10.4161/cbt.29187
- Jin Z, Sinicrope FA. Advances in the therapy of BRAF V600E metastatic colorectal cancer. Expert Rev Anticancer Ther. 2019;19(9):823-829. doi: 10.1080/14737140.2019.1661778
- Diaz LA Jr., Williams RT, Wu J, et al. The molecular evolution of acquired resistance to targeted EGFR blockade in colorectal cancers. Nature. 2012;486(7404):537-540. doi: 10.1038/nature11219
- Amelio I, Bertolo R, Bove P, et al. Liquid biopsies and cancer omics. Cell Death Discov. 2020;6(1):131. doi: 10.1038/s41420-020-00373-0
- Pinzani P, D’Argenio V, Del Re M, et al. Updates on liquid biopsy: Current trends and future perspectives for clinical application in solid tumors. Clin Chem Lab Med. 2021;59(7):1181-1200. doi: 10.1515/cclm-2020-1685
- Schwarze K, Buchanan J, Fermont JM, et al. The complete costs of genome sequencing: A microcosting study in cancer and rare diseases from a single center in the United Kingdom. Genet Med. 2020;22(1):85-94. doi: 10.1038/s41436-019-0618-7
- Wan JCM, Heider K, Gale D, et al. ctDNA monitoring using patient-specific sequencing and integration of variant reads. Sci Transl Med. 2020;12(548):eaaz8084. doi: 10.1126/scitranslmed.aaz8084
- Rose Brannon A, Jayakumaran G, Diosdado M, et al. Enhanced specificity of clinical high-sensitivity tumor mutation profiling in cell-free DNA via paired normal sequencing using MSK-ACCESS. Nat Commun. 2021;12(1):3770. doi: 10.1038/s41467-021-24109-5
- Bauml J, Levy B. Clonal hematopoiesis: A new layer in the liquid biopsy story in lung cancer. Clin Cancer Res. 2018;24(18):4352-4354. doi: 10.1158/1078-0432.CCR-18-0969
- Bettegowda C, Sausen M, Leary RJ, et al. Detection of circulating tumor DNA in early- and late-stage human malignancies. Sci Transl Med. 2014;6(224):224ra24. doi: 10.1126/scitranslmed.3007094
- Van Wezel EM, Zwijnenburg D, Zappeij-Kannegieter L, et al. Whole-genome sequencing identifies patient-specific DNA minimal residual disease markers in neuroblastoma. J Mol Diagn. 2015;17(1):43-52. doi: 10.1016/j.jmoldx.2014.09.005
- Wang Y, Li L, Cohen JD, et al. Prognostic potential of circulating tumor DNA measurement in postoperative surveillance of nonmetastatic colorectal cancer. JAMA Oncol. 2019;5(8):1118-1123. doi: 10.1001/jamaoncol.2019.0512
- Newman AM, Bratman SV, To J, et al. An ultrasensitive method for quantitating circulating tumor DNA with broad patient coverage. Nat Med. 2014;20(5):548-554. doi: 10.1038/nm.3519
- Phallen J, Sausen M, Adleff V, et al. Direct detection of early-stage cancers using circulating tumor DNA. Sci Transl Med. 2017;9(403):eaan2415. doi: 10.1126/scitranslmed.aan2415
- Abbosh C, Birkbak NJ, Wilson GA, et al. Phylogenetic ctDNA analysis depicts early-stage lung cancer evolution. Nature. 2017;545(7655):446-451. doi: 10.1038/nature22364
- Adalsteinsson VA, Ha G, Freeman SS, et al. Scalable whole-exome sequencing of cell-free DNA reveals high concordance with metastatic tumors. Nat Commun. 2017;8(1):1324. doi: 10.1038/s41467-017-00965-y
- Zviran A, Schulman RC, Shah M, et al. Genome-wide cell-free DNA mutational integration enables ultra-sensitive cancer monitoring. Nat Med. 2020;26(7):1114-1124. doi: 10.1038/s41591-020-0915-3
- Snyder MW, Kircher M, Hill AJ, Daza RM, Shendure J. Cell-free DNA comprises an in vivo nucleosome footprint that informs its tissues-of-origin. Cell. 2016;164(1-2):57-68. doi: 10.1016/j.cell.2015.11.050
- Mouliere F, Chandrananda D, Piskorz AM, et al. Enhanced detection of circulating tumor DNA by fragment size analysis. Sci Transl Med. 2018;10(466):eaat4921. doi: 10.1126/scitranslmed.aat4921
- Widman AJ, Shah M, Frydendahl A, et al. Ultrasensitive plasma-based monitoring of tumor burden using machine-learning-guided signal enrichment. Nat Med. 2024;30(6):1655-1666. doi: 10.1038/s41591-024-03040-4
- Kidess E, Jeffrey SS. Circulating tumor cells versus tumor-derived cell-free DNA: Rivals or partners in cancer care in the era of single-cell analysis? Genome Med. 2013;5(8):70. doi: 10.1186/gm474
- Franken B, De Groot MR, Mastboom WJ, et al. Circulating tumor cells, disease recurrence and survival in newly diagnosed breast cancer. Breast Cancer Res. 2012;14(5):R133. doi: 10.1186/bcr3333
- Poruk KE, Valero V, He J, et al. Circulating epithelial cells in intraductal papillary mucinous neoplasms and cystic pancreatic lesions. Pancreas. 2017;46(7):943-947. doi: 10.1097/MPA.0000000000000869
- Franses JW, Basar O, Kadayifci A, et al. Improved detection of circulating epithelial cells in patients with intraductal papillary mucinous neoplasms. Oncologist. 2018;23(1):121-127. doi: 10.1634/theoncologist.2017-0234
- Man Y, Wang Q, Kemmner W. Currently used markers for CTC isolation - advantages, limitations and impact on cancer prognosis. J Clin Exp Pathol. 2011;1(1):102. doi: 10.4172/2161-0681.1000102
- Alix-Panabières C, Pantel K. Liquid biopsy: From discovery to clinical application. Cancer Discov. 2021;11(4):858-873. doi: 10.1158/2159-8290.CD-20-1311
- Ni X, Zhuo M, Su Z, et al. Reproducible copy number variation patterns among single circulating tumor cells of lung cancer patients. Proc Natl Acad Sci. 2013;110(52):21083-21088. doi: 10.1073/pnas.1320659110
- Court CM, Hou S, Liu L, et al. Somatic copy number profiling from hepatocellular carcinoma circulating tumor cells. NPJ Precis Oncol. 2020;4(1):16. doi: 10.1038/s41698-020-0123-0
- Silvestri M, Dugo M, Vismara M, et al. Copy number alterations analysis of primary tumor tissue and circulating tumor cells from patients with early-stage triple negative breast cancer. Sci Rep. 2022;12(1):1470. doi: 10.1038/s41598-022-05502-6
- Baslan T, Kendall J, Rodgers L, et al. Genome-wide copy number analysis of single cells. Nat Protoc. 2012;7(6):1024-1041. doi: 10.1038/nprot.2012.039
- Ross MG, Russ C, Costello M, et al. Characterizing and measuring bias in sequence data. Genome Biol. 2013;14(5):R51. doi: 10.1186/gb-2013-14-5-r51
- Lasken RS, Stockwell TB. Mechanism of chimera formation during the Multiple Displacement Amplification reaction. BMC Biotechnol. 2007;7(1):19. doi: 10.1186/1472-6750-7-19
- Salter SJ, Cox MJ, Turek EM, et al. Reagent and laboratory contamination can critically impact sequence-based microbiome analyses. BMC Biol. 2014;12(1):87. doi: 10.1186/s12915-014-0087-z
- Merchant S, Wood DE, Salzberg SL. Unexpected cross-species contamination in genome sequencing projects. PeerJ. 2014;2:e675. doi: 10.7717/peerj.675
- Wood DE, Lu J, Langmead B. Improved metagenomic analysis with Kraken 2. Genome Biol. 2019;20(1):257. doi: 10.1186/s13059-019-1891-0
- Lu J, Breitwieser FP, Thielen P, Salzberg SL. Bracken: Estimating species abundance in metagenomics data. PeerJ Comput Sci. 2017;3:e104. doi: 10.7717/peerj-cs.104
- Wingett SW, Andrews S. FastQ screen: A tool for multi-genome mapping and quality control [version 2; peer review: 4 approved] F1000Res. 2018;7:1338. doi: 10.12688/f1000research.15931.2
- Fu A, Yao B, Dong T, et al. Tumor-resident intracellular microbiota promotes metastatic colonization in breast cancer. Cell. 2022;185(8):1356-1372.e26. doi: 10.1016/j.cell.2022.02.027
- Shen JJ, Zhang NR. Change-point model on nonhomogeneous Poisson processes with application in copy number profiling by next-generation DNA sequencing. Ann Appl Stat. 2012;6(2):476-496. doi: 10.1214/11-AOAS517
- Chen H, Bell JM, Zavala NA, Ji HP, Zhang NR. Allele-specific copy number profiling by next-generation DNA sequencing. Nucleic Acids Res. 2015;43(4):e23-e23. doi: 10.1093/nar/gku1252
- Garvin T, Aboukhalil R, Kendall J, et al. Interactive analysis and assessment of single-cell copy-number variations. Nat Methods. 2015;12(11):1058-1060. doi: 10.1038/nmeth.3578
- Wang X, Chen H, Zhang NR. DNA copy number profiling using single-cell sequencing. Brief Bioinform. 2018;19(5):731-736. doi: 10.1093/bib/bbx004
- Talevich E, Shain AH, Botton T, Bastian BC. CNVkit: Genome-wide copy number detection and visualization from targeted DNA sequencing. PLoS Comput Biol. 2016;12(4):e1004873. doi: 10.1371/journal.pcbi.1004873
- Van Loo P, Nordgard SH, Lingjærde OC, et al. Allele-specific copy number analysis of tumors. Proc Natl Acad Sci. 2010;107(39):16910-16915. doi: 10.1073/pnas.1009843107
- Oulhen M, Pawlikowska P, Tayoun T, et al. Circulating tumor cell copy-number heterogeneity in ALK-rearranged non-small-cell lung cancer resistant to ALK inhibitors. NPJ Precis Oncol. 2021;5(1):67. doi: 10.1038/s41698-021-00203-1
- Liu X, Li J, Cadilha BL, et al. Epithelial-type systemic breast carcinoma cells with a restricted mesenchymal transition are a major source of metastasis. Sci Adv. 2019;5(6):eaav4275. doi: 10.1126/sciadv.aav4275
- Pailler E, Faugeroux V, Oulhen M, et al. Acquired resistance mutations to ALK inhibitors identified by single circulating tumor cell sequencing in ALK -rearranged non-small-cell lung cancer. Clin Cancer Res. 2019;25(22):6671-6682. doi: 10.1158/1078-0432.CCR-19-1176
- Fu Y, Zhang F, Zhang X, et al. High-throughput single-cell whole-genome amplification through centrifugal emulsification and eMDA. Commun Biol. 2019;2(1):147. doi: 10.1038/s42003-019-0401-y
- Kuipers J, Tuncel MA, Ferreira P, Jahn K, Beerenwinkel N. Single-cell copy number calling and event history reconstruction. Cancer Biol. 2020. doi: 10.1101/2020.04.28.065755
- Bakker B, Taudt A, Belderbos ME, et al. Single-cell sequencing reveals karyotype heterogeneity in murine and human malignancies. Genome Biol. 2016;17(1):115. doi: 10.1186/s13059-016-0971-7
- Wang R, Lin DY, Jiang Y. SCOPE: A normalization and copy-number estimation method for single-cell DNA sequencing. Cell Syst. 2020;10(5):445-452.e6. doi: 10.1016/j.cels.2020.03.005
- Funnell T, O’Flanagan CH, Williams MJ, et al. Single-cell genomic variation induced by mutational processes in cancer. Nature. 2022;612(7938):106-115. doi: 10.1038/s41586-022-05249-0
- Peterson VM, Zhang KX, Kumar N, et al. Multiplexed quantification of proteins and transcripts in single cells. Nat Biotechnol. 2017;35(10):936-939. doi: 10.1038/nbt.3973
- Sinkala E, Sollier-Christen E, Renier C, et al. Profiling protein expression in circulating tumour cells using microfluidic western blotting. Nat Commun. 2017;8(1):14622. doi: 10.1038/ncomms14622
- Armbrecht L, Rutschmann O, Szczerba BM, Nikoloff J, Aceto N, Dittrich PS. Quantification of protein secretion from circulating tumor cells in microfluidic chambers. Adv Sci. 2020;7(11):1903237. doi: 10.1002/advs.201903237
- Macaulay IC, Haerty W, Kumar P, et al. G&T-seq: Parallel sequencing of single-cell genomes and transcriptomes. Nat Methods. 2015;12(6):519-522. doi: 10.1038/nmeth.3370
- Cheng YH, Chen YC, Lin E, et al. Hydro-Seq enables contamination-free high-throughput single-cell RNA-sequencing for circulating tumor cells. Nat Commun. 2019;10(1):2163. doi: 10.1038/s41467-019-10122-2
- Pixberg C, Schulz W, Stoecklein N, Neves R. Characterization of DNA methylation in circulating tumor cells. Genes. 2015;6(4):1053-1075. doi: 10.3390/genes6041053
- Gkountela S, Castro-Giner F, Szczerba BM, et al. Circulating tumor cell clustering shapes DNA methylation to enable metastasis seeding. Cell. 2019;176(1-2):98-112.e14. doi: 10.1016/j.cell.2018.11.046
- Chimonidou M, Strati A, Tzitzira A, et al. DNA methylation of tumor suppressor and metastasis suppressor genes in circulating tumor cells. Clin Chem. 2011;57(8):1169-1177. doi: 10.1373/clinchem.2011.165902
- Peneder P, Stütz AM, Surdez D, et al. Multimodal analysis of cell-free DNA whole-genome sequencing for pediatric cancers with low mutational burden. Nat Commun. 2021;12(1):3230. doi: 10.1038/s41467-021-23445-w
- Cristiano S, Leal A, Phallen J, et al. Genome-wide cell-free DNA fragmentation in patients with cancer. Nature. 2019;570(7761):385-389. doi: 10.1038/s41586-019-1272-6
- Ulz P, Perakis S, Zhou Q, et al. Inference of transcription factor binding from cell-free DNA enables tumor subtype prediction and early detection. Nat Commun. 2019;10(1):4666. doi: 10.1038/s41467-019-12714-4
- Wong D, Luo P, Oldfield LE, et al. Early cancer detection in Li-Fraumeni syndrome with cell-free DNA. Cancer Discov. 2024;14(1):104-119. doi: 10.1158/2159-8290.CD-23-0456
- Grabuschnig S, Soh J, Heidinger P, et al. Circulating cell-free DNA is predominantly composed of retrotransposable elements and non-telomeric satellite DNA. J Biotechnol. 2020;313:48-56. doi: 10.1016/j.jbiotec.2020.03.002
- Papasotiriou I, Pantopikou K, Apostolou P. L1 retrotransposon expression in circulating tumor cells. PLoS One. 2017;12(2):e0171466. doi: 10.1371/journal.pone.0171466
- Ponomaryova AA, Rykova EY, Gervas PA, Cherdyntseva NV, Mamedov IZ, Azhikina TL. Aberrant methylation of LINE-1 transposable elements: A search for cancer biomarkers. Cells. 2020;9(9):2017. doi: 10.3390/cells9092017
- Su SF, De Castro Abreu AL, Chihara Y, et al. A panel of three markers hyper- and hypomethylated in urine sediments accurately predicts bladder cancer recurrence. Clin Cancer Res. 2014;20(7):1978-1989. doi: 10.1158/1078-0432.CCR-13-2637
- Ross JP, Rand KN, Molloy PL. Hypomethylation of repeated DNA sequences in cancer. Epigenomics. 2010;2(2):245-269. doi: 10.2217/epi.10.2
- Burns KH. Transposable elements in cancer. Nat Rev Cancer. 2017;17(7):415-424. doi: 10.1038/nrc.2017.35
- Annapragada AV, Niknafs N, White JR, et al. Genome-wide repeat landscapes in cancer and cell-free DNA. Sci Transl Med. 2024;16(738):eadj9283. doi: 10.1126/scitranslmed.adj9283
- Park MK, Lee JC, Lee JW, Hwang SJ. Alu cell-free DNA concentration, Alu index, and LINE-1 hypomethylation as a cancer predictor. Clin Biochem. 2021;94:67-73. doi: 10.1016/j.clinbiochem.2021.04.021
- Douville C, Lahouel K, Kuo A, et al. Machine learning to detect the SINEs of cancer. Sci Transl Med. 2024;16(731):eadi3883. doi: 10.1126/scitranslmed.adi3883
- Reggiardo RE, Maroli SV, Peddu V, et al. Profiling of repetitive RNA sequences in the blood plasma of patients with cancer. Nat Biomed Eng. 2023;7(12):1627-1635. doi: 10.1038/s41551-023-01081-7
- Aceto N, Bardia A, Wittner BS, et al. AR expression in breast cancer CTCs associates with bone metastases. Mol Cancer Res. 2018;16(4):720-727. doi: 10.1158/1541-7786.MCR-17-0480
- Chen C, Mahalingam D, Osmulski P, et al. Single‐cell analysis of circulating tumor cells identifies cumulative expression patterns of EMT‐related genes in metastatic prostate cancer. Prostate. 2013;73(8):813-826. doi: 10.1002/pros.22625
- D’Avola D, Villacorta-Martin C, Martins-Filho SN, et al. High-density single cell mRNA sequencing to characterize circulating tumor cells in hepatocellular carcinoma. Sci Rep. 2018;8(1):11570. doi: 10.1038/s41598-018-30047-y
- Papadaki MA, Stoupis G, Theodoropoulos PA, Mavroudis D, Georgoulias V, Agelaki S. Circulating tumor cells with stemness and epithelial-to-mesenchymal transition features are chemoresistant and predictive of poor outcome in metastatic breast cancer. Mol Cancer Ther. 2019;18(2):437-447. doi: 10.1158/1535-7163.MCT-18-0584
- Negishi R, Yamakawa H, Kobayashi T, et al. Transcriptomic profiling of single circulating tumor cells provides insight into human metastatic gastric cancer. Commun Biol. 2022;5(1):20. doi: 10.1038/s42003-021-02937-x
- Ring A, Mineyev N, Zhu W, et al. EpCAM based capture detects and recovers circulating tumor cells from all subtypes of breast cancer except claudin-low. Oncotarget. 2015;6(42):44623-44634. doi: 10.18632/oncotarget.5977
- Wolf FA, Angerer P, Theis FJ. SCANPY: Large-scale single-cell gene expression data analysis. Genome Biol. 2018;19(1):15. doi: 10.1186/s13059-017-1382-0
- Satija R, Farrell JA, Gennert D, Schier AF, Regev A. Spatial reconstruction of single-cell gene expression data. Nat Biotechnol. 2015;33(5):495-502. doi: 10.1038/nbt.3192
- Serin Harmanci A, Harmanci AO, Zhou X. CaSpER identifies and visualizes CNV events by integrative analysis of single-cell or bulk RNA-sequencing data. Nat Commun. 2020;11(1):89. doi: 10.1038/s41467-019-13779-x
- Bařinka J, Hu Z, Wang L, et al. RNAseqCNV: Analysis of large-scale copy number variations from RNA-seq data. Leukemia. 2022;36(6):1492-1498. doi: 10.1038/s41375-022-01547-8
- Gao R, Bai S, Henderson YC, et al. Delineating copy number and clonal substructure in human tumors from single-cell transcriptomes. Nat Biotechnol. 2021;39(5):599-608. doi: 10.1038/s41587-020-00795-2
- Hou Y, Guo H, Cao C, et al. Single-cell triple omics sequencing reveals genetic, epigenetic, and transcriptomic heterogeneity in hepatocellular carcinomas. Cell Res. 2016;26(3):304-319. doi: 10.1038/cr.2016.23
- Han KY, Kim KT, Joung JG, et al. SIDR: Simultaneous isolation and parallel sequencing of genomic DNA and total RNA from single cells. Genome Res. 2018;28(1):75-87. doi: 10.1101/gr.223263.117
- Rodriguez-Meira A, Buck G, Clark SA, et al. Unravelling intratumoral heterogeneity through high-sensitivity single-cell mutational analysis and parallel RNA sequencing. Mol Cell. 2019;73(6):1292-1305.e8. doi: 10.1016/j.molcel.2019.01.009
- Cortés-López M, Chamely P, Hawkins AG, et al. Single-cell multi-omics defines the cell-type-specific impact of splicing aberrations in human hematopoietic clonal outgrowths. Cell Stem Cell. 2023;30(9): 1262-1281.
- Belhocine K, DeMare L, Habern O. Single-cell multiomics: Simultaneous epigenetic and transcriptional profiling: 10x Genomics shares experimental planning and sample preparation tips for the chromium single cell multiome ATAC + gene expression system. Genet Eng Biotechnol News. 2021;41(1):66-68. doi: 10.1089/gen.41.01.17
- Zhong W, Gummesson A, Tebani A, et al. Whole-genome sequence association analysis of blood proteins in a longitudinal wellness cohort. Genome Med. 2020;12(1):53. doi: 10.1186/s13073-020-00755-0
- Enroth S, Johansson Å, Enroth SB, Gyllensten U. Strong effects of genetic and lifestyle factors on biomarker variation and use of personalized cutoffs. Nat Commun. 2014;5(1):4684. doi: 10.1038/ncomms5684
- Ding Z, Wang N, Ji N, Chen ZS. Proteomics technologies for cancer liquid biopsies. Mol Cancer. 2022;21(1):53. doi: 10.1186/s12943-022-01526-8
- Landegren U, Hammond M. Cancer diagnostics based on plasma protein biomarkers: Hard times but great expectations. Mol Oncol. 2021;15(6):1715-1726. doi: 10.1002/1878-0261.12809
- Feng W, Beer JC, Hao Q, et al. NULISA: A proteomic liquid biopsy platform with attomolar sensitivity and high multiplexing. Nat Commun. 2023;14(1):7238. doi: 10.1038/s41467-023-42834-x
- Wolf J, Rasmussen DK, Sun YJ, et al. Liquid-biopsy proteomics combined with AI identifies cellular drivers of eye aging and disease in vivo. Cell. 2023;186(22):4868-4884.e12. doi: 10.1016/j.cell.2023.09.012
- Zhao T, Chiang ZD, Morriss JW, et al. Spatial genomics enables multi-modal study of clonal heterogeneity in tissues. Nature. 2022;601(7891):85-91. doi: 10.1038/s41586-021-04217-4
- He S, Bhatt R, Brown C, et al. High-plex imaging of RNA and proteins at subcellular resolution in fixed tissue by spatial molecular imaging. Nat Biotechnol. 2022;40(12):1794-1806. doi: 10.1038/s41587-022-01483-z
- Zhang D, Deng Y, Kukanja P, et al. Spatial epigenome-transcriptome co-profiling of mammalian tissues. Nature. 2023;616(7955):113-122. doi: 10.1038/s41586-023-05795-1
- Van TM, Blank CU. A user’s perspective on GeoMxTM digital spatial profiling. Immunooncol Technol. 2019;1:11-18. doi: 10.1016/j.iotech.2019.05.001
- Zeune LL, De Wit S, Berghuis AMS, IJzerman MJ, Terstappen LWMM, Brune C. How to agree on a CTC: Evaluating the consensus in circulating tumor cell scoring. Cytometry A. 2018;93(12):1202-1206. doi: 10.1002/cyto.a.23576
- Nanou A, Stoecklein NH, Doerr D, Driemel C, Terstappen LWMM, Coumans FAW. Training an automated circulating tumor cell classifier when the true classification is uncertain. PNAS Nexus. 2024;3(2):pgae048. doi: 10.1093/pnasnexus/pgae048
- Svensson CM, Hübler R, Figge MT. Automated classification of circulating tumor cells and the impact of interobsever variability on classifier training and performance. J Immunol Res. 2015;2015:573165. doi: 10.1155/2015/573165
- Lannin TB, Thege FI, Kirby BJ. Comparison and optimization of machine learning methods for automated classification of circulating tumor cells. Cytometry A. 2016;89(10):922-931. doi: 10.1002/cyto.a.22993
- Raub CB, Nehmetallah G. Holography, machine learning, and cancer cells. Cytometry A. 2017;91(8):754-756. doi: 10.1002/cyto.a.23112
- Ko J, Baldassano SN, Loh PL, Kording K, Litt B, Issadore D. Machine learning to detect signatures of disease in liquid biopsies - a user’s guide. Lab Chip. 2018;18(3):395-405. doi: 10.1039/C7LC00955K
- Pirone D, Montella A, Sirico DG, et al. Label-free liquid biopsy through the identification of tumor cells by machine learning-powered tomographic phase imaging flow cytometry. Sci Rep. 2023;13(1):6042. doi: 10.1038/s41598-023-32110-9
- Rieke N, Hancox J, Li W, et al. The future of digital health with federated learning. NPJ Digit Med. 2020;3(1):119. doi: 10.1038/s41746-020-00323-1
- Sohn E. The reproducibility issues that haunt health-care AI. Nature. 2023;613(7943):402-403. doi: 10.1038/d41586-023-00023-2
- Ravi N, Chaturvedi P, Huerta EA, et al. FAIR principles for AI models with a practical application for accelerated high energy diffraction microscopy. Sci Data. 2022;9(1):657. doi: 10.1038/s41597-022-01712-9
- Mignan A, Broccardo M. One neuron versus deep learning in aftershock prediction. Nature. 2019;574(7776):E1-E3. doi: 10.1038/s41586-019-1582-8
- Laubenbacher R, Niarakis A, Helikar T, et al. Building digital twins of the human immune system: Toward a roadmap. NPJ Digit Med. 2022;5(1):64. doi: 10.1038/s41746-022-00610-z
- Popa EO, Van Hilten M, Oosterkamp E, Bogaardt MJ. The use of digital twins in healthcare: Socio-ethical benefits and socio-ethical risks. Life Sci Soc Policy. 2021;17(1):6. doi: 10.1186/s40504-021-00113-x
- Goodwin S, McPherson JD, McCombie WR. Coming of age: Ten years of next-generation sequencing technologies. Nat Rev Genet. 2016;17(6):333-351. doi: 10.1038/nrg.2016.49
- Siravegna G, Marsoni S, Siena S, Bardelli A. Integrating liquid biopsies into the management of cancer. Nat Rev Clin Oncol. 2017;14(9):531-548. doi: 10.1038/nrclinonc.2017.14
- Moser T, Kühberger S, Lazzeri I, Vlachos G, Heitzer E. Bridging biological cfDNA features and machine learning approaches. Trends Genet. 2023;39(4):285-307. doi: 10.1016/j.tig.2023.01.004
- Han Y, Li X, Zhang M, et al. Enhanced detection of genitourinary cancers using fragmentation and copy number profiles obtained from urinary cell-free DNA. Clin Chem. 2021;67(2):394-403. doi: 10.1093/clinchem/hvaa283
- Ge G, Peng D, Guan B, et al. Urothelial carcinoma detection based on copy number profiles of urinary cell-free DNA by shallow whole-genome sequencing. Clin Chem. 2020;66(1):188-198. doi: 10.1373/clinchem.2019.309633
- Zeune LL, Boink YE, Van Dalum G, et al. Deep learning of circulating tumour cells. Nat Mach Intell. 2020;2(2):124-133. doi: 10.1038/s42256-020-0153-x
- Fernandez-Garcia D, Nteliopoulos G, Hastings RK, et al. Shallow WGS of individual CTCs identifies actionable targets for informing treatment decisions in metastatic breast cancer. Br J Cancer. 2022;127(10):1858-1864. doi: 10.1038/s41416-022-01962-9
- Chen JY, Chou HH, Lim SC, et al. Multiomic characterization and drug testing establish circulating tumor cells as an ex vivo tool for personalized medicine. iScience. 2022;25(10):105081. doi: 10.1016/j.isci.2022.105081
- Dong X, Zhang L, Hao X, Wang T, Vijg J. SCCNV: A software tool for identifying copy number variation from single-cell whole-genome sequencing. Front Genet. 2020;11:505441. doi: 10.3389/fgene.2020.505441
- Zaccaria S, Raphael BJ. Characterizing allele- and haplotype-specific copy numbers in single cells with CHISEL. Nat Biotechnol. 2021;39(2):207-214. doi: 10.1038/s41587-020-0661-6
- Favero F, Joshi T, Marquard AM, et al. Sequenza: Allele-specific copy number and mutation profiles from tumor sequencing data. Ann Oncol. 2015;26(1):64-70. doi: 10.1093/annonc/mdu479
- Boeva V, Popova T, Bleakley K, et al. Control-FREEC: A tool for assessing copy number and allelic content using next-generation sequencing data. Bioinformatics. 2012;28(3):423-425. doi: 10.1093/bioinformatics/btr670
- Gao T, Soldatov R, Sarkar H, et al. Haplotype-aware analysis of somatic copy number variations from single-cell transcriptomes. Nat Biotechnol. 2023;41(3):417-426. doi: 10.1038/s41587-022-01468-y