The role of artificial intelligence in higher medical education and the ethical challenges of its implementation
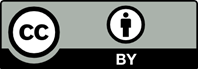
Artificial intelligence (AI) is penetrating higher medical education; however, its adoption remains low. A PRISMA-S search of the Web of Science database from 2020 to 2024, utilizing the search terms “artificial intelligence,” “medicine,” “education,” and “ethics,” reveals this trend. Four key areas of AI application in medical education are examined for their potential benefits: Educational support (such as personalized distance education), radiology (diagnostics), virtual reality (VR) (visualization and simulations), and generative text engines (GenText), such as ChatGPT (from the production of notes to syllabus design). However, significant ethical risks accompany AI adoption, and specific concerns are linked to each of these four areas. While AI is recognized as an important support tool in medical education, its slow integration hampers learning and diminishes student motivation, as evidenced by the challenges in implementing VR. In radiology, data-intensive training is hindered by poor connectivity, particularly affecting learners in developing countries. Ethical risks, such as bias in datasets (whether intentional or unintentional), need to be highlighted within educational programs. Students must be informed of the possible motivation behind the introduction of social and political bias in datasets, as well as the profit motive. Finally, the ethical risks accompanying the use of GenText are discussed, ranging from student reliance on instant text generation for assignments, which can hinder the development of critical thinking skills, to the potential danger of relying on AI-generated learning and treatment plans without sufficient human moderation.
- Dolega-Dolegowski D, Proniewska K, Dolega- Dolegowska M, et al. Application of holography and augmented reality based technology to visualize the internal structure of the dental root – a proof of concept. Head Face Med. 2022;18(1):12. doi: 10.1186/s13005-022-00307-4
- Baker RS, Hawn A. Algorithmic bias in education. Int J Artif Intell Educ. 2022;32:1052-1092. doi: 10.1007/s40593-021-00285-9
- Albahri AS, Duhaim AM, Fadhel MA, et al. A systematic review of trustworthy and explainable artificial intelligence in healthcare: Assessment of quality, bias risk, and data fusion. Inform Fusion. 2023;96:156-191. doi: 10.1016/J.INFFUS.2023.03.008
- Rudnicka Z, Szczepanski J, Pregowska A. Artificial intelligence-based algorithms in medical image scan segmentation and intelligent visual content generation-a concise overview. Electronics (Basel). 2024;13(4):746. doi: 10.3390/electronics13040746
- Pregowska A, Perkins M. Artificial Intelligence in Medical Education: Technology and Ethical Risk. Available from: https://ssrn.com/abstract=4643763 [Last accessed on 2024 Oct 18]. doi: 10.2139/ssrn.4643763
- Naik N, Hameed BMZ, Shetty DK, et al. Legal and ethical consideration in artificial intelligence in healthcare: Who takes responsibility? Front Surg. 2022;9:862322. doi: 10.3389/fsurg.2022.862322
- Bae CY, Im Y, Lee J, et al. Comparison of biological age prediction models using clinical biomarkers commonly measured in clinical practice settings: AI techniques Vs. traditional statistical methods. Front Anal Sci. 2021;1:709589. doi: 10.3389/frans.2021.709589
- Hassija V, Chamola V, Mahapatra A, et al. Interpretability of black-box models: A review on explainable artificial intelligence (XAI). Cognit Comput. 2024;16:45-74. doi: 10.1007/s12559-023-10179-8
- Kunze KN, Williams RJ 3rd, Ranawat AS, et al. Artificial intelligence (AI) and large data registries: Understanding the advantages and limitations of contemporary data sets for use in AI research. Knee Surg Sports Traumatol Arthrosc. 2024;32(1):13-18. doi: 10.1002/ksa.12018
- Baniecki H, Biecek P. Adversarial attacks and defenses in explainable artificial intelligence: A survey. Information Fusion. 2023;107:102303. doi: 10.1016/j.inffus.2024.102303
- Rethlefsen ML, Kirtley S, Waffenschmidt S, et al. PRISMA-S: An extension to the PRISMA statement for reporting literature searches in systematic reviews. Syst Rev. 2021;10:39. doi: 10.1186/s13643-020-01542-z
- Lee J, Wu AS, Li D, Kulasegaram K (Mahan). Artificial intelligence in undergraduate medical education: A scoping review. Acad Med. 2021;96(11S):S62-S70. doi: 10.1097/ACM.0000000000004291
- Weidener L, Fischer M. Teaching AI ethics in medical education: A scoping review of current literature and practices. Perspect Med Educ. 2023;12:399-410. doi: 10.5334/pme.954
- Murat Civaner M, Uncu Y, Bulut F, Chalil G, Tatli A. A three-arm single blind randomised control trial of naïve medical students performing a shoulder joint clinical examination. BMC Med Educ. 2021;22;772. doi: 10.1186/s12909-022-03852-3
- Andersson J, Nyholm T, Ceberg C, et al. Artificial intelligence and the medical physics profession - a Swedish perspective. Phys Med. 2021;88:218-225. doi: 10.1016/J.EJMP.2021.07.009
- Mosch L, Fürstenau D, Brandt J, et al. The medical profession transformed by artificial intelligence: Qualitative study. Digit Health. 2022;8:20552076221143903. doi: 10.1177/20552076221143903
- Garlinska M, Osial M, Proniewska K, Pregowska A. The influence of emerging technologies on distance education. Electronics (Switzerland). 2023;12(7):1550. doi: 10.3390/electronics12071550
- Klauschen F, Dippel J, Keyl P, et al. Toward explainable artificial intelligence for precision pathology. Annu Rev Pathol Mech Dis. 2024;19:541-570. doi: 10.1146/annurev-pathmechdis
- Gordon M, Daniel M, Ajiboye A, et al. A scoping review of artificial intelligence in medical education: BEME Guide No. 84. Med Teach. 2024;46:446-470. doi: 10.1080/0142159X.2024.2314198
- Pinto Dos Santos D, Giese D, Brodehl S, et al. Medical students’ attitude towards artificial intelligence: A multicentre survey. Eur Radiol. 2019;29:1640-1646. doi: 10.1007/s00330-018-5601-1
- Chengoden R, Victor N, Huynh-The T, et al. Metaverse for healthcare: A survey on potential applications, challenges and future directions. IEEE Access. 2023;PP:1-1. doi: 10.1109/ACCESS.2023.3241628
- Musamih A, Yaqoob I, Salah K, et al. Metaverse in healthcare: Applications, challenges, and future directions. IEEE Consum Electron Mag. 2023;12(4):33-46. doi: 10.1109/MCE.2022.3223522
- Zechner O, Guirao DG, Schrom-Feiertag H, et al. Multimodal technologies and interaction NextGen training for medical first responders: Advancing mass-casualty incident preparedness through mixed reality technology. Multimodal Technol Interact. 2023;7:113. doi: 10.3390/mti7120113
- Balak N, Ganau M, Tsianaka E, Park JJ, Tiefenbach J, Demetriades AK. The role of artificial intelligence in surgical simulation. Front Med Technol. 2022;4:1076755. doi: 10.3389/fmedt.2022.1076755
- Neves CA, Tran ED, Kessler IM, Blevins NH. Fully automated preoperative segmentation of temporal bone structures from clinical CT scans. Sci Rep. 2021;11:116. doi: 10.1038/s41598-020-80619-0
- Hamabe A, Ishii M, Kamoda R, et al. Artificial intelligence-based technology to make a three-dimensional pelvic model for preoperative simulation of rectal cancer surgery using MRI. Ann Gastroenterol Surg. 2022;6:788-794. doi: 10.1002/ags3.12574
- Saricilar EC, Burgess A, Freeman A, et al. A pilot study of the use of artificial intelligence with high-fidelity simulations in assessing endovascular procedural competence independent of a human examiner. ANZ J Surg. 2023;93:1525-1531. doi: 10.1111/ans.18484
- Mirchi IN, Bissonnette V, Yilmaz R, Ledwos N, Winkler- Schwartz A, Del Maestro RF. The Virtual Operative Assistant: An explainable artificial intelligence tool for simulation-based training in surgery and medicine. PLoS One. 2020;15:e0229596. doi: 10.1371/journal.pone.0229596
- Proniewska K, Dolega-Dolegowski D, Kolecki R, Osial M, Pregowska A. The 3D operating room with unlimited perspective change and remote support. In: Applications of Augmented Reality - Current State of the Art [Working Title]. London: Intechopen; 2023. doi: 10.5772/intechopen.1002252
- Winkler-Schwartz A, Bissonnette V, Mirchi N, et al. Artificial intelligence in medical education: Best practices using machine learning to assess surgical expertise in virtual reality simulation. J Surg Educ. 2019;76(6):1681-1690. doi: 10.1016/J.JSURG.2019.05.015
- Natheir S, Christie S, Yilmaz R, et al. Utilizing artificial intelligence and electroencephalography to assess expertise on a simulated neurosurgical task. Comput Biol Med. 2023;152:106286. doi: 10.1016/j.compbiomed.2022.106286
- Wölfel M, Taecharungroj V. “What Can ChatGPT Do?” Analyzing early reactions to the innovative AI Chatbot on twitter. Big Data Cogn Comput. 2023;7:35. doi: 10.3390/bdcc7010035
- Alkaissi H, Mcfarlane SI. Artificial hallucinations in ChatGPT: Implications in scientific writing. Cureus. 2023;15:e35179. doi: 10.7759/cureus.35179
- Available from: https://lawlibguides.sandiego.edu/c. php?g=1317323&p=9686671 [Last accessed on 2024 Oct 18].
- Beilby K, Hammarberg K. ChatGPT: A reliable fertility decision-making tool? Hum Reprod. 2024;39:443-447. doi: 10.1093/humrep/dead272
- Funk PF, Hoch CC, Manuel F, et al. Citation: ChatGPT’s response consistency: A study on repeated queries of medical examination questions. J Investig Health Psychol Educ. 2024;14:657-668. doi: 10.3390/ejihpe14030043
- Mu Y, He D. The potential applications and challenges of ChatGPT in the medical field. Int J Gen Med. 2024;17:817-826. doi: 10.2147/ijgm.s456659
- Carr SE, Canny BJ, Wearn A, et al. Twelve tips for medical students experiencing an interruption in their academic progress. Med Teach. 2022;44(10):1081-1086. doi: 10.1080/0142159X.2021.1921134
- Shen Y, Heacock L, Elias J, et al. ChatGPT and other large language models are double-edged swords. Radiology. 2023;307(2):e230163. doi: 10.1148/radiol.230163
- Lee H. The rise of ChatGPT: Exploring its potential in medical education. 2023. doi: 10.1002/ase.2270
- Saleem N, Mufti T, Sohail SS, Madsen DØ. ChatGPT as an innovative heutagogical tool in medical education. Cogent Education. 2024;11(1). doi: 10.1080/2331186X.2024.2332850
- Emir B, Yurdem T, Ozel T, et al. Artificial intelligence readiness status of medical faculty students. Konuralp Med J. 2024;16(1):88-95. doi: 10.18521/ktd.1387826
- Rezazadeh H, Ahmadipour H, Salajegheh M. Psychometric evaluation of Persian version of medical artificial intelligence readiness scale for medical students. BMC Med Educ. 2023;23(1):527. doi: 10.1186/s12909-023-04516-6
- Dennett D. The Self as a Center of Narrative Gravity. Self and Consciousness: Multiple Perspectives. Hillsdale, NJ: Lawrence Erlbaum; 1992. p. 53.
- Acharya V, Padhan P, Bahinipati J, et al. Artificial intelligence in medical education. J Integr Med Res. 2023;1(3):87-91.
- Katznelson G, Gerke S. The need for health AI ethics in medical school education. Adv Health Sci Educ. 2021;26:1447-1458. doi: 10.1007/s10459-021-10040-3
- Ötleş E, James CA, Lomis KD, Woolliscroft JO. Teaching artificial intelligence as a fundamental toolset of medicine. Cell Rep Med. 2022;3(12):100824. doi: 10.1016/J.XCRM.2022.100824
- Zarei M, Eftekhari Mamaghani H, Abbasi A, Hosseini MS. Application of artificial intelligence in medical education: A review of benefits, challenges, and solutions. Med Clín Práct. 2024;7(2):100422. doi: 10.1016/J.MCPSP.2023.100422
- Krive J, Isola M, Chang L, Patel T, Anderson M, Sreedhar R. Grounded in reality: Artificial intelligence in medical education. JAMIA Open. 2023;6:ooad037. doi: 10.1093/jamiaopen/ooad037
- Piorkowski A, Obuchowicz R, Najjar R. Redefining radiology: A review of artificial intelligence integration in medical imaging. Diagnostics (Basel). 2023;13:2760. doi: 10.3390/diagnostics13172760
- Brady AP, Allen B, Chong J, et al. STATEMENT Open Access. J Med Imaging Radiat Oncol. 15. doi: 10.1186/s13244-023-01541-3
- Choudhury A, Elkefi S. Acceptance, initial trust formation, and human biases in artificial intelligence: Focus on clinicians. Front Digit Health. 2022;4:966174. doi: 10.3389/fdgth.2022.966174
- Pagano TP, Loureiro RB, Lisboa FVN, et al. Bias and unfairness in machine learning models: A systematic review on datasets, tools, fairness metrics, and identification and mitigation methods. Big Data Cogn. Comput. 2023;7:15. doi: 10.3390/bdcc7010015
- Pessach D, Shmueli E. A review on fairness in machine learning. ACM Comput Surv. 2022;55(3):51. doi: 10.1145/3494672
- Rudnicka Z, Proniewska K, Perkins M, Pregowska A. Cardiac healthcare digital twins supported by artificial intelligence-based algorithms and extended reality-a systematic review. Electronics (Basel). 2024;13(5):866. doi: 10.3390/electronics13050866
- Ueda D, Kakinuma T, Fujita S, et al. Fairness of artificial intelligence in healthcare: Review and recommendations. Jpn J Radiol. 2024;42:3-15. doi: 10.1007/s11604-023-01474-3
- Shen D, Liu T. Grand challenges in AI in radiology. Front Radiol. 2021;1:629992. doi: 10.3389/fradi.2021.629992
- Park Y, Hu J. Bias in artificial intelligence: Basic primer. Clin J Am Soc Nephrol. 2023;18(3):394-396. doi: 10.2215/CJN.0000000000000078
- Meyers PM, Gabelloni M, Wagner M, Schweitzer M, Khosravi P. Artificial intelligence in neuroradiology: A scoping review of some ethical challenges. Front Radiol. 2023;3:1149461. doi: 10.3389/fradi.2023.1149461
- Bell LC, Shimron E. Sharing data is essential for the future of AI in medical imaging. Radiol Artif Intell. 2023;6(1):e230337. doi: 10.1148/ryai.230337
- Bernstein MH, Atalay MK, Dibble EH, et al. Can incorrect artificial intelligence (AI) results impact radiologists, and if so, what can we do about it? A multi-reader pilot study of lung cancer detection with chest radiography Chest radiograph DSI Data Science Institute FN False negative FP False positive GLMM Generalized linear mixed modeling PACS Picture archiving and communication system. Eur Radiol. 2023;33:8263-8269. doi: 10.1007/s00330-023-09747-1
- Eltawil FA, Atalla M, Boulos E, Amirabadi A, Tyrrell PN. Analyzing barriers and enablers for the acceptance of artificial intelligence innovations into radiology practice: A scoping review. Tomography. 2023;9(4):1443-1455. doi: 10.3390/tomography9040115
- Kelly BS, Quinn C, Belton N, et al. Cybersecurity considerations for radiology departments involved with artificial intelligence. Eur Radiol. 2023;33:8833-8841. doi: 10.1007/s00330-023-09860-1
- Available from: https://www.fda.gov/medical-devices/ software-medical-device-samd/artificial-intelligence-and-machine-learning-aiml-enabled-medical-devices [Last accessed on 2024 Oct 08].
- Available from: https://www.gov.uk/government/ organisations/medicines-and-healthcare-products-regulatory-agency[Last accessed on 2024 Oct 08].
- UK Digital Health - the Future of Software as a Medical Device. Available from: https://www.gov.uk/government/ publications/software-and-ai-as-a-medical-device-change-programme/software-and-ai-as-a-medical-device-change-programme-roadmap [Last accessed on 2024 Oct 18].
- Khazane H, Ridouani M, Salahdine F, Kaabouch N. A holistic review of machine learning adversarial attacks in IoT networks. Future Internet. 2024;16:32. doi: 10.3390/fi16010032
- Tools M, Tsai MJ, Lin PY. Medical images under tampering. Multimed Tools Appl. 2024;83:65407-65439. doi: 10.1007/s11042-023-17968-1
- Prajapati JB, Kumar A, Singh S, et al. Artificial intelligence-assisted generative pretrained transformers for applications of ChatGPT in higher education among graduates. SN Soc Sci. 2024;4:19. doi: 10.1007/s43545-023-00818-0
- Narayanan S, Ramakrishnan R, Durairaj E, Das A. Artificial intelligence revolutionizing the field of medical education. Cureus. 2023;15(11):e49604. doi: 10.7759/cureus.49604
- Lie SS, Helle N, Sletteland NV, Dubland Vikman M, Bonsaksen T. Implementation of virtual reality in health professions education: Scoping Review. JMIR Res Protoc. 2022;11:e37222. doi: 10.2196/37222
- Dhar E, Upadhyay U, Huang Y, et al. A scoping review to assess the effects of virtual reality in medical education and clinical care. Digit Health. 2023;9:20552076231158022. doi: 10.1177/20552076231158022
- Kim HY, Kim EY, Dominguez-Morales M, Billis A, Kim HY, Kim EY. Effects of medical education program using virtual reality: A systematic effects of medical education program using virtual reality: A systematic review and meta-analysis. Int J Environ Res Public Health. 2023;20:3895. doi: 10.3390/ijerph20053895
- Leng L. Challenge, integration, and change: ChatGPT and future anatomical education. Med Educ Online. 2024;29(1):2304973. doi: 10.1080/10872981.2024.2304973
- Pedram S, Kennedy G, Sanzone S. Assessing the validity of VR as a training tool for medical students. Virtual Real. 2024;28:15. doi: 10.1007/s10055-023-00912-x
- Mergen M, Meyerheim M, Graf N. Reviewing the current state of virtual reality integration in medical education - a scoping review protocol. Syst Rev. 2023;12(1):97. doi: 10.1186/s13643-023-02266-6
- Mergen M, Meyerheim M, Graf N. Towards integrating virtual reality into medical curricula: A single center student survey. Educ Sci. 2023;13:477. doi: 10.3390/educsci13050477
- Available from: https://www.technologyreview. com/2023/08/07/1077324/ai-language-models-are-rife-with-political-biases [Last accessed on 2024 Oct 08].
- Feng Y, Chen Z, Kang Z, et al. JailbreakLens: Visual Analysis of Jailbreak Attacks against Large Language Models. doi: 10.48550/arXiv.2404.08793
- Shah R, Feuillade-Montixi Q, Pour S, Tagade A, Casper S, Rando J. Scalable and Transferable Black-Box Jailbreaks for Language Models via Persona Modulation. doi: 10.48550/arXiv.2311.03348
- Eysenbach G. The role of ChatGPT, generative language models, and artificial intelligence in medical education: A conversation with ChatGPT and a call for papers. JMIR Med Educ. 2023;9:e46885. doi: 10.2196/46885
- Májovský M, Černý M, Kasal M, Komarc M, Netuka D. Artificial intelligence can generate fraudulent but authentic-looking scientific medical articles: Pandora’s box has been opened. J Med Internet Res. 2023;25:e46924. doi: 10.2196/46924
- Choi EPH, Lee JJ, Ho MH, Kwok JYY, Lok KYW. Chatting or cheating? The impacts of ChatGPT and other artificial intelligence language models on nurse education. Nurse Educ Today. 2023;125:105796. doi: 10.1016/J.NEDT.2023.105796
- Tsegay SM, Imafuku R, Alam F, Lim MA, Zulkipli IN. Integrating AI in medical education: Embracing ethical usage and critical understanding. Front Med. 2023;10:1279707. doi: 10.1080/14779072.2023.2223978
- Kitamura FC. ChatGPT is shaping the future of medical writing but still requires Human Judgment. Radiology. 2023;307(2):e230171. doi: 10.1148/radiol.230171
- Filgueiras F. Artificial intelligence and education governance. Educ Citizsh Soc Justice. 2023. doi: 10.1177/17461979231160674
- Taran S, Adhikari NKJ, Fan E. Falsifiability in medicine: What clinicians can learn from Karl Popper. Intensive Care Med. 2021;47:1054-1056. doi: 10.1007/s00134-021-06432-z
- Abdelhady AM, Davis CR. Plastic surgery and artificial intelligence: How ChatGPT improved operation note accuracy, time, and education. Mayo Clin Proc Digit Health. 2023;1(3):299-308. doi: 10.1016/J.MCPDIG.2023.06.002
- Giannos P, Delardas O. Performance of ChatGPT on UK standardized admission tests: Insights from the BMAT, TMUA, LNAT, and TSA examinations. JMIR Med Educ. 2023;9:e47737. doi: 10.2196/47737
- Gritti MN, Hussain A, Farid P, Morgan CT. Progression of an artificial intelligence chatbot (ChatGPT) for pediatric cardiology educational knowledge assessment. Pediatr Cardiol. 2024;45:309-313. doi: 10.1007/s00246-023-03385-6
- Rizzo MG, Cai N, Constantinescu D. The performance of ChatGPT on orthopaedic in-service training exams: A comparative study of the GPT-3.5 turbo and GPT-4 models in orthopaedic education. J Orthop. 2024;50:70-75. doi: 10.1016/J.JOR.2023.11.056
- Davies NP, Wilson R, Winder MS, et al. ChatGPT sits the DFPH exam: Large language model performance and potential to support public health learning. BMC Med Educ. 2024;24(1):57. doi: 10.1186/s12909-024-05042-9
- Sevgi UT, Erol G, Doğruel Y, et al. The role of an open artificial intelligence platform in modern neurosurgical education: A preliminary study. Neurosurg Rev. 1998;46:86. doi: 10.1007/s10143-023-01998-2