Emerging trends and future directions of machine learning in arthroplasty: A narrative review
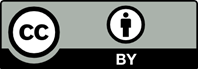
Artificial intelligence (AI) is rapidly transforming orthopedic surgery, particularly in total joint arthroplasty (TJA), offering new possibilities for improving patient outcomes. Thus, this narrative review examines the current applications and future directions of machine learning (ML) in hip, knee, and shoulder arthroplasty, focusing on predictive models for clinical outcomes, complications, and patient-reported outcome measures (PROMs). Preoperatively, ML algorithms have shown promise in identifying implants, predicting implant sizes, and assessing implant positioning on radiographs. In outcome prediction, ML models have been developed to predict PROMs, readmissions, length of stay, and healthcare costs associated with TJA. By analyzing large datasets to generate personalized predictions for patients, these models represent a novel approach to assist clinicians in individualized patient decision-making. Furthermore, AI has shown promise in predicting specific post-operative complications, such as dislocations, implant loosening, and prolonged opioid use, highlighting its value in improving surgical planning and patient management. Looking ahead, AI holds the potential to revolutionize orthopedic surgery by equipping clinicians with valuable tools to enhance decision-making and improve patient outcomes. However, the current efforts are shadowed by the challenges of transparency and validation of AI models. As AI continues to find utility in orthopedic clinics and operating rooms, efforts to enhance transparency and validate models will be crucial in realizing its full potential in orthopedic surgery.
- Helm JM, Swiergosz AM, Haeberle HS, et al. Machine learning and artificial intelligence: Definitions, applications, and future directions. Curr Rev Musculoskelet Med. 2020;13(1):69-76. doi: 10.1007/s12178-020-09600-8
- Patel AV, Stevens AJ, Mallory N, et al. Modern applications of machine learning in shoulder arthroplasty: A review. JBJS Rev. 2023;11(7): e22.00225. doi: 10.2106/JBJS.RVW.22.00225
- Sidey-Gibbons JAM, Sidey-Gibbons CJ. Machine learning in medicine: A practical introduction. BMC Med Res Methodol. 2019;19(1):64. doi: 10.1186/s12874-019-0681-4
- Halawi MJ. Outcome measures in total joint arthroplasty: Current status, challenges, and future directions. Orthopedics. 2015;38(8):e685-e689. doi: 10.3928/01477447-20150804-55
- Tokgöz E. Artificial intelligence, deep learning, and machine learning applications in total hip arthroplasty. In: Total Hip Arthroplasty. Berlin, Germany: Springer International Publishing; 2023. p. 231-246. doi: 10.1007/978-3-031-08927-5_11
- Hakwer, GA, Badley, EM, Borkhoff, CM, et al. Which patients are most likely to benefit from total joint arthroplasty? Arthritis Rheum. 2013;65(5):1243-1252. doi: 10.1002/art.37901
- Klemt C, Uzosike AC, Esposito JG, et al. The utility of machine learning algorithms for the prediction of patient-reported outcome measures following primary hip and knee total joint arthroplasty. Arch Orthop Trauma Surg. 2023;143(4):2235-2245. doi: 10.1007/s00402-022-04526-x
- Karlin EA, Lin CC, Meftah M, Slover JD, Schwarzkopf R. The impact of machine learning on total joint arthroplasty patient outcomes: A systemic review. J Arthroplasty. 2023;38(10):2085-2095. doi: 10.1016/j.arth.2022.10.039
- BorjaliA, ChenAF, MuratogluOK, MoridMA, VaradarajanKM. Detecting total hip replacement prosthesis design on plain radiographs using deep convolutional neural network. J Orthop Res. 2020;38(7):1465-1471. doi: 10.1002/jor.24617
- Karnuta JM, Murphy MP, Luu BC, et al. Artificial intelligence for automated implant identification in total hip arthroplasty: A multicenter external validation study exceeding two million plain radiographs. J Arthroplasty. 2023;38(10):1998-2003.e1. doi: 10.1016/j.arth.2022.03.002
- Karnuta JM, Haeberle HS, Luu BC, et al. Artificial intelligence to identify arthroplasty implants from radiographs of the hip. J Arthroplasty. 2021;36(7S):S290-S294.e1. doi: 10.1016/j.arth.2020.11.015
- Ren M, Yi PH. Artificial intelligence in orthopedic implant model classification: A systematic review. Skeletal Radiol. 2022;51(2):407-416. doi: 10.1007/s00256-021-03884-8
- Wilson NA, Jehn M, York S, Davis CM 3rd. Revision total hip and knee arthroplasty implant identification: Implications for use of unique device identification 2012 AAHKS member survey results. J Arthroplasty. 2014;29(2):251-255. doi: 10.1016/j.arth.2013.06.027
- Borjali A, Chen AF, Bedair HS, et al. Comparing the performance of a deep convolutional neural network with orthopedic surgeons on the identification of total hip prosthesis design from plain radiographs. Med Phys. 2021;48(5):2327-2336. doi: 10.1002/mp.14705
- Urakawa T, Tanaka Y, Goto S, Matsuzawa H, Watanabe K, Endo N. Detecting intertrochanteric hip fractures with orthopedist-level accuracy using a deep convolutional neural network. Skeletal Radiol. 2019;48(2):239-244. doi: 10.1007/s00256-018-3016-3
- Cheng CT, Ho TY, Lee TY, et al. Application of a deep learning algorithm for detection and visualization of hip fractures on plain pelvic radiographs. Eur Radiol. 2019;29(10):5469-5477. doi: 10.1007/s00330-019-06167-y
- Krogue JD, Cheng KV, Hwang KM, et al. Automatic hip fracture identification and functional subclassification with deep learning. Radiol Artif Intell. 2020;2(2):e190023. doi: 10.1148/ryai.2020190023
- Sato Y, Takegami Y, Asamoto T, et al. Artificial intelligence improves the accuracy of residents in the diagnosis of hip fractures: A multicenter study. BMC Musculoskelet Disord. 2021;22(1):407. doi: 10.1186/s12891-021-04260-2
- Rouzrokh P, Wyles CC, Philbrick KA, et al. A deep learning tool for automated radiographic measurement of acetabular component inclination and version after total hip arthroplasty. J Arthroplasty. 2021;36(7):2510-2517.e6. doi: 10.1016/j.arth.2021.02.026
- Rouzrokh P, Wyles CC, Kurian SJ, et al. Deep learning for radiographic measurement of femoral component subsidence following total hip arthroplasty. Radiol Artif Intell. 2022;4(3):e210206. doi: 10.1148/ryai.210206
- Archer H, Reine S, Alshaikhsalama A, et al. Artificial intelligence-generated hip radiological measurements are fast and adequate for reliable assessment of hip dysplasia: An external validation study. Bone Jt Open. 2022;3(11):877-884. doi: 10.1302/2633-1462.311.BJO-2022-0125.R1
- Xue Y, Zhang R, Deng Y, Chen K, Jiang T. A preliminary examination of the diagnostic value of deep learning in hip osteoarthritis. PLoS One. 2017;12(6):e0178992. doi: 10.1371/journal.pone.0178992
- Üreten K, Arslan T, Gültekin KE, Demir AND, Özer HF, Bilgili Y. Detection of hip osteoarthritis by using plain pelvic radiographs with deep learning methods. Skeletal Radiol. 2020;49(9):1369-1374. doi: 10.1007/s00256-020-03433-9
- Nguyen TP, Chae DS, Park SJ, Yoon J. A novel approach for evaluating bone mineral density of hips based on Sobel gradient-based map of radiographs utilizing convolutional neural network. Comput Biol Med. 2021;132:104298. doi: 10.1016/j.compbiomed.2021.104298
- Fontana MA, Lyman S, Sarker GK, Padgett DE, MacLean CH. Can machine learning algorithms predict which patients will achieve minimally clinically important differences from total joint arthroplasty? Clin Orthop Relat Res. 2019;477(6):1267-1279. doi: 10.1097/CORR.0000000000000687
- Harris AHS, Kuo AC, Bowe TR, Manfredi L, Lalani NF, Giori NJ. Can machine learning methods produce accurate and easy-to-use preoperative prediction models of one-year improvements in pain and functioning after knee arthroplasty? J Arthroplasty. 2021;36(1):112-117.e6. doi: 10.1016/j.arth.2020.07.026
- Kunze KN, Polce EM, Nwachukwu BU, Chahla J, Nho SJ. Development and internal validation of supervised machine learning algorithms for predicting clinically significant functional improvement in a mixed population of primary hip arthroscopy. Arthroscopy. 2021;37(5):1488-1497. doi: 10.1016/j.arthro.2021.01.005
- Huber M, Kurz C, Leidl R. Predicting patient-reported outcomes following hip and knee replacement surgery using supervised machine learning. BMC Med Inform Decis Mak. 2019;19(1):3. doi: 10.1186/s12911-018-0731-6
- Schwartz MH, Ward RE, Macwilliam C, Verner JJ. Using neural networks to identify patients unlikely to achieve a reduction in bodily pain after total hip replacement surgery. Med Care. 1997;35(10):1020-1030. doi: 10.1097/00005650-199710000-00004
- Ramkumar PN, Karnuta JM, Navarro SM, et al. Preoperative prediction of value metrics and a patient-specific payment model for primary total hip arthroplasty: Development and validation of a deep learning model. J Arthroplasty. 2019;34(10):2228-2234.e1. doi: 10.1016/j.arth.2019.04.055
- Ramkumar PN, Navarro SM, Haeberle HS, et al. Development and validation of a machine learning algorithm after primary total hip arthroplasty: Applications to length of stay and payment models. J Arthroplasty. 2019;34(4):632-637. doi: 10.1016/j.arth.2018.12.030
- Magnéli M, Unbeck M, Rogmark C, Sköldenberg O, Gordon M. Measuring adverse events following hip arthroplasty surgery using administrative data without relying on ICD-codes. PLoS One. 2020;15(11):e0242008. doi: 10.1371/journal.pone.0242008
- Shah AA, Devana SK, Lee C, Kianian R, van der Schaar M, SooHoo NF. Development of a novel, potentially universal machine learning algorithm for prediction of complications after total hip arthroplasty. J Arthroplasty. 2021;36(5):1655- 1662.e1. doi: 10.1016/j.arth.2020.12.040
- Harris AHS, Kuo AC, Weng Y, Trickey AW, Bowe T, Giori NJ. Can machine learning methods produce accurate and easy-to-use prediction models of 30-day complications and mortality after knee or hip arthroplasty? Clin Orthop Relat Res. 2019;477(2):452-460. doi: 10.1097/CORR.0000000000000601
- Rouzrokh P, Ramazanian T, Wyles CC, et al. Deep learning artificial intelligence model for assessment of hip dislocation risk following primary total hip arthroplasty from postoperative radiographs. J Arthroplasty. 2021;36(6):2197- 2203.e3. doi: 10.1016/j.arth.2021.02.028
- Karhade AV, Schwab JH, Bedair HS. Development of machine learning algorithms for prediction of sustained postoperative opioid prescriptions after total hip arthroplasty. J Arthroplasty. 2019;34(10):2272-2277.e1. doi: 10.1016/j.arth.2019.06.013
- Loppini M, Gambaro FM, Chiappetta K, Grappiolo G, Bianchi AM, Corino VDA. Automatic identification of failure in hip replacement: An artificial intelligence approach. Bioengineering (Basel). 2022;9(7):288. doi: 10.3390/bioengineering9070288
- Shah RF, Bini SA, Martinez AM, Pedoia V, Vail TP. Incremental inputs improve the automated detection of implant loosening using machine-learning algorithms. Bone Joint J. 2020;102-B(6_Supple_A):101-106. doi: 10.1302/0301-620X.102B6.BJJ-2019-1577.R1
- Charilaou P, Battat R. Machine learning models and over-fitting considerations. World J Gastroenterol. 2022;28(5):605-607. doi: 10.3748/wjg.v28.i5.605
- Karnuta JM, Shaikh HJF, Murphy MP, et al. Artificial intelligence for automated implant identification in knee arthroplasty: A multicenter external validation study exceeding 3.5 million plain radiographs. J Arthroplasty. 2023;38(10):2004-2008. doi: 10.1016/j.arth.2023.03.039
- Yi PH, Wei J, Kim TK, et al. Automated detection and classification of knee arthroplasty using deep learning. Knee. 2020;27(2):535-542. doi: 10.1016/j.knee.2019.11.020
- Kunze KN, Polce EM, Patel A, Courtney PM, Sporer SM, Levine BR. Machine learning algorithms predict within one size of the final implant ultimately used in total knee arthroplasty with good-to-excellent accuracy. Knee Surg Sports Traumatol Arthrosc. 2022;30(8):2565-2572. doi: 10.1007/s00167-022-06866-y
- Kozic N, Weber S, Büchler P, et al. Optimisation of orthopaedic implant design using statistical shape space analysis based on level sets. Med Image Anal. 2010;14(3):265-275. doi: 10.1016/j.media.2010.02.008
- Lambrechts A, Wirix-Speetjens R, Maes F, Van Huffel S. Artificial intelligence based patient-specific preoperative planning algorithm for total knee arthroplasty. Front Robot AI. 2022;9:840282. doi: 10.3389/frobt.2022.840282
- Farooq H, Deckard ER, Carlson J, Ghattas N, Meneghini RM. Coronal and sagittal component position in contemporary total knee arthroplasty: Targeting native alignment optimizes clinical outcomes. J Arthroplasty. 2023;38(7 Suppl 2):S245-S251. doi: 10.1016/j.arth.2023.04.040
- Farooq H, Deckard ER, Arnold NR, Meneghini RM. Machine learning algorithms identify optimal sagittal component position in total knee arthroplasty. J Arthroplasty. 2021;36(7S):S242-S249. doi: 10.1016/j.arth.2021.02.063
- Kim MS, Cho RK, Yang SC, Hur JH, In Y. Machine learning for detecting total knee arthroplasty implant loosening on plain radiographs. Bioengineering (Basel). 2023;10(6):632. doi: 10.3390/bioengineering10060632
- Mehta B, Goodman S, DiCarlo E, et al. Machine learning identification of thresholds to discriminate osteoarthritis and rheumatoid arthritis synovial inflammation. Arthritis Res Ther. 2023;25(1):31. doi: 10.1186/s13075-023-03008-8
- Joseph GB, McCulloch CE, Nevitt MC, Link TM, Sohn JH. Machine learning to predict incident radiographic knee osteoarthritis over 8 years using combined MR imaging features, demographics, and clinical factors: Data from the osteoarthritis initiative. Osteoarthritis Cartilage. 2022;30(2):270-279. doi: 10.1016/j.joca.2021.11.007
- Leung K, Zhang B, Tan J, et al. Prediction of total knee replacement and diagnosis of osteoarthritis by using deep learning on knee radiographs: Data from the osteoarthritis initiative. Radiology. 2020;296(3):584-593. doi: 10.1148/radiol.2020192091
- Tiulpin A, Klein S, Bierma-Zeinstra SMA, et al. Multimodal machine learning-based knee osteoarthritis progression prediction from plain radiographs and clinical data. Sci Rep. 2019;9(1):20038. doi: 10.1038/s41598-019-56527-3
- Farooq H, Deckard ER, Ziemba-Davis M, Madsen A, Meneghini RM. Predictors of patient satisfaction following primary total knee arthroplasty: Results from a traditional statistical model and a machine learning algorithm. J Arthroplasty. 2020;35(11):3123-3130. doi: 10.1016/j.arth.2020.05.077
- Kunze KN, Polce EM, Sadauskas AJ, Levine BR. Development of machine learning algorithms to predict patient dissatisfaction after primary total knee arthroplasty. J Arthroplasty. 2020;35(11):3117-3122. doi: 10.1016/j.arth.2020.05.061
- Mohammadi R, Jain S, Namin AT, et al. Predicting unplanned readmissions following a hip or knee arthroplasty: Retrospective observational study. JMIR Med Inform. 2020;8(11):e19761. doi: 10.2196/19761
- Navarro SM, Wang EY, Haeberle HS, et al. Machine learning and primary total knee arthroplasty: Patient forecasting for a patient-specific payment model. J Arthroplasty. 2018;33(12):3617-3623. doi: 10.1016/j.arth.2018.08.028
- Ramkumar PN, Karnuta JM, Navarro SM, et al. Deep learning preoperatively predicts value metrics for primary total knee arthroplasty: Development and validation of an artificial neural network model. J Arthroplasty. 2019;34(10):2220-2227.e1. doi: 10.1016/j.arth.2019.05.034
- Ko S, Jo C, Chang CB, et al. A web-based machine-learning algorithm predicting postoperative acute kidney injury after total knee arthroplasty. Knee Surg Sports Traumatol Arthrosc. 2022;30(2):545-554. doi: 10.1007/s00167-020-06258-0
- Jo C, Ko S, Shin WC, et al. Transfusion after total knee arthroplasty can be predicted using the machine learning algorithm. Knee Surg Sports Traumatol Arthrosc. 2020;28(6):1757-1764. doi: 10.1007/s00167-019-05602-3
- Aram P, Trela-Larsen L, Sayers A, et al. Estimating an individual’s probability of revision surgery after knee replacement: A comparison of modeling approaches using a national data set. Am J Epidemiol. 2018;187(10):2252-2262. doi: 10.1093/aje/kwy121
- El-Galaly A, Grazal C, Kappel A, Nielsen PT, Jensen SL, Forsberg JA. Can machine-learning algorithms predict early revision TKA in the Danish knee arthroplasty registry? Clin Orthop Relat Res. 2020;478(9):2088-2101. doi: 10.1097/CORR.0000000000001343
- Katakam A, Karhade AV, Schwab JH, Chen AF, Bedair HS. Development and validation of machine learning algorithms for postoperative opioid prescriptions after TKA. J Orthop. 2020;22:95-99. doi: 10.1016/j.jor.2020.03.052
- The White House. Executive Order on the Safe, Secure, and Trustworthy Development and Use of Artificial Intelligence. The White House; 2023. Available from: https://www.whitehouse.gov/briefing-room/ presidential-actions/2023/10/30/executive-order-on-the-safe-secure-and-trustworthy-development-and-use-of-artificial-intelligence [Last accessed on 2024 May 13].
- Yi PH, Kim TK, Wei J, et al. Automated detection and classification of shoulder arthroplasty models using deep learning. Skeletal Radiol. 2020;49(10):1623-1632. doi: 10.1007/s00256-020-03463-3
- Urban G, Porhemmat S, Stark M, Feeley B, Okada K, Baldi P. Classifying shoulder implants in X-ray images using deep learning. Comput Struct Biotechnol J. 2020;18:967-972. doi: 10.1016/j.csbj.2020.04.005
- Sultan H, Owais M, Park C, Mahmood T, Haider A, Park KR. Artificial intelligence-based recognition of different types of shoulder implants in X-ray scans based on dense residual ensemble-network for personalized medicine. J Pers Med. 2021;11(6):482. doi: 10.3390/jpm11060482
- Kunze KN, Jang SJ, Li TY, et al. Artificial intelligence for automated identification of total shoulder arthroplasty implants. J Shoulder Elbow Surg. 2023;32(10):2115-2122. doi: 10.1016/j.jse.2023.03.028
- Fucentese SF, Costouros JG, Kühnel SP, Gerber C. Total shoulder arthroplasty with an uncemented soft-metal-backed glenoid component. J Shoulder Elbow Surg. 2010;19(4):624-631. doi: 10.1016/j.jse.2009.12.021
- DeLude JA, Bicknell RT, MacKenzie GA, et al. An anthropometric study of the bilateral anatomy of the humerus. J Shoulder Elbow Surg. 2007;16(4):477-483. doi: 10.1016/j.jse.2006.09.016
- Tschannen M, Vlachopoulos L, Gerber C, Székely G, Fürnstahl P. Regression forest-based automatic estimation of the articular margin plane for shoulder prosthesis planning. Med Image Anal. 2016;31:88-97. doi: 10.1016/j.media.2016.02.008
- Polce EM, Kunze KN, Fu MC, et al. Development of supervised machine learning algorithms for prediction of satisfaction at 2 years following total shoulder arthroplasty. J Shoulder Elbow Surg. 2021;30(6):e290-e299. doi: 10.1016/j.jse.2020.09.007
- Menendez ME, Shaker J, Lawler SM, Ring D, Jawa A. Negative patient-experience comments after total shoulder arthroplasty. J Bone Joint Surg Am. 2019;101(4):330-337. doi: 10.2106/JBJS.18.00695
- McLendon PB, Christmas KN, Simon P, et al. Machine learning can predict level of improvement in shoulder arthroplasty. JB JS Open Access. 2021;6(1):e20.00128. doi: 10.2106/JBJS.OA.20.00128
- Roche C, Kumar V, Overman S, et al. Validation of a machine learning-derived clinical metric to quantify outcomes after total shoulder arthroplasty. J Shoulder Elbow Surg. 2021;30(10):2211-2224. doi: 10.1016/j.jse.2021.01.021
- Biron DR, Sinha I, Kleiner JE, et al. A novel machine learning model developed to assist in patient selection for outpatient total shoulder arthroplasty. J Am Acad Orthop Surg. 2020;28(13):e580-e585. doi: 10.5435/JAAOS-D-19-00395
- Karnuta JM, Churchill JL, Haeberle HS, et al. The value of artificial neural networks for predicting length of stay, discharge disposition, and inpatient costs after anatomic and reverse shoulder arthroplasty. J Shoulder Elbow Surg. 2020;29(11):2385-2394. doi: 10.1016/j.jse.2020.04.009
- Arvind V, London DA, Cirino C, Keswani A, Cagle PJ. Comparison of machine learning techniques to predict unplanned readmission following total shoulder arthroplasty. J Shoulder Elbow Surg. 2021;30(2):e50-e59. doi: 10.1016/j.jse.2020.05.013
- Gowd AK, Agarwalla A, Beck EC, et al. Prediction of total healthcare cost following total shoulder arthroplasty utilizing machine learning. J Shoulder Elbow Surg. 2022;31(12):2449-2456. doi: 10.1016/j.jse.2022.07.013
- Gowd AK, Agarwalla A, Amin NH, et al. Construct validation of machine learning in the prediction of short-term postoperative complications following total shoulder arthroplasty. J Shoulder Elbow Surg. 2019;28(12):e410-e421. doi: 10.1016/j.jse.2019.05.017
- Lopez CD, Constant M, Anderson MJJ, Confino JE, Heffernan JT, Jobin CM. Using machine learning methods to predict nonhome discharge after elective total shoulder arthroplasty. JSES Int. 2021;5(4):692-698. doi: 10.1016/j.jseint.2021.02.011
- Kumazu Y, Kobayashi N, Kitamura N, et al. Automated segmentation by deep learning of loose connective tissue fibers to define safe dissection planes in robot-assisted gastrectomy. Sci Rep. 2021;11(1):21198. doi: 10.1038/s41598-021-00557-3
- Chen J, Oh PJ, Cheng N, et al. Use of automated performance metrics to measure surgeon performance during robotic vesicourethral anastomosis and methodical development of a training tutorial. J Urol. 2018;200(4):895-902. doi: 10.1016/j.juro.2018.05.080
- Ali S, Jonmohamadi Y, Fontanarosa D, Crawford R, Pandey AK. One step surgical scene restoration for robot assisted minimally invasive surgery. Sci Rep. 2023;13(1):3127. doi: 10.1038/s41598-022-26647-4
- Wang F, Sun X, Li J. Surgical smoke removal via residual Swin transformer network. Int J Comput Assist Radiol Surg. 2023;18(8):1417-1427. doi: 10.1007/s11548-023-02835-z
- Capelli G, Verdi D, Frigerio I, et al. Artificial intelligence surgery editorial board study group on ethics. White paper: Ethics and trustworthiness of artificial intelligence in clinical surgery. Art Int Surg. 2023;3:111-122. doi: 10.20517/ais.2023.04
- Taher H, Grasso V, Tawfik S, Gumbs A. The challenges of deep learning in artificial intelligence and autonomous actions in surgery: A literature review. Art Int Surg. 2022;2:144-158. doi: 10.20517/ais.2022.11
- Gumbs AA, Alexander F, Karcz K, et al. White paper: Definitions of artificial intelligence and autonomous actions in clinical surgery. Art Int Surg. 2022;2:93-100. doi: 10.20517/ais.2022.10