Artificial intelligence for ophthalmic drug discovery and development: Capabilities, applications, and challenges
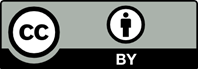
The integration of artificial intelligence (AI) into ophthalmic drug discovery and development presents transformative opportunities to address the inherent complexities and challenges of creating targeted therapies for eye diseases. The ability of AI to process vast datasets can facilitate the discovery of novel drug candidates, improve predictions of drug efficacy and safety, and streamline the drug development pipeline. Applications can range from enhancing target identification and compound screening to refining predictive toxicology. However, challenges such as data limitations, computational demands, model interpretability, and ethical considerations remain. Despite these hurdles, the integration of AI with emerging technologies and its potential to optimize clinical trials signifies a new era of innovation in ophthalmology, emphasizing its critical role in addressing current challenges and advancing therapeutic development. In this paper, we explore the role of AI in ophthalmic drug discovery, highlighting its potential to address critical challenges in the field and delineating its impact across various stages of drug development.
- Wang N, Zhang Y, Wang W, et al. How can machine learning and multiscale modeling benefit ocular drug development? Adv Drug Deliv Rev. 2023;196:114772. doi: 10.1016/j.addr.2023.114772
- Paul D, Sanap G, Shenoy S, Kalyane D, Kalia K, Tekade RK. Artificial intelligence in drug discovery and development. Drug Discov Today. 2021;26(1):80-93. doi: 10.1016/j.drudis.2020.10.010
- Pun FW, Ozerov IV, Zhavoronkov A. AI-powered therapeutic target discovery. Trends Pharmacol Sci. 2023;44(9):561-572. doi: 10.1016/j.tips.2023.06.010
- Crampon K, Giorkallos A, Deldossi M, Baud S, Steffenel LA. Machine-learning methods for ligand-protein molecular docking. Drug Discov Today. 2022;27(1):151-164. doi: 10.1016/j.drudis.2021.09.007
- Wang MWH, Goodman JM, Allen TEH. Machine learning in predictive toxicology: Recent applications and future directions for classification models. Chem Res Toxicol. 2021;34(2):217-239. doi: 10.1021/acs.chemrestox.0c00316
- Yang S, Kar S. Application of artificial intelligence and machine learning in early detection of adverse drug reactions (ADRs) and drug-induced toxicity. Artif Intell Chem. 2023;1(2):100011. doi: 10.1016/j.aichem.2023.100011
- Hsueh HT, Chou RT, Rai U, et al. Machine learning-driven multifunctional peptide engineering for sustained ocular drug delivery. Nat Commun. 2023;14(1):2509. doi: 10.1038/s41467-023-38056-w
- Remtulla R, Das SK, Levin LA. Predicting absorption-distribution properties of neuroprotective phosphine-borane compounds using in silico modeling and machine learning. Molecules. 2021;26(9):2505. doi: 10.3390/molecules26092505
- Li Z, Wang L, Wu X, et al. Artificial intelligence in ophthalmology: The path to the real-world clinic. Cell Rep Med. 2023;4(7):101095. doi: 10.1016/j.xcrm.2023.101095
- Jia Z, Chen J, Xu X, et al. The importance of resource awareness in artificial intelligence for healthcare. Nat Mach Intell. 2023;5(7):687-698. doi: 10.1038/s42256-023-00670-0
- Balagurunathan Y, Mitchell R, El Naqa I. Requirements and reliability of AI in the medical context. Phys Med. 2021;83:72-78. doi: 10.1016/j.ejmp.2021.02.024
- Nosrati H, Nosrati M. Artificial intelligence in regenerative medicine: Applications and implications. Biomimetics (Basel). 2023;8(5):442. doi: 10.3390/biomimetics8050442
- Zhang B, Zhang L, Chen Q, Jin Z, Liu S, Zhang S. Harnessing artificial intelligence to improve clinical trial design. Commun Med (Lond). 2023;3(1):191. doi: 10.1038/s43856-023-00425-3