Role of large language models in improving provider–patient experience and interaction efficiency: A scoping review
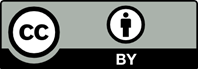
Large language models (LLMs) have rapidly emerged as transformative tools across multiple domains, including healthcare. The ability of LLMs to process vast amounts of data and generate human-like responses has facilitated their integration into patient care, particularly in enhancing communication, improving patient satisfaction, and streamlining administrative processes. Despite this potential, there are concerns regarding their accuracy, reliability, and ethical use in clinical settings. This scoping review aims to investigate and map the current literature on the use of LLMs in improving provider–patient experience and interaction efficiency. Following the Preferred Reporting Items for Systematic Reviews and Meta-Analyses extension for Scoping Reviews guidelines, we conducted a systematic search of Ovid MEDLINE, PubMed, and Google Scholar databases to identify relevant articles published between January 2015 and June 2024. Of the 3568 articles initially screened, 47 satisfied the inclusion criteria. These articles spanned 13 countries and encompassed diverse healthcare settings. Thematic areas of LLM utilization included improving communication between patients and healthcare providers, resolving patient inquiries, enhancing patient education, and increasing operational efficiency. Although numerous studies have yielded positive outcomes, significant challenges related to data accuracy, hallucinations, bias, and ethical concerns remain. LLMs can considerably improve patient experience in healthcare, particularly in areas of communication, education, and administrative efficiency. However, concerns regarding accuracy, ethical implications, and the need for rigorous safeguards to prevent misinformation impede their widespread adoption. Future research should focus on developing context-specific LLMs tailored to healthcare environments and addressing the identified limitations to optimize their implementation in clinical practice.
- Singhal K, Azizi S, Tu T, et al. Large language models encode clinical knowledge. Nature. 2023;620(7974):172-180. doi: 10.1038/s41586-023-06291-2
- Binz M, Schulz E. Using cognitive psychology to understand GPT-3. Proc Natl Acad Sci U S A. 2023;120(1):e2218523120. doi: 10.1073/pnas.2218523120
- Kung TH, Cheatham M, Medenilla A, et al. Performance of ChatGPT on USMLE: Potential for AI-assisted medical education using large language models. PLoS Digit Health. 2023;2(1):e0000198. doi: 10.1371/journal.pdig.0000198
- Chowdhery A, Narang S, Devlin J, et al. PaLM: Scaling language modeling with pathways. J Mach Learn Res. 2023;24:1-113.
- OpenAI. ChatGPT Plugins; 2023. Available from: https:// openai.com/blog/chatgpt-plugins [Last accessed on 2024 Jun 08].
- Sanderson K. GPT-4 is here: What scientists think. Nature. 2023;615(7950):773. doi: 10.1038/d41586-023-00753-8
- Farquhar S, Kossen J, Kuhn L, Gal Y. Detecting hallucinations in large language models using semantic entropy. Nature. 2024;630(7948):625-630. doi: 10.1038/s41586-024-07421-0
- Dong Y, Mu R, Jin G, et al. Building guardrails for Large Language Models. arXiv; 2024 Available from: https://arxiv. org/abs/2402.01822 [Last accessed on 2024 Jun 09]. doi: 10.48550/arXiv.2402.01822.
- Liu X, Sun T, Xu T, et al. SHIELD: Evaluation and defense strategies for copyright compliance in LLM text generation. arXiv; 2024. Available from: https://arxiv.org/abs/2406.12975 [Last accessed on 2024 Jul 09]. doi:10.48550/arXiv.2406.12975.
- Saadé RG, Morin D, Thomas JDE. Critical thinking in E-learning environments. Comput Hum Behav. 2012;28(5):1608-1617. doi: 10.1016/j.chb.2012.03.025
- Tricco AC, Lillie E, Zarin W, et al. PRISMA extension for scoping reviews (PRISMA-ScR): Checklist and explanation. Ann Intern Med. 2018;169(7):467-473. doi: 10.7326/M18-0850
- Klang E, Sourosh A, Nadkarni GN, Sharif K, Lahat A. Evaluating the role of ChatGPT in gastroenterology: A comprehensive systematic review of applications, benefits, and limitations. Ther Adv Gastroenterol. 2023;16:17562848231218618. doi: 10.1177/17562848231218618
- Cè M, Chiarpenello V, Bubba A, et al. Exploring the role of ChatGPT in oncology: Providing information and support for cancer patients. Biomedinformatics. 2024;4(2):877. doi: 10.3390/biomedinformatics4020049
- Al Kuwaiti A, Nazer K, Al-Reedy A, et al. A review of the role of artificial intelligence in healthcare. J Pers Med. 2023;13(6):951. doi: 10.3390/jpm13060951
- Javaid M, Haleem A, Singh RP. ChatGPT for healthcare services: An emerging stage for an innovative perspective. Tech Bench. 2023;3:100105. doi: 10.1016/j.tbench.2023.100105
- Lahat A, Shachar E, Avidan B, et al. Evaluating the utility of a large language model in answering common patients’ gastrointestinal health-related questions: Are we there yet? Diagnostics (Basel). 2023;13(11):1950. doi: 10.3390/diagnostics13111950
- Yang R, Tan TF, Lu W, Thirunavukarasu AJ, Ting DSW, Liu N. Large language models in health care: Development, applications, and challenges. Health Care Sci. 2023;2(4): 255-263. doi: 10.1002/hcs2.61
- Alanzi TM. Impact of ChatGPT on teleconsultants in healthcare: Perceptions of healthcare experts in Saudi Arabia. J Multidiscip Healthc. 2023;16:2309-2321. doi: 10.2147/JMDH.S419847
- Preiksaitis C, Ashenburg N, Bunney G, et al. The role of large language models in transforming emergency medicine: Scoping review. JMIR Med Inform. 2024;12(1):e53787. doi: 10.2196/53787
- Aramaki E, Wakamiya S, Yada S, Nakamura Y. Natural language processing: From bedside to everywhere. Yearb Med Inform. 2022;31(1):243-253. doi: 10.1055/s-0042-1742510
- Reading Turchioe M, Volodarskiy A, Pathak J, Wright DN, Tcheng JE, Slotwiner D. Systematic review of current natural language processing methods and applications in cardiology. Heart. 2022;108(12):909-916. doi: 10.1136/heartjnl-2021-319769
- Yang X, Chen A, PourNejatian N, et al. A large language model for electronic health records. NPJ Digit Med. 2022;5(1):194. doi: 10.1038/s41746-022-00742-2
- Nathaniel Kumar Sarella P, Therissa Mangam V. AI-driven natural language processing in healthcare: Transforming patient-provider communication. Indian J Pharm Pract. 2024;17(1):21-26. doi: 10.5530/ijopp.17.1.4
- Mika AP, Martin JR, Engstrom SM, Polkowski GG, Wilson JM. Assessing ChatGPT responses to common patient questions regarding total hip arthroplasty. J Bone Joint Surg Am. 2023;105(19):1519-1526. doi: 10.2106/JBJS.23.00209
- Yeo YH, Samaan JS, Ng WH, et al. Assessing the performance of ChatGPT in answering questions regarding cirrhosis and hepatocellular carcinoma. Clin Mol Hepatol. 2023;29(3): 721-732. doi: 10.3350/cmh.2023.0089
- Shao CY, Li H, Liu XL, et al. Appropriateness and comprehensiveness of using ChatGPT for perioperative patient education in thoracic surgery in different language contexts: Survey study. Interact J Med Res. 2023;12:e46900. doi: 10.2196/46900
- Hernandez CA, Vazquez Gonzalez AE, Polianovskaia A, et al. The future of patient education: AI-driven guide for type 2 diabetes. Cureus. 2023;15(11):e48919. doi: 10.7759/cureus.48919
- Lee TJ, Campbell DJ, Rao AK, et al. Evaluating ChatGPT responses on atrial fibrillation for patient education. Cureus. 2024;16(6):e61680. doi: 10.7759/cureus.61680
- Gray M, Baird A, Sawyer T, et al. Increasing realism and variety of virtual patient dialogues for prenatal counseling education through a novel application of ChatGPT: Exploratory observational study. JMIR Med Educ. 2024;10:e50705. doi: 10.2196/50705
- Gala D, Makaryus AN. The utility of language models in cardiology: A narrative review of the benefits and concerns of ChatGPT-4. Int J Environ Res Public Health. 2023;20(15):6438. doi: 10.3390/ijerph20156438
- Giuffrè M, Kresevic S, Pugliese N, You K, Shung DL. Optimizing large language models in digestive disease: Strategies and challenges to improve clinical outcomes. Liver Int. 2024;44(9):2114-2124. doi: 10.1111/liv.15974
- Fink MA. Große Sprachmodelle wie ChatGPT und GPT-4 für eine patientenzentrierte radiologie. Radiologie (Heidelb). 2023;63(9):665-671. doi: 10.1007/s00117-023-01187-8
- Sorin V, Glicksberg BS, Artsi Y, et al. Utilizing large language models in breast cancer management: Systematic review. J Cancer Res Clin Oncol. 2024;150(3):140. doi: 10.1007/s00432-024-05678-6
- Shahab O, El Kurdi B, Shaukat A, et al. Large language models: A primer and gastroenterology applications. Therap Adv Gastroenterol. 2024;17:17562848241227031. doi: 10.1177/17562848241227031
- Nolin-Lapalme A, Theriault-Lauzier P, Corbin D, et al. Maximizing large language model utility in cardiovascular care: A practical guide. Can J Cardiol. 2024;40(10): 1774-1787. doi: 10.1016/j.cjca.2024.05.024
- Lawson McLean A, Wu Y, Lawson McLean AC, Hristidis V. Large language models as decision aids in neuro-oncology: A review of shared decision-making applications. J Cancer Res Clin Oncol. 2024;150(3):139. doi: 10.1007/s00432-024-05673-xIttarat
- Ittarat M, Cheungpasitporn W, Chansangpetch S. Personalized care in eye health: Exploring opportunities, challenges, and the road ahead for chatbots. J Pers Med. 2023;13(12):1679. doi: 10.3390/jpm13121679
- Kim J, Chen ML, Rezaei SJ, et al. Patient Perspectives on Large Language Model Responses to Patient Messages. Rochester, NY; 2024. Available from: https://papers.ssrn. com/abstract=4867523 [Last accessed on 2024 Sep 03].
- Yang X, Xiao Y, Liu D, et al. Enhancing Doctor-Patient Communication using Large Language Models for Pathology Report Interpretation; 2024. doi: 10.21203/rs.3.rs-4557335/v1
- Zernikow J, Grassow L, Gröschel J, Henrion P, Wetzel PJ, Spethmann S. Application of “large language models” in the clinic: Does ChatGPT replace medical letter writing? A case report. Inn Med (Heidelb). 2023;64(11):1058-1064. doi: 10.1007/s00108-023-01600-3
- Parker RD, Mancini K, Abram MD. Natural language processing-enhanced qualitative methods: An opportunity to improve health outcomes. Int J Qual Methods. 2023;22:16094069231168678. doi: 10.1177/16094069231168678
- Guevara M, Chen S, Thomas S, et al. Large language models to identify social determinants of health in electronic health records. NPJ Digit Med. 2024;7(1):6. doi: 10.1038/s41746-023-00970-0
- Jeyaraman M, Balaji S, Jeyaraman N, Yadav S. Unraveling the ethical enigma: Artificial intelligence in healthcare. Cureus. 2023;15(8):e43262. doi: 10.7759/cureus.43262
- Zaretsky J, Kim JM, Baskharoun S, et al. Generative artificial intelligence to transform inpatient discharge summaries to patient-friendly language and format. JAMA Netw Open. 2024;7(3):e240357. doi: 10.1001/jamanetworkopen.2024.0357
- Fernandes M, Sun H, Jain A, et al. Classification of the disposition of patients hospitalized with COVID-19: Reading discharge summaries using natural language processing. JMIR Med Inform. 2021;9(2):e25457. doi: 10.2196/25457
- Taggart M, Chapman WW, Steinberg BA, et al. Comparison of 2 natural language processing methods for identification of bleeding among critically ill patients. JAMA Netw Open. 2018;1(6):e183451. doi: 10.1001/jamanetworkopen.2018.3451
- Al-Harrasi AM, Iqbal E, Tsamakis K, et al. Motor signs in Alzheimer’s disease and vascular dementia: Detection through natural language processing, co-morbid features and relationship to adverse outcomes. Exp Gerontol. 2021;146:111223. doi: 10.1016/j.exger.2020.111223
- Magrangeas TT, Kolliakou A, Sanyal J, Patel R, Stewart R. Investigating the relationship between thought interference, somatic passivity, and outcomes in patients with psychosis: A natural language processing approach using a clinical records search platform in south London. BMJ Open. 2022;12(8):e057433. doi: 10.1136/bmjopen-2021-057433
- Joyce C, Markossian TW, Nikolaides J, et al. The evaluation of a clinical decision support tool using natural language processing to screen hospitalized adults for unhealthy substance use: Protocol for a quasi-experimental design. JMIR Res Protoc. 2022;11(12):e42971. doi: 10.2196/42971
- Heston TF. Safety of large language models in addressing depression. Cureus. 2023;15(12):e50729. doi: 10.7759/cureus.50729
- Li Z, Xing X, Lu B, et al. Early Prediction of 30-day ICU re-Admissions Using Natural Language Processing and Machine Learning. arXiv; 2019. Available from: https://arxiv. org/abs/1910.02545 [Last accessed on 2024 Sep 03].
- Tripathi S, Sukumaran R, Cook TS. Efficient healthcare with large language models: Optimizing clinical workflow and enhancing patient care. J Am Med Inform Assoc. 2024;31(6):1436-1440. doi: 10.1093/jamia/ocad258
- Seng EC, Mehdipour S, Simpson S, Gabriel RA. Tracking persistent postoperative opioid use: A proof-of-concept study demonstrating a use case for natural language processing. Reg Anesth Pain Med. 2024;49(4):241-247. doi: 10.1136/rapm-2023-104629
- Han C, Kim DW, Kim S, et al. Large-Language-Model-Based 10-Year Risk Prediction of Cardiovascular Disease: Insight from the UK Biobank Data; 2023. Available from: https:// medrxiv.org/lookup [Last accessed on 2024 Sep 03]. doi: 10.1101/2023.05.22.23289842
- Schopow N, Osterhoff G, Baur D. Applications of the natural language processing tool ChatGPT in clinical practice: Comparative study and augmented systematic review. JMIR Med Inform. 2023;11:e48933. doi: 10.2196/48933
- Wang C, Ong J, Wang C, Ong H, Cheng R, Ong D. Potential for GPT technology to optimize future clinical decision-making using retrieval-augmented generation. Ann Biomed Eng. 2024;52(5):1115-1118. doi: 10.1007/s10439-023-03327-6
- Zhang A, Yuksekgonul M, Guild J, et al. ChatGPT Exhibits Gender and Racial Biases in Acute Coronary Syndrome Management. arXiv; 2023. Available from: https://arxiv.org/ abs/2311.14703 [Last accessed on 2024 Sep 03]. doi: 10.48550/arXiv.2311.14703
- Bridgeman PJ, Bridgeman MB, Barone J. Burnout syndrome among healthcare professionals. Am J Health Syst Pharm. 2018;75(3):147-152. doi:10.2146/ajhp170460
- Priyanshu A, Vijay S, Kumar A, Naidu R, Mireshghallah F. Are chatbots ready for privacy-sensitive applications? An investigation into input regurgitation and prompt-induced sanitization. Cureus. 2023;15(11):e68134. doi: 10.7759/cureus.68134
- van Kolfschooten H, van Oirschot J. The EU Artificial intelligence act (2024): Implications for healthcare. Health Policy. 2024;149:105152. doi: 10.1016/j.healthpol.2024.105152