Artificial intelligence-enabled antibiotic prescribing and clinical support in Nigerian health-care settings: Budgetary constraints, challenges, and prospect
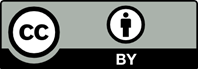
Today, resistance developed by bacteria to common antibiotics that were otherwise regarded as effective is posing a serious challenge. It is believed that without any different efforts, this perennial problem will undermine all the ongoing efforts in antibiotic discovery and therapy development. In Nigeria, antibiotics are frequently prescribed in hospitals. However, issues like multidrug resistance (MDR) and inappropriate use and misuse of antibiotics, including incorrect dosages and use of broad-spectrum antibiotics for targeted infections, have precipitated the rise of MDR bacteria. Consequently, this leads to higher healthcare costs, mainly due to prolonged hospital stays and additional medications as well as increased patient mortality. The prospects of artificial intelligence (AI)-enabled antibiotic prescribing hold significant promise in transforming the current health-care practices. AI has the potential to enhance the precision and efficiency of antibiotic treatment through advanced algorithms and data analytics. This technology can contribute to improved diagnostic accuracy, providing real-time clinical support, optimizing dosage recommendations, personalized treatment plans, and streamlined antimicrobial stewardship, ultimately aiding the global fight against antibiotic resistance and optimizing patient outcomes. The integration of AI in antibiotic prescribing reflects a cutting-edge approach with the potential to revolutionize how antibiotics are prescribed to address challenges in antimicrobial stewardship, clinical decision-making, and combating antibiotic resistance. One of the key impediments to integrating AI into Nigeria’s health-care system is budgetary constraints. Addressing these constraints through strategic investments, improved budgetary allocation to research and development, and leveraging the opportunities presented by AI technologies can significantly enhance antibiotic prescribing and health-care practices, leading to improved public health outcomes.
Ali, A.R., Alhumaid, S., Al Mutair, A., Garout, M., Abulhamayel, Y., Halwani, M.A., et al. (2022). Application of artificial intelligence in combating high antimicrobial resistance rates. Antibiotics (Basel), 11(6):784. https://doi.org/10.3390/antibiotics11060784
Anon. (2020). Alliance for Responsible Use of Antibiotics (ARUA). Community Engagement toolkit for Antimicrobial Resistance. Anon. (2021). Global Fund to Fight AIDS, Tuberculosis and Malaria. Investment Case for Antimicrobial Resistance. Available from: https://www.theglobalfund.org/en/tuberculosis [Last accessed on 2023 Nov 14].
Bajwa, J., Munir, U., Nori, A., & Williams, B. (2021). Artificial intelligence in healthcare: Transforming the practice of medicine. Future Healthcare Journal, 8(2):e188-e194. https://doi.org/10.7861/fhj.2021-0095
Baker, R.E., Mahmud, A.S., Miller, I.F., Rajeev, M., Rasambainarivo, F., Rice, B.L., et al. (2022). Infectious disease in an era of global change. Nature Reviews Microbiology, 20(4):193-205. https://doi.org/10.1038/s41579-021-00639-z
Brownstein, J.S., Rader, B., Astley, C.M., & Tian, H. (2023). Advances in artificial intelligence for infectious disease surveillance. New England Journal of Medicine, 388(17):1597-1607. https://doi.org/10.1056/NEJMra2119215
Cavallaro, M., Moran, E., Collyer, B., McCarthy, N.D., Green, C., & Keeling, M.J. (2023). Informing antimicrobial stewardship with explainable AI. PLOS Digital Health, 2(1):e0000162. https://doi.org/10.1371/journal.pdig.0000162
Chang, A., & Chen, J.H. (2022). BSAC Vanguard Series: Artificial intelligence and antibiotic stewardship. Journal of Antimicrobial Chemotherapy, 77(5):1216-1217. https://doi.org/10.1093/jac/dkac096
Christaki, E. (2015). New technologies in predicting, preventing, and controlling emerging infectious diseases. Virulence, 6(6):558-565. https://doi.org/10.1080/21505594.2015.1040975
European Centre for Disease Prevention and Control (ECDPC). (2021). Digital technologies for the surveillance, prevention and control of infectious diseases-a scoping review of the research literature. Sweden: European Centre for Disease Prevention and Control (ECDPC).
Fanelli, U., Pappalardo, M., Chinè, V., Gismondi, P., Neglia, C., Argentiero, A., et al. (2020). Role of artificial intelligence in fighting antimicrobial resistance in pediatrics. Antibiotics, 9(11):767. https://doi.org/10.3390/antibiotics9110767
GAO. (2020). Artificial Intelligence in Health Care: Benefits and Challenges of Technologies to Augment Patient Care. In: Report to Congressional Requesters Technology Assessment. United States: GAO, pp.13-29.
Gille, F., Smith, S., & Mays, N. (2015). Why public trust in healthcare systems matters and deserves greater research attention. Journal of Health Services Research and Policy, 20(1):62-64. https://doi.org/10.1177/1355819614543161
Goldberg, C.B., Adams, L., Blumenthal, D., Brennan, P.F., Brown, N., Butte, A.J., et al. (2024). To do no harm-and the most good-with AI in health care. Nature Medicine, 30(3):623-627. https://doi.org/10.1038/s41591-024-02853-7
International Business Machines (IBM). (2023). IBM’s Watson AI Saves Woman’s Life by Diagnosing Rare Form of Leukaemia. Available from: Available from: https://www.huffingtonpost.co.uk [Last accessed on 2024 Feb 11].
International Development Research Centre. (2024). Artificial Intelligence for Development (AI4D) Africa. Available from: https://www.idrc.ca/en/project/artificialintelligence-development-ai4d-africa​:citation[oaicite:1]{index=1}​​:citation[oaicite:0]{index=0}​ [Last accessed on 2023 Dec 11].
Jaafaru, I.A., Rabiu, I., Idris, K.B., & Abdulfatai, K. (2022). Determination of inducible clindamycin resistance amongst clinical isolates of methicillin-resistant Staphylococcus aureus in Kaduna, Nigeria. Journal of Advances in Microbiology, 22(1):32-38.
Jiménez-Luna, J., Grisoni, F., Weskamp, N., & Schneider, G. (2021). Artificial intelligence in drug discovery: Recent advances and future perspectives. Expert Opinion on Drug Discovery, 16(9):949-959.https://doi.org/10.1080/17460441.2021.1909567
Kaplan, A.D., Kessler, T.T., Brill, J.C., & Hancock, P.A. (2023). Trust in artificial intelligence: Meta-analytic findings. Human Factors, 65(2):337-359. https://doi.org/10.1177/00187208211013988
Lau, H.J., Lim, C.H., Foo, S.C., & Tan, H.S. (2021). The role of artificial intelligence in the battle against antimicrobialresistant bacteria. Current Journal of Genetics, 67:421-429.
Marra, A.R., Langford, B.J., Nori, P., & Bearman, G. (2023). Revolutionizing antimicrobial stewardship, infection prevention, and public health with artificial intelligence: Themiddle path. Antimicrobial Stewardship and Healthcare Epidemiology, 3(1):e219. https://doi.org/10.1017/ash.2023.494
Okeowo, E., Afolabi, A., Adekambi, A., & Oyegbile, T. (2020). Effectiveness of antibiotic stewardship programs: The role of artificial intelligence. Journal of Antimicrobial Chemotherapy, 75(10):2657-2659.
Rabiu, I., Auwal, Z., & Muhammad, H.A. (2022a). Detection of carbapenemase producing enterobacteriaceae from clinical samples and their susceptibility to conventional antibiotics and medicinal plant extracts. Annals of Experimental and Molecular Biology, 4(1):000115.
Rabiu, I., Jamilu, Z., & Ahmad, F.R. (2023). Buruli ulcer (Mycobacterium ulcerans infection) in Nigeria: An update on the disease burden in Nigeria. Clinical and Medical Research and Studies, 2(1):1-3.
Rabiu, I., Yusha’u, M., & Abdullahi, A.M. (2022c). Antibacterial activity of Colocasia esculenta leaf extracts against multidrug resistant extended spectrum Β-lactamase producing Escherichia coli and Klebsiella pneumoniae. Bayero Journal of Pure and Applied Sciences, 13(1):7-12.
Rajpurkar, P., Chen, E., Banerjee, O., & Topol, E.J. (2022). AI in health and medicine. Nature Medicine, 28(1):31-38. https://doi.org/10.1038/s41591-021-01614-0
Rawayau, A.M., Kano1, A.M., Nuhu, I.A., Salisu, A., Imrana, I., Malami, M.A., et al. (2022). In vivo evaluation of antidiabetic effects of some polyherbal formulations in alloxan-induced diabetic wistar rats. Tropical Journal of Natural Product Research, 6(5):818-825. https://doi.org/10.26538/tjnpr/v6i5.26
Sahu, A., Mishra, J., & Kushwaha, N. (2022). Artificial intelligence (AI) in drugs and pharmaceuticals. Combinatorial Chemistry and High Throughput Screening, 25(11):1818-1837. https://doi.org/10.2174/1386207325666211207153943
Sarkar, C., Das, B., Rawat, V.S., Wahlang, J.B., Nongpiur, A., Tiewsoh, I., et al. (2023). Artificial intelligence and machine learning technology driven modern drug discovery and development. International Journal of Molecular Sciences, 24(3):2026. https://doi.org/10.3390/ijms24032026
Shitu, A.S., Mukhkar, M., Bukar, A., & Yusha’u, M. (2020). Extended spectrum beta-lactamase production, biofilm formation and antibiotic resistance in clinical isolates of Klebsiella pneumoniae. Nigerian Journal of Microbiology, 34(1):4874-4883.
Sulaiman, A., Singh, D., Muhammad, A. Abdullahi, U. Z., & Rabiu, I. (2022). Evaluation of serum level of minerals, alkaline phosphatase and vitamin D in healthy pregnant women of Jodhpur. International Journal of Scientific and Engineering Research, 13(2):797.
Tamma, P.D., Aitken, S.L., Bonomo, R.A., Mathers, A.J., Van Duin, D., & Clancy, C.J. (2023). Infectious Diseases Society of America 2023 guidance on the treatment of antimicrobial resistant gram-negative infections. Clinical Infectious Diseases:ciad428. https://doi.org/10.1093/cid/ciad428
Valderrama-Rios, M.C., Álvarez-Moreno, C.A., & Cortes, J.A. (2023). Interventions to improve antibiotic use in hospitals with different levels of complexity in Colombia: Findings from a before-and-after study and suggestions for the future. Antibiotics (Basel), 12(5):867. https://doi.org/10.3390/antibiotics12050867
Van Dort, B.A., Penm, J., Ritchie, A., & Baysari, M.T. (2022). The impact of digital interventions on antimicrobial stewardship in hospitals: A qualitative synthesis of systematic reviews. Journal of Antimicrobial Chemotherapy, 77(7):1828-1837. https://doi.org/10.1093/jac/dkac112
Vestesson, E., De Corte, K., Chappell, P., Crellin, E., & Clarke, G.M. (2023). Antibiotic prescribing in remote versus face-to-face consultations for acute respiratory infections in primary care in England: An observational study using target maximum likelihood estimation. EClinicalMedicine, 64:102245. https://doi.org/10.1016/j.eclinm.2023.102245
World Bank. (2017). Financing the Health Sector in Low-and Middle-Income Countries. Available from: https://pubdocs.worldbank.org/en/908481507403754670/annual-report-2017-wbg.pdf [Last accessed on 2024 Jan 20].
WorldHealthOrganization(WHO).(2015). MonitoringandEvaluating Implementation of the Global Action Plan for the Containment of Antimicrobial Resistance. Available from: https://www.bing.com/search?Q=world+health+organization+(who)%2c+2015.+monitoring+and+evaluating+implementation+of+the+global+action+plan+for+the+containment+of+antimicrobial+resistance.&cvid=0693873f64c043918b42b4851e98882c&gs_lcrp=egzjahjvbwuybggaeeuyodibbzy2ogowajsoagiwage&form=anab01&pc=astS [Last accessed on 2024 Jun 04].
World Health Organization (WHO). (2019a). Engaging Communities in Addressing Antimicrobial Resistance. Available from: https://www.who.int/publications/i/item/9789240010529 [Last accessed on 2024 Jun 04].
World Health Organization (WHO). (2019b). Global Strategy on Human Resources for Health: Workforce 2030. Available from: https://www.who.int/publications/i/item/9789241511131 [Last accessed on 2024 Jun 04].
Yusha’u, M., Aliyu, H., Kumurya, A., & Suleiman, K. (2010). Prevalence of extended spectrum β-lactamases (ESBLs) among Enterobacteriaceae in Murtala Mohammed specialist hospital, Kano, Nigeria. Bayero Journal of Pure and Applied Sciences, 3(1):169-172.