Machine learning-driven prediction of EBNA1 inhibitors against Epstein–Barr virus in nasopharyngeal carcinoma
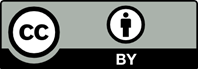
Nasopharyngeal carcinoma (NPC), particularly prevalent in regions such as Malaysia, is a significant health concern often linked to Epstein-Barr virus (EBV) infection. The EBV nuclear antigen 1 (EBNA1), crucial for EBV survival and NPC tumorigenicity, has emerged as a potential therapeutic target for EBV-positive NPC. In this study, we utilized quantitative structure-activity relationship (QSAR) models to predict potential inhibitors of EBNA1. These models were developed based on the molecular fingerprints of known EBNA1 inhibitors, using both classification and regression approaches. Our QSAR classification models demonstrated consistently high precision, recall, F1 score, and accuracy scores across the training set. The top-performing models, constructed using logistic regression algorithms, achieved perfect precision scores of 1.000 in the test set evaluation. These models’ recall, F1 score, and accuracy scores were 0.571, 0.727, and 0.667, respectively. On the other hand, the best-performing model among the regression models was built using the sequential minimal optimization regression algorithm, achieving a correlation coefficient of 0.703. The mean absolute error and root mean square error of this QSAR regression model were 0.173 and 0.217, respectively, whereas the relative absolute error was 0.689. We screened the enamine advanced compound library using this regression model to predict compounds with potential EBNA1 inhibitory effects. This led to the identification of the top 10 compounds with the most promising predicted EBNA1 inhibitory properties.
- Singh N, Vayer P, Tanwar S, Poyet JL, Tsaioun K, Villoutreix BO. Drug discovery and development: Introduction to the general public and patient groups. Front Drug Discov. 2023;3:1201419. doi: 10.3389/fddsv.2023.1201419
- Sun J, Warden AR, Ding X. Recent advances in microfluidics for drug screening. Biomicrofluidics. 2019;13(6):061503. doi: 10.1063/1.5121200
- Thorne N, Auld DS, Inglese J. Apparent activity in high-throughput screening: Origins of compound-dependent assay interference. Curr Opin Chem Biol. 2010;14(3):315-324. doi: 10.1016/j.cbpa.2010.03.020
- Hansch C, Fujita T. p-σ-π analysis. A method for the correlation of biological activity and chemical structure. J Am Chem Soc. 1964;86(8):1616-1626. doi: 10.1021/ja01062a035
- Chatterjee A. 27 - Computational methods and tools for sustainable and green approaches in drug discovery. In: Banik BK, editor. Green Approaches in Medicinal Chemistry for Sustainable Drug Design. Amsterdam: Elsevier; 2020. p. 965-988. doi: 10.1016/B978-0-12-817592-7.00027-7
- Gupta S, Basant N, Singh KP. Nonlinear QSAR modeling for predicting cytotoxicity of ionic liquids in leukemia rat cell line: An aid to green chemicals designing. Environ Sci Pollut Res. 2015;22:12699-12710. doi: 10.1007/s11356-015-4526-3
- Gomes MN, Braga RC, Grzelak EM, et al. QSAR-driven design, synthesis and discovery of potent chalcone derivatives with antitubercular activity. Eur J Med Chem. 2017;137:126-138. doi: 10.1016/j.ejmech.2017.05.026
- Lian W, Fang J, Li C, Pang X, Liu AL, Du GH. Discovery of influenza a virus neuraminidase inhibitors using support vector machine and Naïve Bayesian models. Mol Divers. 2016;20(2):439-451. doi: 10.1007/s11030-015-9641-z
- Luo M, Wang XS, Roth BL, Golbraikh A, Tropsha A. Application of quantitative structure-activity relationship models of 5-HT1A receptor binding to virtual screening identifies novel and potent 5-HT1A ligands. J Chem Inf Model. 2014;54(2):634-647. doi: 10.1021/ci400460q
- Zhang L, Fourches D, Sedykh A, et al. Discovery of novel antimalarial compounds enabled by QSAR-based virtual screening. J Chem Inf Model. 2013;53(2):475-492. doi: 10.1021/ci300421n
- Kamano Y, Yamashita A, Nogawa T, et al. QSAR evaluation of the Ch’an Su and related bufadienolides against the colchicine-resistant primary liver carcinoma cell line PLC/ PRF/5. J Med Chem. 2002;45(25):5440-5447. doi: 10.1021/jm0202066
- Avram S, Stan MS, Udrea AM, Buiu C, Boboc AA, Mernea M. 3D-ALMOND-QSAR models to predict the antidepressant effect of some natural compounds. Pharmaceutics. 2021;13(9):1449. doi: 10.3390/pharmaceutics13091449
- Ravichandran V, Jain A, Mourya V, Agrawal RK. Prediction of anti-HIV activity and cytotoxicity of pyrimidinyl and triazinyl amines: A QSAR study. Chem Pap. 2008;62:596-602. doi: 10.2478/s11696-008-0072-5
- Yuan H, Parrill AL. QSAR studies of HIV-1 integrase inhibition. Bioorg Med Chem. 2002;10(12):4169-4183. doi: 10.1016/s0968-0896(02)00332-2
- Bray F, Ferlay J, Soerjomataram I, Siegel RL, Torre LA, Jemal A. Global cancer statistics 2018: GLOBOCAN estimates of incidence and mortality worldwide for 36 cancers in 185 countries. CA Cancer J Clin. 2018;68(6):394-424. doi: 10.3322/caac.21492
- Azizah AM, Hashimah B, Nirmal K, et al. Malaysia National Cancer Registry Report (MNCR). Putrajaya, Malaysia: National Cancer Institute, Ministry of Health; 2019.
- Devi BCR, Pisani P, Tang TS, Parkin DM. High incidence of nasopharyngeal carcinoma in native people of Sarawak, Borneo Island. Cancer Epidemiol Biomarkers Prev. 2004;13(3):482-486.
- Yates J, Warren N, Reisman D, Sugden B. A cis-acting element from the Epstein-Barr viral genome that permits stable replication of recombinant plasmids in latently infected cells. Proc Natl Acad Sci U S A. 1984;81(12):3806-3810. doi: 10.1073/pnas.81.12.3806
- Frappier L, O’Donnell M. Epstein-barr nuclear antigen 1 mediates a DNA loop within the latent replication origin of Epstein-Barr virus. Proc Natl Acad Sci U S A. 1991;88(23):10875-10879. doi: 10.1073/pnas.88.23.10875
- Chaudhuri B, Xu H, Todorov I, Dutta A, Yates JL. Human DNA replication initiation factors, ORC and MCM, associate with oriP of Epstein-Barr virus. Proc Natl Acad Sci U S A. 2001;98(18):10085-10089. doi: 10.1073/pnas.181347998
- Harris A, Young BD, Griffin BE. Random association of Epstein-Barr virus genomes with host cell metaphase chromosomes in Burkitt’s lymphoma-derived cell lines. J Virol. 1985;56(1):328-332. doi: 10.1128/JVI.56.1.328-332.1985
- Petti L, Sample C, Kieff E. Subnuclear localization and phosphorylation of Epstein-Barr virus latent infection nuclear proteins. Virology. 1990;176(2):563-574. doi: 10.1016/0042-6822(90)90027-o
- Lee MA, Diamond ME, Yates JL. Genetic evidence that EBNA-1 is needed for efficient, stable latent infection by Epstein-Barr virus. J Virol. 1999;73(4):2974-2982. doi: 10.1128/jvi.73.4.2974-2982.1999
- Lupton S, Levine AJ. Mapping genetic elements of Epstein- Barr virus that facilitate extrachromosomal persistence of Epstein-Barr virus-derived plasmids in human cells. Mol Cell Biol. 1985;5:2533-2542. doi: 10.1128/mcb.5.10.2533-2542.1985
- Wood VHJ, O’Neil JD, Wei W, Stewart SE, Dawson CW, Young LS. Epstein-Barr virus-encoded EBNA1 regulates cellular gene transcription and modulates the STAT1 and TGFbeta signaling pathways. Oncogene. 2007;26(28):4135-4147. doi: 10.1038/sj.onc.1210496
- Valentine R, Dawson CW, Hu C, et al. Epstein-Barr virus-encoded EBNA1 inhibits the canonical NF-κB pathway in carcinoma cells by inhibiting IKK phosphorylation. Mol Cancer. 2010;9:1. doi: 10.1186/1476-4598-9-1
- Sivachandran N, Sarkari F, Frappier L. Epstein-Barr nuclear antigen 1 contributes to nasopharyngeal carcinoma through disruption of PML nuclear bodies. PLoS Pathog. 2008;4(10):e1000170. doi: 10.1371/journal.ppat.1000170
- Scaglioni PP, Yung TM, Cai LF, et al. A CK2-dependent mechanism for degradation of the PML tumor suppressor. Cell. 2006;126(2):269-283. doi: 10.1016/j.cell.2006.05.041
- Sivachandran N, Cao JY, Frappier L. Epstein-Barr virus nuclear antigen 1 Hijacks the host kinase CK2 to disrupt PML nuclear bodies. J Virol. 2010;84(21):11113-11123. doi: 10.1128/JVI.01183-10
- Holowaty MN, Zeghouf M, Wu H, et al. Protein profiling with Epstein-Barr nuclear antigen-1 reveals an interaction with the herpesvirus-associated ubiquitin-specific protease HAUSP/USP7. J Biol Chem. 2003;278(32):29987-29994. doi: 10.1074/jbc.M303977200
- Gruhne B, Sompallae R, Marescotti D, Kamranvar SA, Gastaldello S, Masucci MG. The Epstein-Barr virus nuclear antigen-1 promotes genomic instability via induction of reactive oxygen species. Proc Natl Acad Sci U S A. 2009;106(7):2313-2318. doi: 10.1073/pnas.0810619106
- Cao JY, Mansouri S, Frappier L. Changes in the nasopharyngeal carcinoma nuclear proteome induced by the EBNA1 protein of Epstein-Barr virus reveal potential roles for EBNA1 in metastasis and oxidative stress responses. J Virol. 2012;86(1):382-394. doi: 10.1128/JVI.05648-11
- Gianti E, Messick TE, Lieberman PM, Zauhar RJ. Computational analysis of EBNA1 “druggability” suggests novel insights for Epstein-Barr virus inhibitor design. J Comput Aided Mol Des. 2016;30(4):285-303. doi: 10.1007/s10822-016-9899-y
- Bouckaert RR, Frank E, Hall M. WEKA Manual for Version 3-9-1. Hamilton, New Zealand: University of Waikato; 2016. p. 1-341.
- Holmes G, Donkin A, Witten IH. Weka: A Machine Learning Workbench. In: Proceedings of ANZIIS’94-Australian New Zealand Intelligent Information Systems Conference. IEEE; 1994. p. 357-361.
- Kononenko I, Hong SJ. Attribute selection for modelling. Future Gener Comput Syst. 1997;13(2):181-195. doi: 10.1016/S0167-739X(97)81974-7
- Hall MA. Correlation-based Feature Subset selection for Machine Learning. Thesis Submitted in Partial Fulfilment of the Requirements of the Degree of Doctor of Philosophy at the University of Waikato; 1988.
- Hall M, Guetlein M. BestFirst; 2019. Available from: https:// weka.sourceforge.io/doc.dev/weka/attributeselection/ bestfirst.html [Last accessed on 2024 Nov 07].
- Hall M. GreedyStepwise; 2019. Available from: https:// weka.sourceforge.io/doc.dev/weka/attributeselection/ greedystepwise.html [Last accessed on 2024 Nov 07].
- Vujović Ž. Classification model evaluation metrics. Int J Adv Comput Sci Appl. 2021;12(6):599-606. doi: 10.14569/IJACSA.2021.0120670
- Ratner B. The correlation coefficient: Its values range between +1/−1, or do they? Journal of Target Meas Anal Mark. 2009;17(2):139-142. doi: 10.1057/jt.2009.5
- Tatachar AV. Comparative assessment of regression models based on model evaluation metrics. Int J Innov Technol Explor Eng. 2021;8(9):853-860.
- Gill J, Moullet M, Martinsson A, et al. Evaluating the performance of machine-learning regression models for pharmacokinetic drug-drug interactions. CPT Pharmacometrics Syst Pharmacol. 2023;12(1):122-134. doi: 10.1002/psp4.12884
- Damodharan S, Reddy SV, Sarojamma B. WEKA models for rainfall data. Int J Emerg Technol Innovat Res. 2022;9:C111-C119.
- Tropsha A, Gramatica P, Gombar VK. The importance of being earnest: Validation is the absolute essential for successful application and interpretation of QSPR models. QSAR Comb Sci. 2003;22(1):69-77. doi: 10.1002/qsar.200390007