LLMs-Healthcare: Current applications and challenges of large language models in various medical specialties
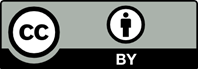
The purpose of this review is to provide a comprehensive overview of the latest advancements in utilizing large language models (LLMs) in the health-care sector, emphasizing their transformative impact across various medical domains. LLMs have become pivotal in supporting healthcare, including physicians, health-care providers, and patients. Our review provides insight into the applications of LLMs in healthcare, specifically focusing on diagnostic and treatment-related functionalities. We shed light on how LLMs are applied in cancer care, dermatology, dental care, neurodegenerative disorders, and mental health, highlighting their innovative contributions to medical diagnostics and patient care. Throughout our analysis, we explore the challenges and opportunities associated with integrating LLMs in healthcare, recognizing their potential across various medical specialties despite existing limitations. In addition, we offer an overview of handling diverse data types within the medical field.
- Min B, Ross H, Sulem E, et al. Recent advances in natural language processing via large pre-trained language models: A survey. ACM Comput Surv. 2023;56:1-40. doi: 10.1145/3605943
- Wei J, Tay Y, Bommasani R, et al. Emergent Abilities of Large Language Models. arXiv:2206.07682 [arXiv Preprint]; 2022.
- Brown T, Mann B, Ryder N, et al. Language models are few-shot learners. Adv Neural Inform Process Syst. 2020;33:1877-1901.
- Thirunavukarasu AJ, Ting DSJ, Elangovan K, Gutierrez L, Tan TF, Ting DSW. Large language models in medicine. Nat Med. 2023;29:1930-1940. doi: 10.1038/s41591-023-02448-8
- Cascella M, Montomoli J, Bellini V, Bignami E. Evaluating the feasibility of ChatGPT in healthcare: An analysis of multiple clinical and research scenarios. J Med Syst. 2023;47:33. doi: 10.1007/s10916-023-01925-4
- Sorin V, Klang E, Sklair-Levy M, et al. Large language model (ChatGPT) as a support tool for breast tumor board. NPJ Breast Cancer. 2023;9:44. doi: 10.1038/s41523-023-00557-8
- Lukac S, Dayan D, Fink V, et al. Evaluating ChatGPT as an adjunct for the multidisciplinary tumor board decision-making in primary breast cancer cases. Arch Gynecol Obstet. 2023;308:1831-1844. doi: 10.1007/s00404-023-07130-5
- Gebrael G, Sahu KK, Chigarira B, et al. Enhancing triage efficiency and accuracy in emergency rooms for patients with metastatic prostate cancer: A retrospective analysis of artificial intelligence-assisted triage using ChatGPT 4.0. Cancers (Basel). 2023;15:3717. doi: 10.3390/cancers15143717
- Rao A, Kim J, Kamineni M, et al. Evaluating GPT as an adjunct for radiologic decision making: GPT-4 Versus GPT-3.5 in a breast imaging pilot. J Am Coll Radiol. 2023;20:990-997. doi: 10.1016/j.jacr.2023.05.003
- Haver HL, Ambinder EB, Bahl M, Oluyemi ET, Jeudy J, Yi PH. Appropriateness of breast cancer prevention and screening recommendations provided by ChatGPT. Radiology. 2023;307:e230424. doi: 10.1148/radiol.230424
- Sarraju A, Bruemmer D, Van Iterson E, Cho L, Rodriguez F, Laffin L. Appropriateness of cardiovascular disease prevention recommendations obtained from a popular online chat-based artificial intelligence model. JAMA. 2023;329:842-844. doi: 10.1001/jama.2023.1044
- Schulte B. capacity of ChatGPT to identify guideline-based treatments for advanced solid tumors. Cureus. 2023;15:e37938. doi: 10.7759/cureus.37938
- Haemmerli J, Sveikata L, Nouri A, et al. ChatGPT in glioma adjuvant therapy decision making: Ready to assume the role of a doctor in the tumour board? BMJ Health Care Inform. 2023;30:e100775. doi: 10.1136/bmjhci-2023-100775
- Chen S, Kann BH, Foote MB, et al. Use of artificial intelligence chatbots for cancer treatment information. JAMA Oncol. 2023;9:1459-1462. doi: 10.1001/jamaoncol.2023.2954
- Yakupu A, Aimaier R, Yuan B, et al. The burden of skin and subcutaneous diseases: Findings from the global burden of disease study 2019. Front Public Health. 2023;11:1145513. doi: 10.3389/fpubh.2023.1145513
- Urban K, Chu S, Giesey RL, et al. Burden of skin disease and associated socioeconomic status in Asia: A cross-sectional analysis from the global burden of disease study 1990-2017. JAAD Int. 2020;2:40-50. doi: 10.1016/j.jdin.2020.10.006
- Burlando M, Muracchioli A, Cozzani E, Parodi A. Psoriasis, vitiligo, and biologic therapy: Case report and narrative review. Case Rep Dermatol. 2021;13:372-378. doi: 10.1159/000514198
- Zhou J, He X, Sun L, et al. SkinGPT-4: An interactive dermatology diagnostic system with visual large language model. 2023. medRxiv preprint.
- Dugger BN, Dickson DW. Pathology of neurodegenerative disease. Cold Spring Harb Perspect Biol. 2017;9:a028035. doi: 10.1101/cshperspect.a028035
- Koga S, Martin NB, Dickson DW. Evaluating the performance of large language models: ChatGPT and Google bard in generating differential diagnoses in clinicopathological conferences of neurodegenerative disorders. Brain Pathol. 2023. doi: 10.1111/bpa.13207
- Agbavor F, Liang H. Predicting dementia from spontaneous speech using large language models. PLOS Digit Health. 2022;1(12):e0000168. doi: 10.1371/journal.pdig.0000168
- Luz S, Haider F, de la Fuente S, Fromm D, MacWhinney B. Detecting Cognitive Decline Using Speech Only: The ADReSSo Challenge. arXiv: 210409356 [arXiv Preprint]; 2021.
- Mao C, Xu J, Rasmussen L, et al. AD-BERT: Using pre-trained language model to predict the progression from mild cognitive impairment to Alzheimer’s disease. J Biomed Inform. 2023;14:104442. doi: 10.1016/j.jbi.2023.104442
- Cai H, Huang X, Liu Z, et al. Exploring Multimodal Approaches for Alzheimer’s Disease Detection Using Patient Speech Transcript and Audio Data. arXiv:2307.02514 [arXiv Preprint]; 2023.
- Feng Y, Wang J, Gu X, Xu X, Zhang M. Large Language Models Improve Alzheimer’s Disease Diagnosis Using Multi-modality Data. arXiv:2305.19280 [arXiv Preprint]; 2023.
- Ying Y, Yang T, Zhou H. Multimodal fusion for Alzheimer’s disease recognition. Appl Intell. 2023;53:16029-16040. doi: 10.1007/s10489-022-04255-z
- Mohammad-Rahimi H, Motamedian SR, Rohban MH, et al. Deep learning for caries detection: A systematic review. J Dent. 2022;122:104115. doi: 10.1016/j.jdent.2022.104115
- Urban R, Haluzová, S, Strunga M, et al. AI-assisted CBCT data management in modern dental practice: Benefits, limitations and innovations. Electronics. 2023;12:1710. doi: 10.3390/electronics12071710
- Huang H, Zheng O, Wang D, et al. ChatGPT for shaping the future of dentistry: The potential of multi-modal large language model. Int J Oral Sci. 2023;15(1):29. doi: 10.1038/s41368-023-00239-y
- Galatzer-Levy IR, McDuff DN, Natarajan V, Karthikesalingam A, Malgaroli M. The capability of large language models to measure psychiatric functioning. 2023. arXiv preprint.
- Xu X, Yao B, Dong Y, et al. Leveraging Large Language Models for Mental Health Prediction via Online Text Data. arXiv:2307.14385 [arXiv Preprint]; 2023.
- Ma Z, Mei Y, Su Z. Understanding the benefits and challenges of using large language model-based conversational agents for mental well-being support. AMIA Annu Symp Proc. 2024;2023:1105-1114.
- Kjell O, Kjell K, Schwartz HA. AI-based Large Language Models are Ready to Transform Psychological Health Assessment; 2023. PsyArXiv.
- Wu S, Koo M, Blum, L, et al. A Comparative Study of Open-source Large Language Models, GPT-4 and Claude 2: Multiple-choice Test Taking in Nephrology. arxiv: 2308.04709 [arxiv Preprint]; 2023.
- Lahat A, Shachar E, Avidan B, Glicksberg B, Klang E. Evaluating the utility of a large language model in answering common patients’ gastrointestinal health-related questions: Are we there yet? Diagnostics (Basel). 2023;13:1950. doi: 10.3390/diagnostics13111950
- Goktas P, Karakaya G, Kalyoncu AF, Damadoglu E. Artificial intelligence Chatbots in allergy and immunology practice: Where have we been and where are we going? J Allergy Clin Immunol Pract. 2023;11:2697-2700. doi: 10.1016/j.jaip.2023.05.042
- Singhal K, Azizi S, Tu T, et al. Large language models encode clinical knowledge. Nature. 2023;620:172-180. doi: 10.1038/s41586-023-06291-2
- Wang S, Zhao Z., Ouyang, X., Wang Q, Shen D. ChatCAD: Interactive computer-aided diagnosis on medical image using large language models. 2023. arXiv:2302.07257.
- Bazi Y, Al Rahhal MM, Bashmal L, Zuair M. Vision-language model for visual question answering in medical imagery. Bioengineering. 2023;10(3):380. doi: 10.3390/bioengineering10030380
- Tan RSY, Lin Q, Low GH, et al. Inferring cancer disease response from radiology reports using large language models with data augmentation and prompting. J Am Med Inform Assoc. 2023;30:1657-1664. doi: 10.1093/jamia/ocad133
- Chen Z, Balan MM, Brown K. Language Models are Few-shot Learners for Prognostic Prediction. arXiv: 2302.12692 [arXiv Preprint]; 2023.