Are random price generators useful for health policy processes and analysis? effectiveness of random price generators in health policy processes and analysis
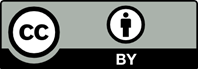
This paper discusses why using random price generators in an economic model for medical markets may be useful. It summarizes the different steps for the development of the model and describes an original contribution of the physicians’ choice model with random drug prices applied to Type 2 diabetes in the US. Pharmaceutical markets have been supply-driven to boost life science and medical technologies; however, with the widening inequalities inside national health systems and the global agenda for universal health coverage, more economic research is being done to strengthen the analysis of demand for healthcare services. Research, especially, examines more disaggregated levels of demand systems to understand the heterogeneity of physicians’ choices and better capture patient needs. It can also be an approach to calibrate supply and demand adjustments in medical markets. This paper argues that choice modeling is particularly relevant, using random price generators in a structural model where a demand approach is useful. If validated by additional experimental studies, this first study by Professors Huttin & Hausman (2021) could be used in advanced value assessment frameworks. Random price generators on medical markets could also be tested with additional models that fit oligopolistic market structures (e.g., models for differentiated product markets such as the Berry–Levinsohn–Pakes model). It may also help with a policy analysis process that addresses major disruptive transformations of market dynamics, evolving boundaries in science, fast digitalization, and artificial intelligence-based information systems.
Abaluck, J., & Gruber, J. (2013). Evolving Choice Inconsistencies in Choice of Prescription Drug Insurance, June, WP 19163. United States: NBER.
Berry, S. (1994). Estimating discrete-choice models of product differentiation. Rand Journal of Economics, 25(2):242-262. https://doi.org/10.2307/2555829
Berry, S., Levinsohn, J., & Pakes, A. (1995). Automobile prices in market equilibrium. Econometrica, 63(4):841-890. https://doi.org/10.2307/2171802
Berry, S.T., & Haile, P.A. (2014). Identification in differentiated product markets using market level data. Econometrica, 82(5):1749-1797. https://doi.org/10.3982/ECTA9027
Burda, M., Harding, M., & Hausman, J.A. (2012). Bayesian mixed logit-probit model for multinomial choice. Journal of Econometrics, 147:232-246.
Chen, Y., Pan, Y., & Ding, Y. (2023). How does market competition affect supplier-induced demand? An experimental study. Frontier in Public Health, 11:1024337. https://doi.org/10.3389/fpubh.2023.1024337
Colon, C., & Gortmaker, J. (2020). Best practices for differentiated products demand estimation with PyBLP. Rand Journal of Economics, 51(4):1108-1161.
Colorni, A., & Tsoukias, A. (2018). In: Matsatsinis, N., & Grigoroudis, E. (eds.). General Framework for Designing Alternatives. Berlin: Springer, p.1-15. https://doi.org:10.1007/978-3-319-90599-0-1
Ellis, A., De Bekker-Grob, E., Howard, K., Thomas, K., Lancsar, E., Ryan, M., et al. (2019). Number of Halton Draws Required for Valid Random Parameter Estimation with Discrete Choice Data. In: Proceedings, IAHPR Conference.
Evans, R. (1974). Supplier-Induced Demand. Some Empirical Evidence and Implications. London: McMillan.
Glenk, K., Johnston, R., Meyerhoff, J., & Sagebiel, J. (2020). Valuation in environmental and resource economics. Methods, Trends and Challenges, 75:215-242. https://doi.org/10.1007/s10640-018-00311-w
Grimmett, G.R., & Stirzaker, D.R. (2020), Probability and Random Processes. 4th ed. Oxford: Oxford University Press.
Hahn, J., Hausman, J., & Lustig, J. (2020). Specification test on mixed logit models. Journal of Econometrics, 219:19-37. https://doi.org/10.1016/j.jeconom.2020.03.015
Hatchuel, A., Weil, B., & Le Masson, P. (2012). Towards an ontology of design: Lessons from C-K design theory and forcing. Research in Engineering Design, 24(2):147-163.
Hauber, A.B., González, J.M., Groothuis-Oudshoorn, C.G., Prior, T., Marshall, D.A., Cunningham, C., et al. (2016). Statistical methods for the analysis of discrete choice experiments. A report of the ISPOR conjoint analysis good research practices task force. Value in Health, 19:300-315. https://doi.org/10.1016/j.jval.2016.04.004
Hausman, J., & McFadden, D. (1984). Specification tests for the multinomial logit model. Econometrica, 52(5):1219-1240.
Huttin, C.C. (2012). Mobile economics and pricing of healthcare services. Technology and Health Care, 20:459-461. https://doi.org/10.3233/THC-2012-0693
Huttin, C.C. (2015). Perspectives of biologists, epidemiologists and geneticists’ controversies in science and health system reforms. Technology and Health Care, 23(1):103-108.
Huttin, C.C. (2017). Stated and revealed preference issues-products, patients and physicians’ attributes in choice experiments. Technology and Health Care, 25(5):1005-1020.
Huttin, C.C., & Hausman, J. (2021). Development of a physicians ‘choice model using mixed logit with random prices for drugs case study on diabetes TYPE II. Archives of Health Sciences, 5(1):1-10. https://doi.org/10.21203/rs.3.rs-124401/v1
Kurzweil, R. (2005). The Singularity is Near. When Humans Transcend Biology. New York: Penguin Books.
Mariel, P., & Meyerhoff, J. (2018). A more flexible model or simply more effort? On the use of correlated Random Parameters in applied choice studies. Ecological Economics, 154:419-429.
Park, S., & Gupta, S. (2012). Comparison of SML and GMM estimators for the Random coefficient logit model using aggregate data. Empirical Economics, 43:1353-1372. https://doi.org/10.1007/s00181-011-0519-3
Sina Shih, Y.C., & Tai-Seale, M. (2012). Physicians ‘perceptions of demand-induced supply in the information age: A latent class model. Health Economics, 21(3):252-269. https://doi.org/10.1002/hec.1710
Soekhai, V., De Bekker-Grob, E., Ellis, A., & Vass, C.M. (2019). Discrete choice experiments in health economics: Past, present and future. Pharmacoeconomics, 37(2):201-226. https://doi.org/10.1007/s40273-018-0734-2
Solow, B., & Pezalla, E. (2018). ISPOR’s initiative on US value assessment frameworks: The use of cost effectiveness research in decision making among US insurers. Value in Health, 21:166-168. https://doi.org/10.1016/j.jval.2017.12.004
Sunstein, C.R. (2016). The Ethics of Influence: Government in the Age of Behavioral Science. Cambridge: Cambridge University Press.
Sunstein, C.R. (2019). How Change Happens. United States: MIT Press.
United Nation. (2003). Integrated Environmental and Economic Accounting, internal Documents on Pricing Taxonomies. UN: United States.
Vass, C., Boeri, M., Karim, S., Marshall, D., Craig, B., Ho, K., et al. (2022). Accounting for Preference heterogeneity in discrete-choice experiments: A ispor special interest group report. Value in Health, 25(5):685-694. https://doi.org/10.1016/j.jval.2022.01.012
Zhou, M., & Bridges, J.F. (2019). Explore preference heterogeneity for treatment among people with Type 2 diabetes: A comparison of random-parameters and latent class estimation techniques. Journal of Choice Modelling, 30:38-49. https://doi.org/10.1016/j.jocm.2018.11.002