Does improving diagnostic accuracy increase artificial intelligence adoption? A public acceptance survey using randomized scenarios of diagnostic methods
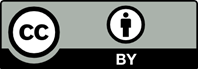
This study examines the acceptance of artificial intelligence (AI)-based diagnostic alternatives compared to traditional biological testing through a randomized scenario experiment in the domain of neurodegenerative diseases (NDs). A total of 3225 pairwise choices of ND risk-prediction tools were offered to participants, with 1482 choices comparing AI with the biological saliva test and 1743 comparing AI+ with the saliva test (with AI+ using digital consumer data, in addition to electronic medical data). Overall, only 36.68% of responses showed preferences for AI/AI+ alternatives. Stratified by AI sensitivity levels, acceptance rates for AI/AI+ were 35.04% at 60% sensitivity and 31.63% at 70% sensitivity, and increased markedly to 48.68% at 95% sensitivity (p <0.01). Similarly, acceptance rates by specificity were 29.68%, 28.18%, and 44.24% at 60%, 70%, and 95% specificity, respectively (P < 0.01). Notably, AI consistently garnered higher acceptance rates (45.82%) than AI+ (28.92%) at comparable sensitivity and specificity levels, except at 60% sensitivity, where no significant difference was observed. These results highlight the nuanced preferences for AI diagnostics, with higher sensitivity and specificity significantly driving acceptance of AI diagnostics.
- Reyna MA, Nsoesie EO, Clifford GD. Rethinking algorithm performance metrics for artificial intelligence in diagnostic medicine. JAMA. 2022;328(4):329-330. doi: 10.1001/jama.2022.10561
- Aggarwal R, Sounderajah V, Martin G, et al. Diagnostic accuracy of deep learning in medical imaging: A systematic review and meta-analysis. NPJ Digit Med. 2021;4(1):65. doi: 10.1038/s41746-021-00438-z
- Laux J, Wachter S, Mittelstadt B. Trustworthy artificial intelligence and the European Union AI act: On the conflation of trustworthiness and acceptability of risk. Regul Gov. 2024;18(1):3-32. doi: 10.1111/rego.12512
- Choung H, David P, Ross A. Trust in AI and its role in the acceptance of AI technologies. Int J Hum Comput Interact. 2023;39(9):1727-1739. doi: 10.1080/10447318.2022.2050543
- Nadarzynski T, Miles O, Cowie A, Ridge D. Acceptability of artificial intelligence (AI)-led chatbot services in healthcare: A mixed-methods study. Digit Health. 2019;5:2055207619871808. doi: 10.1177/205520761987180
- Esmaeilzadeh P. Use of AI-based tools for healthcare purposes: A survey study from consumers’ perspectives. BMC Med Inform Decis Mak. 2020;20:1-19. doi: 10.1186/s12911-020-01191-1
- Floruss J, Vahlpahl N. Artificial Intelligence in Healthcare: Acceptance of AI-based Support Systems by Healthcare Professionals. Jönköping University. Master Thesis; 2020. Available from: https://www.diva-portal.org/smash/get/ diva2:1433298/fulltext01.pdf [Last accessed on 2024 Oct 11].
- Lambert SI, Madi M, Sopka S, et al. An integrative review on the acceptance of artificial intelligence among healthcare professionals in hospitals. NPJ Digit Med. 2023;6(1):111. doi: 10.1038/s41746-023-00874-z
- De Bekker‐Grob EW, Ryan M, Gerard K. Discrete choice experiments in health economics: A review of the literature. Health Econ. 2012;21(2):145-172. doi: 10.1002/hec.1697
- Szinay D, Cameron R, Naughton F, Whitty JA, Brown J, Jones A. Understanding uptake of digital health products: Methodology tutorial for a discrete choice experiment using the bayesian efficient design. J Med Internet Res. 2021;23(10):e32365. doi: 10.2196/32365
- Clark MD, Determann D, Petrou S, Moro D, De Bekker-Grob EW. Discrete choice experiments in health economics: A review of the literature. Pharmacoeconomics. 2014;32:883-902. doi: 10.1007/s40273-014-0170-x
- Alawode DO, Heslegrave AJ, Ashton NJ, et al. Transitioning from cerebrospinal fluid to blood tests to facilitate diagnosis and disease monitoring in Alzheimer’s disease. J Int Med. 2021;290(3):583-601. doi: 10.1111/joim.13332
- Greenwood PE, Nikulin MS. A Guide to Chi-squared Testing. Vol. 280. United States: John Wiley and Sons; 1996.
- Campbell I. Chi‐Squared and Fisher-Irwin tests of two‐by‐two tables with small sample recommendations. Stat Med. 2007;26(19):3661-3675. doi: 10.1002/sim.2832
- Freeman D, Lambe S, Yu LM, et al. Injection fears and COVID-19 vaccine hesitancy. Psychol Med. 2023;53(4):1185-1195. doi: 10.1017/S0033291721002609
- McLenon J, Rogers MA. The fear of needles: A systematic review and meta‐analysis. J Adv Nurs. 2019;75(1):30-42. doi: 10.1111/jan.13818
- Von Wedel P, Hagist C. Physicians’ preferences and willingness to pay for artificial intelligence-based assistance tools: A discrete choice experiment among german radiologists. BMC Health Serv Res. 2022;22(1):398. doi: 10.1186/s12913-022-07769-x