A stacked ensemble deep learning model for predicting the intensive care unit patient mortality
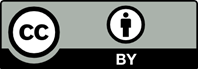
Accurate mortality prediction in intensive care units (ICUs) is essential for optimizing patient treatment, nursing care, and resource allocation. Traditional models, such as Acute Physiology and Chronic Health Evaluation and Simplified Acute Physiology Score, have been very important in clinical practice, but they frequently have issues with prediction accuracy and adaptability, especially when dealing with complex and evolving patient data. These issues can be resolved, and the accuracy of mortality prediction increased due to recent developments in machine learning, especially deep learning. The present study introduces a new deep learning ensemble model that achieves a significant improvement over existing methods. Using stacked ensemble learning, our approach combines the advantages of one Random Forests model and two CatBoost models. We achieved a notable performance in mortality prediction by carefully training and optimizing this ensemble using the electronic ICU Collaborative Research Database. Our model boasts an accuracy of 94.19%, precision of 94.097%, recall of 94.29%, and F1-score of 94.191%, demonstrating a substantial improvement over conventional approaches. The prediction of ICU mortality has been significantly improved using ensemble learning, which helps medical and nursing staff to better treat patients individually, allocate resources efficiently, and enhance patient outcomes. This approach gives healthcare experts the ability to make data-driven decisions, leading to more effective and efficient care within the ICU.
- Simopoulos D, Kosmidis D, Koutsouki S, Bonnotte N, Anastassopoulos G. Advanced mortality prediction in adult ICU: Introducing a deep learning approach in healthcare. In: Maglogiannis I, liadis L, Macintyre J, Avlonitis M, Papaleonidas A, editors. IFIP Advances in Information and Communication Technology. Vol. 711. Switzerland: Springer Nature; 2024. p. 17-30. doi: 10.1007/978-3-031-63211-2_2
- Moreno RP, Metnitz PGH, et al. SAPS 3-From evaluation of the patient to evaluation of the intensive care unit. Part 2: Development of a prognostic model for hospital mortality at ICU admission. Intensive Care Med. 2005;31(10):1345-1355. doi: 10.1007/s00134-005-2762-6
- Zimmerman JE, Kramer AA, McNair DS, Malila FM. Acute physiology and chronic health evaluation (APACHE) IV: Hospital mortality assessment for today’s critically ill patients. Crit Care Med. 2006;34(5):1297-310. doi: 10.1097/01.CCM.0000215112.84523.F0
- Fadja AN, Fraccaroli M, Bizzarri A, Mazzuchelli G, Lamma E. Neural-symbolic ensemble learning for early-stage prediction of critical state of covid-19 patients. Med Biol Eng Comput. 2022;60(12):3461-3474. doi: 10.1007/s11517-022-02674-1
- Ho KM, Dobb GJ, Knuiman M, Finn J, Lee KY, Webb SAR. A comparison of admission and worst 24-hour Acute Physiology and Chronic Health Evaluation II scores in predicting hospital mortality: A retrospective cohort study. Crit Care. 2005;10(1):R4. doi: 10.1186/cc3913
- Falcão ALE, Barros AGA, Bezerra AAM, et al. The prognostic accuracy evaluation of SAPS 3, SOFA and APACHE II scores for mortality prediction in the surgical ICU: An external validation study and decision-making analysis. Ann Intensive Care. 2019;9(1):18. doi: 10.1186/s13613-019-0488-9
- Polderman KH, Girbes ARJ, Thijs LG, Strack van Schijndel RJM. Accuracy and reliability of APACHE II scoring in two intensive care units problems and pitfalls in the use of APACHE II and suggestions for improvement. Anaesthesia. 2001;56(1):47-50. doi: 10.1046/j.1365-2044.2001.01763.x
- Sedloň P, Kameník L, Škvařil J, Malý M, Táborský M, Zavoral M. Comparison of the accuracy and correctness of mortality estimates for intensive care unit patients in internal clinics of the Czech Republic using APACHE II, APACHE IV, SAPS 3 and MPMoIII models. Med Glas (Zenica). 2016;13(2):82-89. doi: 10.17392/860-16
- Subudhi S, Verma A, Patel AB, et al. Comparing machine learning algorithms for predicting ICU admission and mortality in COVID-19. NPJ Digit Med. 2021;4(1):87. doi: 10.1038/s41746-021-00456-x
- Choi MH, Kim D, Choi EJ, et al. Mortality prediction of patients in intensive care units using machine learning algorithms based on electronic health records. Sci Rep. 2022;12(1):7180. doi: 10.1038/s41598-022-11226-4
- Bi S, Chen S, Li J, Gu J. Machine learning-based prediction of in-hospital mortality for post cardiovascular surgery patients admitting to intensive care unit: A retrospective observational cohort study based on a large multi-center critical care database. Comput Methods Programs Biomed. 2022;226:107115. doi: 10.1016/j.cmpb.2022.107115
- Huang B, Liang D, Zou R, et al. Mortality prediction for patients with acute respiratory distress syndrome based on machine learning: A population-based study. Ann Transl Med. 2021;9(9):794. doi: 10.21037/atm-20-6624
- Chiu CC, Wu CM, Chien TN, Kao LJ, Li C, Jiang HL. Applying an improved stacking ensemble model to predict the mortality of ICU patients with heart failure. J Clin Med. 2022;11(21):6460. doi: 10.3390/jcm11216460
- Yang J, Peng H, Luo Y, Zhu T, Xie L. Explainable ensemble machine learning model for prediction of 28-day mortality risk in patients with sepsis-associated acute kidney injury. Front Med (Lausanne). 2023;10:1165129. doi: 10.3389/fmed.2023.1165129
- Chassagnon G, Vakalopoulou M, Battistella E, et al. AI-driven quantification, staging and outcome prediction of COVID-19 pneumonia. Med Image Anal. 2021;67:101860. doi: 10.1016/j.media.2020.101860
- Alabbad DA, Almuhaideb AM, Alsunaidi SJ, et al. Machine learning model for predicting the length of stay in the intensive care unit for Covid-19 patients in the eastern province of Saudi Arabia. Inform Med Unlocked. 2022;30. doi: 10.1016/j.imu.2022.100937
- Alzubaidi L, Zhang J, Humaidi AJ, et al. Review of deep learning: Concepts, CNN architectures, challenges, applications, future directions. J Big Data. 2021;8(1):100937. doi: 10.1186/s40537-021-00444-8
- Pollard TJ, Johnson AEW, Raffa JD, Celi LA, Mark RG, Badawi O. The eICU collaborative research database, a freely available multi-center database for critical care research. Sci Data. 2018;5:180178. doi: 10.1038/sdata.2018.178
- Bach M, Werner A, Palt M. The proposal of undersampling method for learning from imbalanced datasets. Procedia Comput Sci. 2019;159:125-134. doi: 10.1016/j.procs.2019.09.167
- Breiman L. Random forests. Mach Learn. 2001;45(1):5-32. doi: 10.1023/A:1010933404324
- Geurts P, Ernst D, Wehenkel L. Extremely randomized trees. Mach Learn. 2006;63(1):3-42. doi: 10.1007/s10994-006-6226-1
- Chen T, Guestrin C. XGBoost: A Scalable Tree Boosting System. In: Proceedings of the 22nd ACM SIGKDD International Conference on Knowledge Discovery and Data Mining. KDD ’16. Association for Computing Machinery; 2016. p. 785-794. doi: 10.1145/2939672.2939785
- Prokhorenkova L, Gusev G, Vorobev A, Dorogush AV, Gulin A. CatBoost: Unbiased boosting with categorical features. Adv Neural Inf Process Syst. 2018;31: 2-8.
- Ke G, Meng Q, Finley T, et al. LightGBM: A highly efficient gradient boosting decision tree. In: Advances in Neural Information Processing Systems. Vol. 2017. United States: The MIT Press; 2017.
- McCulloch WS, Pitts W. A logical calculus of the ideas immanent in nervous activity: The bulletin of mathematical biophysics. Bull Math Biophys. 1943;5(4):115-133. doi: 10.1007/BF02478259
- Caruana R, Niculescu-Mizil A, Crew G, Ksikes A. Ensemble selection from libraries of models. In: Proceedings of the Twenty-First International Conference on Machine Learning; 2004. doi: 10.1145/1015330.1015432
- Alshari H, Abdulrazak Yahya S, Odabaş A. Comparison of gradient boosting decision tree algorithms for CPU performance. Erciyes Univ J Inst Sci Technol. 2021;37(1):157-168.
- Anghel A, Papandreou N, Parnell T, De Palma A, Pozidis H. Benchmarking and Optimization of Gradient Boosting Decision Tree Algorithms. [arXiv Preprint]. doi: 10.48550/arXiv.1809.04559
- Wolpert DH. Stacked generalization. Neural Netw. 1992;5(2):241-259. doi: 10.1016/S0893-6080(05)80023-1
- Viton F, Elbattah M, Guérin JL, Dequen G. Multi-channel ConvNet approach to predict the risk of in-hospital mortality for ICU patients. In: Proceedings of the 1st International Conference on Deep Learning Theory and Applications. Portugal: SCITEPRESS-Science and Technology Publications; 2020. p. 98-102. doi: 10.5220/0009891900980102
- Darabi HR, Tsinis D, Zecchini K, Whitcomb WF, Liss A. Forecasting mortality risk for patients admitted to intensive care units using machine learning. In: Procedia Comput Sci. 2018;140:306-313. doi: 10.1016/j.procs.2018.10.313
- Dan T, Li Y, Zhu Z, et al. Machine Learning to Predict ICU Admission, ICU Mortality and Survivors’ Length of Stay among COVID-19 Patients: Toward Optimal Allocation of ICU Resources. In: Proceedings-2020 IEEE International Conference on Bioinformatics and Biomedicine, BIBM 2020; 2020. doi: 10.1109/BIBM49941.2020.9313292
- Yu L, Halalau A, Dalal B, et al. Machine learning methods to predict mechanical ventilation and mortality in patients with COVID-19. PLoS One. 2021;16(4):e0249285. doi: 10.1371/journal.pone.0249285
- Hwangbo L, Kang YJ, Kwon H, et al. Stacking ensemble learning model to predict 6-month mortality in ischemic stroke patients. Sci Rep. 2022;12(1):17389. doi: 10.1038/s41598-022-22323-9
- Iwase S, Nakada TA, Shimada T, et al. Prediction algorithm for ICU mortality and length of stay using machine learning. Sci Rep. 2022;12(1):12912. doi: 10.1038/s41598-022-17091-5
- Pang K, Li L, Ouyang W, Liu X, Tang Y. Establishment of ICU mortality risk prediction models with machine learning algorithm using MIMIC-IV database. Diagnostics. 2022;12(5):1068. doi: 10.3390/diagnostics12051068
- Saadatmand S, Salimifard K, Mohammadi R, Kuiper A, Marzban M, Farhadi A. Using machine learning in prediction of ICU admission, mortality, and length of stay in the early stage of admission of COVID-19 patients. Ann Oper Res. 2023;328(1):1-29. doi: 10.1007/s10479-022-04984-x
- Sun Y, He Z, Ren J, Wu Y. Prediction model of in-hospital mortality in intensive care unit patients with cardiac arrest: A retrospective analysis of MIMIC-IV database based on machine learning. BMC Anesthesiol. 2023;23(1):178. doi: 10.1186/s12871-023-02138-5
- Churpek MM, Snyder A, Han X, et al. Quick sepsis-related organ failure assessment, systemic inflammatory response syndrome, and early warning scores for detecting clinical deterioration in infected patients outside the intensive care unit. Am J Respir Crit Care Med. 2017;195(7):906-911. doi: 10.1164/rccm.201604-0854OC
- Liu X, Niu H, Peng J. Enhancing predictions with a stacking ensemble model for ICU mortality risk in patients with sepsis-associated encephalopathy. J Int Med Res. 2024;52(3):03000605241239013. doi: 10.1177/03000605241239013
- Freijeiro-González L, Febrero-Bande M, González- Manteiga W. A critical review of LASSO and its derivatives for variable selection under dependence among covariates. Int Stat Rev. 2022;90(1):118-145. doi: 10.1111/insr.12469
- Ren N, Zhao X, Zhang X. Mortality prediction in ICU using a stacked ensemble model. Comput Math Methods Med. 2022;2022:3938492. doi: 10.1155/2022/3938492
- Wong J, Murray Horwitz M, Zhou L, Toh S. Using machine learning to identify health outcomes from electronic health record data. Curr Epidemiol Rep. 2018;5(4):331-342. doi: 10.1007/s40471-018-0165-9
- Si Y, Du J, Li Z, et al. Deep representation learning of patient data from Electronic Health Records (EHR): A systematic review. J Biomed Inform. 2021;115:103671. doi: 10.1016/j.jbi.2020.103671
- Nguyen K, Wilson DL, Diiulio J, et al. Design and development of a machine-learning-driven opioid overdose risk prediction tool integrated in electronic health records in primary care settings. Bioelectron Med. 2024;10(1):24. doi: 10.1186/s42234-024-00156-3
- BuHamra SS, Almutairi AN, Buhamrah AK, Almadani SH, Alibrahim YA. An NLP tool for data extraction from electronic health records: COVID-19 mortalities and comorbidities. Front Public Health. 2022;10:1070870. doi: 10.3389/fpubh.2022.1070870
- Kuo ZM, Chen KF, Tseng YJ. MoCab: A framework for the deployment of machine learning models across health information systems. Comput Methods Programs Biomed. 2024;255:108336. doi: 10.1016/j.cmpb.2024.108336
- Kawamoto K, Finkelstein J, Del Fiol G. Implementing machine learning in the electronic health record: Checklist of essential considerations. Mayo Clin Proc. 2023;98(3):366-369. doi: 10.1016/j.mayocp.2023.01.013