News and Announcements
LETTERS TO EDITOR
Artificial intelligence in radiation oncology
Show Less
1 Department of Radiation Oncology, Faculty of Medicine, Osmangazi University, Eskişehir,
Turkey
Received: 7 January 2025 | Accepted: 14 March 2025 | Published online: 27 March 2025
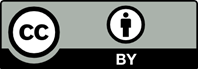
© 2025 by the Author(s). This article is an open access article distributed under the terms and conditions of the Creative Commons Attribution 4.0 International License ( https://creativecommons.org/licenses/by/4.0/ )
Conflict of interest
The author declares that she has no conflict of interest and has no competing interests.
References
- Lambin P, Rios-Velazquez E, Leijenaar R, et al. Radiomics: Extracting more information from medical images using advanced feature analysis. Eur J Cancer. 2012;48(4):441-446. doi: 10.1016/j.ejca.2011.11.036
- Jiang F, Jiang Y, Zhi H, et al. Artificial intelligence in healthcare: Past, present and future. Stroke Vasc Neurol. 2017;2(4):230-243. doi: 10.1136/svn-2017-000101
- Yakar M, Etiz D. Artificial intelligence in radiation oncology. Artif Intell Med Imaging. 2021;2(2):13-31. doi: 10.35711/aimi.v2.i2.13
- Huynh E, Hosny A, Guthier C, et al. Artificial intelligence in radiation oncology. Nat Rev Clin Oncol. 2020;17(12):771-781. doi: 10.1038/s41571-020-0417-8
- Yakar M, Etiz D, Metintas M, Ak G, Celik O. Prediction of radiation pneumonitis with machine learning in stage III lung cancer: A pilot study. Technol Cancer Res Treat. 2021;20:15330338211016373. doi: 10.1177/15330338211016373
- Akcay M, Etiz D, Celik O, Ozen A. Evaluation of acute hematological toxicity by machine learning in gynecologic cancers using postoperative radiotherapy. Indian J Cancer. 2022;59(2):178-186. doi: 10.4103/ijc.IJC_666_19
- Akcay M, Etiz D, Celik O, Ozen A. Evaluation of prognosis in nasopharyngeal cancer using machine learning. Technol Cancer Res Treat. 2020;19:1533033820909829. doi: 10.1177/1533033820909829
- Akcay M, Etiz D, Celik O. Prediction of survival and recurrence patterns by machine learning in gastric cancer cases undergoing radiation therapy and chemotherapy. Adv Radiat Oncol. 2020;5(6):1179-1187. doi: 10.1016/j.adro.2020.07.007
- Janssen S, El Shafie RA, Ruder AM, et al. Mobile applications in radiation oncology-current choices and future potentials. Strahlenther Onkol. 2023;199(4):337-349. doi: 10.1007/s00066-023-02048-y
- Bibault JE, Giraud P, Burgun A. Big Data and machine learning in radiation oncology: State of the art and future prospects. Cancer Lett. 2016;382(1):110-117. doi: 10.1016/j.canlet.2016.05.033
- Bibault JE, Giraud P. Deep learning for automated segmentation in radiotherapy: A narrative review. Br J Radiol. 2024;97(1153):13-20. doi: 10.1093/bjr/tqad018
- Samarasinghe G, Jameson M, Vinod S, et al. Deep learning for segmentation in radiation therapy planning: A review. J Med Imaging Radiat Oncol. 2021;65(5):578-595. doi: 10.1111/1754-9485.13286
- Liu X, Chen X, Chen D, et al. A patient-specific auto-planning method for MRI-guided adaptive radiotherapy in prostate cancer. Radiother Oncol. 2024;200:110525. doi: 10.1016/j.radonc.2024.110525
- Zeverino M, Piccolo C, Wuethrich D, et al. Clinical implementation of deep learning-based automated left breast simultaneous integrated boost radiotherapy treatment planning. Phys Imaging Radiat Oncol. 2023;28:100492. doi: 10.1016/j.phro.2023.100492
- Gichoya JW, Thomas K, Celi LA, et al. AI pitfalls and what not to do: Mitigating bias in AI. Br J Radiol. 2023;96(1150):20230023. doi: 10.1259/bjr.20230023
- Mittermaier M, Raza MM, Kvedar JC. Bias in AI-based models for medical applications: Challenges and mitigation strategies. NPJ Digit Med. 2023;6(1):113. doi: 10.1038/s41746-023-00858-z
- Naik N, Hameed BM, Shetty DK, et al. Legal and ethical consideration in artificial intelligence in healthcare: Who takes responsibility? Front Surg. 2022;9:862322. doi: 10.3389/fsurg.2022.862322