Natural language processing in electronic health records: A review
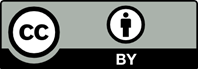
The two fundamental tasks that a physician performs during every interaction with a patient are reading and updating electronic health records (EHRs). Reading the records is necessary to gain better knowledge of a patient’s health status while updating the records is essential for creating a database for future information extraction. If a patient’s history consists of only a few records, manual reading is the best approach. However, this method may lead to overlooking important aspects of the patient’s health, which could be detrimental. Therefore, automation is required to extract important information. Natural language processing (NLP) facilitates information extraction and operates on seven different levels. In our review, we aimed to understand how NLP levels assist in extracting information. We examined articles published in PubMed and, after critical evaluation, selected 65 out of 382 identified articles that met the inclusion criteria for the final review. Among these, 47 articles were included in the final review. We found a higher number of articles on the lexical (7), semantic (30), and morphological (4) levels, while fewer articles focused on the phonetic (1), syntactic (2), discourse (2), and pragmatic (1) levels. This distribution underscores the current emphasis within the literature on the specific aspects of NLP. In conclusion, our review underscores the critical role played by NLP in extracting information from EHR, shedding light on the varied levels at which this technology operates.
- Manohara MM, Pai RG, 2021, Standard electronic health record (EHR) framework for Indian healthcare system. Health Serv Outcomes Res Method, 21: 339–362.
- Zandeh SO, Yoon-Flannery K, Kuperman GJ, et al., 2008, Challenges to EHR implementation in electronic versuspaper-based office practices. J Gen Intern Med, 23: 755–761. https://doi.org/10.1007/s11606-008-0573-5
- Tully MP, 2012, Prescribing errors in hospital practice. Br J Clin Pharmacol, 74: 668–675. https://doi.org/10.1111/j.1365-2125.2012.04313.x
- Franklin BD, Birch S, Savage I, et al., 2009, Methodological variability in detecting prescribing errors and consequences for the evaluation of interventions. Pharmacoepidemiol Drug Saf, 18: 999. https://doi.org/10.1002/pds.1811
- Franklin BD, Reynolds M, Shebl NA, et al., 2011, Prescribing errors in hospital inpatients: A three-centre study of their prevalence, types and cause. Postgrad Med J, 87: 739–745.
- Otero P, Leyton A, Mariani G, et al., 2008, Medication errors in pediatric inpatients: Prevalence and results of a prevention program. Patient Safety Committee, 122: e737–e743. https://doi.org/10.1542/peds.2008-0014
- Ghaleb MA, Barber N, Franklin BD, Wong IC, 2010, The incidence and nature of prescribing and medication administration errors in paediatric inpatients. Arch Dis Child, 95: 113–118. https://doi.org/10.1136/adc.2009.158485
- Akici A, Kalaça S, Uğurlu MU, et al., 2004, Patient knowledge about drugs prescribed at primary healthcare facilities. Pharmacoepidemiology Drug Saf, 13: 871–876.
- WHO, 2018, Global status Report on Road Safety. Geneva: WHO.
- Tashkandy MA, Gazzaz ZJ, Farooq MU, et al., 2008, Reasons for delay in inpatient admission at an emergency department. J Ayub Med Coll Abottabad, 20: 38–42.
- Bukhari H, Albazli K, Almaslmani S, et al., 2014, Analysis of waiting time in emergency department of Al-Noor specialist hospital, Makkah, Saudi Arabia. J Emerg Med, 2: 67–73. https://doi.org/10.24941/ijcr.41372.05.2021
- Baker C, Melby V, 1996, An investigation into the attitudes and practices of intensive care nurses towards verbal communication with unconscious patients. J Clin Nurs, 5: 185–192. https://doi.org/10.1111/j.1365-2702.1996.tb00248.x
- Roman LC, Ancker JS, Johnson SB, et al., 2017, Navigation in the electronic health record: A review of the safety and usability literature. J Biomed Inf, 67: 69–79. https://doi.org/10.1016/j.jbi.2017.01.005
- Slawomirski L, Lindner L, De Bienassis K, et al., 2021, Progress on implementing and using Electronic Health Record Systems: Developments in OECD Countries as of 2021. France: OECD. https://doi.org/10.1787/18152015
- Wulff A, Mast M, Hassler M, et al., 2020, Designing an openEHR-based pipeline for extracting and standardizing unstructured clinical data using Natural language processing. Methods Inf Med, 59: e64–e78.
- Evans RS, 2016, Electronic Health Records: Then, Now, and in the Future. Yearb Med Inform, 1: s48–s61.
- Zhang J, Walji MF, 2011, TURF: Toward a unified framework of EHR usability. J Biomed Inform, 44: 1056–1067. https://doi.org/10.1016/j.jbi.2011.08.005
- Tranfield D, Denyer D, Smart P, 2003, Towards a methodology for developing evidence-informed management knowledge by means of systematic review. Br J Manag, 14: 207–222.
- Tissot H, Dobson R, 2019, Combining string and phonetic similarity matching to identify misspelt names of drugs in medical records written in Portuguese. J Biomed Semantics, 10: 17.
- Feller DJ, Zucker J, Yin MT, 2018, Using clinical notes and natural language processing for automated HIV risk assessment. J Acquir Immune Defic Syndr, 77: 160–166. https://doi.org/10.1016/j.ijmedinf.2017.12.024
- Workman TE, Shao Y, Divita G, 2019, An efficient prototype method to identify and correct misspellings in clinical text. BMC Res Notes, 12: 42. https://doi.org/10.1186/s13104-019-4073-y
- Hanauer DA, Barnholtz-Sloan JS, Beno MF, et al., 2020, Electronic medical record search engine (EMERSE): An information retrieval tool for supporting cancer research. JCO Clin Cancer Inform, 4: 454–463. https://doi.org/10.1200/CCI.19.00134
- Baxter SL, Klie AR, Radha Saseendrakumar B, et al, 2020, Text processing for detection of fungal ocular involvement in critical care patients: Cross-sectional study. J Med Internet Res., 22: e18855. https://doi.org/10.2196/18855
- Tao C, Filannino M, Uzuner O, 2017, Prescription extraction using CRFs and word embeddings. J Biomed Inform, 72: 60–66. https://doi.org/10.1016/j.jbi.2017.07.002
- Liu Z, Yang M, Wang X, et al., 2017, Entity recognition from clinical texts via recurrent neural network. BMC Med Inform Decis Mak, 17: 67. https://doi.org/10.1186/s12911-017-0468-7
- Cai X, Dong S, Hu J, 2019, A deep learning model incorporating part of speech and self-matching attention for named entity recognition of Chinese electronic medical records. BMC Med Inform Decis Mak, 19: 65. https://doi.org/10.1186/s12911-019-0762-7
- Thirukumaran CP, Zaman A, Rubery PT, et al., 2019, Natural language processing for the identification of surgical site infections in orthopaedics. J Bone Joint Surg Am, 101: 2167–2174. https://doi.org/10.2106/JBJS.19.00661
- Dipaola F, Gatti M, Pacetti V, et al., 2019, Artificial intelligence algorithms and natural language processing for the recognition of syncope patients on emergency department medical records. J Clin Med, 8: 1677. https://doi.org/10.3390/jcm8101677
- Maarseveen TD, Meinderink T, Reinders MJ, et al., 2020, Machine learning electronic health record identification of patients with rheumatoid arthritis: Algorithm pipeline development and validation study. JMIR Med Inform, 8: e23930. https://doi.org/10.2196/23930
- Gregg JR, Lang M, Wang LL, et al., 2017, Automating the determination of prostate cancer risk strata from electronic medical records. JCO Clin Cancer Inform, 1: CCI.16.00045. https://doi.org/10.1200/CCI.16.00045
- Nath C, Albaghdadi MS, Jonnalagadda SR, 2016, A natural language processing tool for large-scale data extraction from echocardiography reports. PLoS One, 11: e0153749. https://doi.org/10.1371/journal.pone.0153749
- Goldstein A, Shahar Y, 2018, An automated knowledge-based textual summarization system for longitudinal, multivariate clinical data. J Biomed Inform, 61: 159–175. https://doi.org/10.1016/j.jbi.2016.03.022
- He B, Dong B, Guan Y, 2017, Building a comprehensive syntactic and semantic corpus of Chinese clinical texts. J Biomed Inform, 69: 203–217. https://doi.org/10.1016/j.jbi.2017.04.006
- Becker M, Kasper S, Böckmann B, et al., 2019, Natural Language Processing of German clinical colorectal cancer notes for guideline-based treatment evaluation. Int J Med Inform, 127: 141–146. https://doi.org/10.1016/j.ijmedinf.2019.04.022
- Afzal N, Sohn S, Abram S, et al., 2016, Identifying peripheral arterial disease cases using natural language processing of clinical notes. IEEE EMBS Int Conf Biomed Health Inform, 2016: 126–131. https://doi.org/10.1109/BHI.2016.7455851
- Afzal N, Sohn S, Abram S, et al., 2017, Mining peripheral arterial disease cases from narrative clinical notes using natural language processing. J Vasc Surg, 65: 1753–1761. https://doi.org/10.1016/j.jvs.2016.11.031
- Hassanpour S, Bay G, Langlotz CP, 2017, Characterization of change and significance for clinical findings in radiology reports through natural language processing. J Digit Imaging, 30: 314–322. https://doi.org/10.1007/s10278-016-9931-8
- Chase HS, Mitrani LR, Lu GG, et al., 2017, Early recognition of multiple sclerosis using natural language processing of the electronic health record. BMC Med Inform Decision Making, 17: 24. https://doi.org/10.1186/s12911-017-0418-4
- Zeng Z, Espino S, Roy A, et al., 2018, Using natural language processing and machine learning to identify breast cancer local recurrence. BMC Bioinformatics, 19: 498. https://doi.org/10.1186/s12859-018-2466-x
- Jones BE, South BR, Shao Y, et al., 2018, Development and validation of a natural language processing tool to identify patients treated for pneumonia across VA emergency department. Appl Clin Inform, 9: 122–128. https://doi.org/10.1055/s-0038-1626725
- Goff DJ, Loehfelm TW, 2018, Automated radiology report summarization using an open-source natural language processing pipeline. J Digit Imaging, 31: 185–192. https://doi.org/10.1007/s10278-017-0030-2
- Bai T, Chanda AK, Egleston BL, et al., 2018, EHR phenotyping via jointly embedding medical concepts and words into a unified vector space. BMC Med Inform Decision Mak, 18: 123. https://doi.org/10.1186/s12911-018-0672-0
- Chapman AB, Mowery DL, Swords DS, et al., 2018, Detecting evidence of Intra-abdominal surgical site infections from radiology reports using natural language processing. AMIA Annu Symp Proc., 2017: 515–524.
- Zhang Y, Li HJ, Wang J, et al., 2018, Adapting word embeddings from multiple domains to symptom recognition from psychiatric notes. AMIA Jt Summits Trnsl Sci Proc, 2018: 281–289.
- Afshar M, Joyce C, Oakey A, et al., 2018, A computable phenotype for acute respiratory distress syndrome using natural language processing and machine learning. AMIA Annu Symp Proc, 2018: 157–165.
- Percha B, Zhang Y, Bozkurt S, et al., 2018, Expanding a radiology lexicon using contextual patterns in radiology reports. J Am Med Inform Assoc, 25: 679–685. https://doi.org/10.1093/jamia/ocx152
- Koza W, Filippo D, Cotik V, et al., 2019, Automatic detection of negated findings in radiological reports for Spanish language: Methodology based on lexicon-grammatical information processing. J Digit Imaging, 32: 19–29. https://doi.org/10.1007/s10278-018-0113-8
- Lee JK, Jensen CD, Levin TR, et al., 2019, Accurate identification of colonoscopy quality and polyp findings using natural language processing. J Clin Gastroenterol, 53: e25–e30. https://doi.org/10.1097/MCG.0000000000000929
- Shen F, Larson DW, Naessens JM, et al., 2019, Detection of surgical site infection utilizing automated feature generation in clinical notes. J Healthc Inform Res., 3: 267–282. https://doi.org/10.1007/s41666-018-0042-9
- Topaz M, Adams V, Wilson P, et al., 2020, Free-text documentation of dementia symptoms in home healthcare: A natural language processing study. Gerontol Geriatr Med, 6: 1–10. https://doi.org/10.1177/2333721420959861
- Shi J, Liu S, Pruitt LC, et al., 2019, Using natural language processing to improve EHR structured data-based surgical site infection surveillance. AMIA Annu Symp Proc., 2019: 794–803.
- Senders JT, Karhade AV, Cote DJ, et al., 2019, Natural language processing for automated quantification of brain metastases reported in free-text radiology reports. Clin Cancer Inform, 3: 1–9. https://doi.org/10.1200/CCI.18.00138
- Misra-Hebert AD, Milinovich A, Zajichek A, et al., 2020, Natural language processing improves detection of nonsevere hypoglycemia in medical records versus coding alone in patients with type 2 diabetes but does not improve prediction of severe hypoglycemia events: An analysis using the electronic medical record in a large health system. Diabetes Care, 43: 1937–1940. https://doi.org/10.2337/dc19-1791
- König M, Sander A, Demuth I, et al., 2019, Knowledge-based best of breed approach for automated detection of clinical events based on German free text digital hospital discharge letters. PLoS One, 14: e0224916. https://doi.org/10.1371/journal.pone.0224916
- Oliveira CR, Niccolai P, Ortiz AM, et al., 2020, Natural language processing for surveillance of cervical and anal cancer and precancer: Algorithm development and Split-validation study. JMIR Med Inform, 8: e20826.
- Wang Y, Ananiadou S, Tsujii J, 2019, Improving clinical named entity recognition in Chinese using the graphical and phonetic feature. BMC Med Inform Decis Mak, 19: 273. https://doi.org/10.1186/s12911-019-0980-z
- Tou, H, Yao, L., Wei, Z, et al., 2018, Automatic infection detection based on electronic medical records. BMC Bioinformatics, 19: 117. https://doi.org/10.1186/s12859-018-2101-x
- Bozkurt S, Alkim E, Banerjee I, et al., 2019, Automated detection of measurements and their descriptors in radiology reports using a hybrid natural language processing algorithm. Journal of Digit Imaging, 32: 544–553. https://doi.org/10.1007/s10278-019-00237-9
- Doan S, Maehara CK, Chaparro JD, et al., 2016, Building a natural language processing tool to identify patients with high clinical suspicion for kawasaki disease from emergency department notes. Acad Emerg Med., 23: 628–636. https://doi.org/10.1111/acem.12925
- Li X, Wang, H, He H, et al., 2019, Intelligent diagnosis with Chinese electronic medical records based on convolutional neural networks. BMC Bioinformatics, 20: 62. https://doi.org/10.1186/s12859-019-2617-8
- Cai T, Zhang L, Yang N, et al., 2019, EXTraction of EMR numerical data: An efficient and generalizable tool to EXTEND clinical research. BMC Med Inf Decis Mak, 19: 226. https://doi.org/10.1186/s12911-019-0970-1
- Liu Y, Liu Q, Han C, et al., 2019, The implementation of Natural Language Processing to extract index lesions from breast magnetic resonance imaging reports. BMC Med Inform Decis Mak, 19: 288. https://doi.org/10.1186/s12911-019-0997-3
- Si Y, Roberts K, 2018, A frame-based NLP system for cancer-related information extraction. AMIA Annu Symp Proc, 2018: 1524–1533.
- Li R, Hu B, Liu F, 2019, Detection of bleeding events in electronic health record notes using convolutional neural network models enhanced with recurrent neural network autoencoders: Deep learning approach. JMIR Med Inform, 8: e10788. https://doi.org/10.2196/10788
- Afzal N, Sohn S, Scott CG, 2017, Surveillance of peripheral arterial disease cases using natural language processing of clinical notes. AMIA Jt Summits Transl Sci Proc, 2017: 28–36.
- Kim Y, Garvin JH, Goldstein MK, 2017, Extraction of left ventricular ejection fraction information from various types of clinical reports. J Biomed Inform, 67: 42–48. https://doi.org/10.1016/j.jbi.2017.01.017
- McCoy TH Jr., Yu S, Hart KL, et al., 2018, High throughput phenotyping for dimensional psychopathology in electronic health records. Biol Psychatry, 83: 997–1004. https://doi.org/10.1016/j.biopsych.2018.01.011
- Viani N, Miller TA, Napolitano C, et al., 2019, Supervised methods to extract clinical events from cardiology reports in Italian. J Biomed Inform, 95: 103219. https://doi.org/10.1016/j.jbi.2019.103219
- Kang SK, Garry K, Chung R, 2019, Natural language processing for identification of incidental pulmonary nodules in radiology reports. J Am Coll Radiol, 16: 1587–1594. https://doi.org/10.1016/j.jacr.2019.04.026
- Chen T, Dredze M, Weiner JP, et al., 2019, Extraction of geriatric syndromes from electronic health record clinical notes: Assessment of statistical natural language processing methods. JMIR Med Inform, 7: e13039. https://doi.org/10.2196/13039
- Hardjojo A, Gunachandran A, Pang L, et al., 2018, Validation of a natural language processing algorithm for detecting infectious disease symptoms in primary care electronic medical records in Singapore. JMIR Med Inform, 6: e36. https://doi.org/10.2196/jmir.8371
- Guan M, Cho S, Petro R, et al., 2019, Natural language processing and recurrent network models for identifying genomic mutation-associated cancer treatment change from patient progress notes. JAMIA Open, 2: 139–149. https://doi.org/10.1093/jamiaopen/ooy061
- Moon S, Carlson LA, Moser ED, et al., 2022, Identifying information gaps in electronic health records by using natural language processing: gynecologic surgery history identification. J Med Internet Res, 24: e29015. https://doi.org/10.2196/29015