Computer vision profiling and identification of authentic Jackson Pollock drip paintings
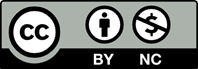
The drip paintings of Jackson Pollock introduced a unique style of abstract expressionism that has attracted substantial interest. Here, a quantitative approach to the analysis of Jackson Pollock’s drip paintings was applied. The analysis was performed using quantitative elements from authentic Jackson Pollock paintings, and comparing them to the same quantitative elements computed from fake Jackson Pollock paintings. Profiling the differences can lead to the identification of unique mathematical elements that characterize Jackson Pollocks artistic style. These elements provide a new way of examining and analyzing the visual content of art. That quantitative analysis can expand on the qualitative analysis methods and provide new insights about visual art. The results show that authentic Jackson Pollock paintings can be differentiated from faked Jackson Pollock paintings in ∼96% of the cases. While fractals provide the strongest differences between authentic and faked Pollock paintings, the analysis also reveals other elements of the paintings that are unique to Jackson Pollock’s style. These elements include textures, entropy, and the distribution of light.
- Abraham RG, Van Den Bergh S, Nair P. A new approach to galaxy morphology. I. Analysis of the Sloan digital sky survey early data release. Astrophys J. 2003;588(1):218-229. doi: 10.1086/373919
- Aviv V. What does the brain tell us about abstract art? Front Hum Neurosci. 2014;8:85. doi: 10.3389/fnhum.2014.00085
- Bavi A, Shamir L, Xi TM, Ajand Y. Computer Algorithm Classified Artwork for Generative Design in 20th Generative Art Conference, at Milano, Italy; 2017.
- Bianco S, Mazzini D, Schettini R. Deep Multibranch Neural Network for Painting Categorization. In: International Conference on Image Analysis and Processing. Netherlands: Springer; 2017. p. 414-423.
- Bishop CM. Pattern Recognition and Machine Learning. Netherlands: Springer; 2006.
- Burcoff A, Shamir L. Computer analysis of Pablo Picasso’s artistic style. Int J Art Cult Des Technol. 2017;6(1):1-18. doi: 10.4018/IJACDT.2017010101
- Coddington J, Elton J, Rockmore D, Wang Y. Multifractal Analysis and Authentication of Jackson Pollock Paintings. In: Computer Image Analysis in the Study of Art. Vol. 6810, International Society for Optics and Photonics; 2008. p. 68100F. doi: 10.1117/12.765015
- Cover T, Hart P. Nearest neighbor pattern classification. IEEE Trans Inform Theory. 1967;13:21-27. doi: 10.1109/TIT.1967.1053964
- Criminisi A, Kemp M, Zisserman A. Bringing pictorial space to life: Computer techniques for the analysis of paintings. In: Digital Art History: A Subject in Transition. Vol. 1. United Kingdom: Intellect; 2005. p. 5.
- Hadjidemetriou E, Grossberg MD, Nayar SK. Spatial Information in Multiresolution Histograms. In: Proceedings of the 2001 IEEE Computer Society Conference on Computer Vision and Pattern Recognition. Vol. 1. New Jersey: IEEE; 2001. p. 1-709. doi: 10.1109/CVPR.2001.990544
- Haralick RM, Shanmugam K, Dinstein IH. Textural features for image classification. IEEE Trans Syst Man Cybernet. 1973;3(6):610-621. doi: 10.1109/TSMC.1973.4309314
- Hunter S, Pollock J, Karpel B. Jackson Pollock. Bull Museum Modern Art. 1956;24(2):3-36.
- Irfan M, Stork DG. Multiple Visual Features for the Computer Authentication of Jackson Pollock’s Drip Paintings: Beyond Box Counting and Fractals. In: ‘Image Processing: Machine Vision Applications II. Vol. 7251, International Society for Optics and Photonics. 2009. p. 72510Q.
- Khan FS, Beigpour S, Van de Weijer J, Felsberg M. Painting-91: A large scale database for computational painting categorization. Mach Vision Appl. 2014;25(6):1385- 1397. doi: 10.1007/s00138-014-0621-6
- Khandekar N, Mancusi-Ungaro C, Cooper H, et al. A technical analysis of three paintings attributed to Jackson Pollock. Stud Conserv. 2010;55(3):204-215. doi: 10.1179/sic.2010.55.3.204
- Lim JS. Two-dimensional Signal and Image Processing. New Haven: Prentice Hall; 1990.
- Orlov N, Shamir L, Macura T, Johnston J, Eckley DM, Goldberg IG. ‘Wnd-charm: Multi-purpose image classification using compound image transforms. Pattern Recognit Lett. 2008;29(11):1684-1693. doi: 10.1016/j.patrec.2008.04.013
- Ratcliff C. The Fate of a Gesture: Jackson Pollock and Post-war American Art. United Kingdom: Routledge; 2019.
- Ryzhik IM, Gradshten IS. Table of Integrals, Series, and Products. New York: Academic Press; 1965.
- Sartori A, Culibrk D, Yan Y, Sebe N. Who’s Afraid of Itten: Using the Art Theory of Color Combination to Analyze Emotions in Abstract Paintings. In: ‘Proceedings of the 23rd ACM International Conference on Multimedia; 2015. p. 311-320. doi: 10.1145/2733373.2806250
- Shamir L. Human Perception-based Color Segmentation Using Fuzzy Logic. In: International Conference on Image Processing, Computer Vision and Pattern Recognition. Vol. 2. 2006. p. 96-502.
- Shamir L. Computer analysis reveals similarities between the artistic styles of van Gogh and Pollock. Leonardo. 2012;45(2):149-154. doi: 10.1162/LEON_a_00281
- Shamir L. What makes a Pollock Pollock: A machine vision approach. Int J Arts Technol. 2015;8(1):1-10. doi: 10.1504/IJART.2015.067389
- Shamir L. Udat: A Multi-purpose Data Analysis Tool. Astrophysics Source Code Library ascl:1704.002; 2017.
- Shamir L. UDAT: Compound quantitative analysis of text using machine learning. Digit Scholarsh Humanit. 2021;36(1):187-208. doi: 10.1093/llc/fqaa007
- Shamir L, Tarakhovsky JA. Computer analysis of art. J Comput Cult Herit. 2012;5(2):1-11. doi: 10.1145/2307723.2307726
- Shamir L, Macura T, Orlov N, Eckley DM, Goldberg IG. Impressionism, expressionism, surrealism: Automated recognition of painters and schools of art. ACM Trans Appl Percept. 2010;7(2):1-17. doi: 10.1145/1670671.1670672
- Shamir L, Nissel J, Winner E. Distinguishing between abstract art by artists vs. children and animals: Comparison between human and machine perception. ACM Trans Appl Percept. 2016;13(3):1-17. doi: 10.1145/2912125
- Shamir L, Orlov N, Goldberg IG. Evaluation of the Informativeness of Multi-order Image Transforms. In: International Conference on Image Processing, Computer Vision and Pattern Recognition; 2009. p. 37-42.
- Shamir L, Orlov N, Eckley DM, Macura T, Johnston J, Goldberg IG. Wndchrm-an open source utility for biological image analysis. Source Code Biol Med. 2008;3(1):13. doi: 10.1186/1751-0473-3-13
- Solomon D. Jackson Pollock: A Biography. Maryland: Rowman and Littlefield; 2001.
- Solso RL. The cognitive neuroscience of art: A preliminary fMRI observation. J Conscious Stud. 2000;7(8-9):75-86.
- Solso RL. Brain activities in a skilled versus a novice artist: An fMRI study. Leonardo. 2001;34(1):31-34. doi: 10.1162/002409401300052479
- Stork DG. Computer Vision and Computer Graphics Analysis of Paintings and Drawings: An Introduction to the Literature. In: International Conference on Computer Analysis of Images and Patterns. Netherlands: Springer; 2009. p. 9-24. doi: 10.1007/978-3-642-03767-2_2
- Tamura H, Mori S, Yamawaki T. Textural features corresponding to visual perception. IEEE Trans Syst Man Cybernet. 1978;8(6):460-473.
- Tan WR, Chan CS, Aguirre HE, Tanaka K. Ceci N’est Pas Une Pipe: A Deep Convolutional Network for Fine-art Paintings Classification. In: IEEE International Conference on Image Processing. New Jersey: IEEE; 2016. p. 3703-3707.
- Taylor RP, Guzman R, Martin T, et al. Authenticating Pollock paintings using fractal geometry. Pattern Recogn Lett, 2007;28(6):695-702. doi: 10.1016/j.patrec.2006.08.012
- Taylor RP, Micolich AP, Jonas D. Fractal analysis of Pollock’s drip paintings. Nature, 1999;399(6735):422.
- Teague MR. Image analysis via the general theory of moments. J Opt Soc Am. 1980;70(8):920-930. doi: 10.1364/JOSA.70.000920
- Wu CM, Chen YC, Hsieh KS. Texture features for classification of ultrasonic liver images. IEEE Trans Med Imaging. 1992;11(2):141-152. doi: 10.1109/42.141636