Robust reformulations of ambiguous chance constraints with discrete probability distributions
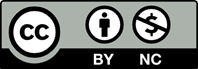
[1] Charnes, A. & Cooper, W.W. (1959). Chance- constrained programming. Management Sci- ence, 6(1), 73–79.
[2] Charnes, A., Cooper, W.W. & Symonds, G.H.(1958). Cost horizons and certainty equiva- lents: an approach to stochastic programming of heating oil. Management Science, 4(3), 235–263.
[3] Miller, B.L. & Wagner, H.M. (1965). Chance constrained programming with joint con- straints. Operations Research, 13(6), 930–945.
[4] Pr,ekopa, A. (1970). E伍cient robust opti- mization of metal forming processes using a sequential metamodel based strategy. In Proceedings of the Princeton Symposium on Mathematical Programming, Princeton Uni- versity Press, Princeton, NJ, 113–138.
[5] Burkauskas, A. (1986). On the convexity problem of probabilistic constraint stochastic programming problems. Alkalmazott Matem- atikai Lapok, 12, 77–90.
[6] Pr,ekopa, A. (1974). Programming under probabilistic constraints with a random tech- nology matrix. Mathematische Operations- forschung und Statistik, 5, 109–116.
[7] Van de Panne, C. & Popp, W., (1963). Minimum-cost cattle feed under probabilis- tic protein constraints. Management Science, 9(3), 405–430.
[8] Pr,ekopa, A. (1973). On logarithmic concave measures and functions. Acta Scientiarum Mathematicarum, 34, 335–343.
[9] Pr,ekopa, A. (1995). Stochastic Programming Kluwer Academic Publishers, Dordrecht, The Netherlands.
[10] Nemirovski, A. & Shapiro, A. (2006). Convex approximations of chance constrained pro- grams. SIAM Journal on Optimization, 17(4), 969–996.
[11] Ben-Tal, A. & Nemirovski, A. (2000). Robust solutions of linear programming problems contaminated with uncertain data. Mathe- matical Programming, 88(3), 411–424.
[12] Chen, W., Sim, M., Sun, J. & Teo, C.P.(2010). From CVaR to uncertainty set: impli- cations in joint chance-constrained optimiza- tion. Operations Research, 58(2), 470–485.
[13] Chen, X., Sim, M. & Sun, P. (2007). A robust optimization perspective on stochastic pro- gramming. Operations Research 55(6), 1058– 1107.
[14] Zymler S., Kuhn, D. & R¨ustem, B. (2013). Distributionally robust joint chance con- straints with second-order moment informa- tion. Mathematical Programming, 137(1-2), 167–198.
[15] Calafiore, G.C. & Campi, M.C. (2005). Un- certain convex programs: randomized so- lutions and confidence levels. Mathematical Programming, 102, 25–46.
[16] Campi, M.C. & Garatti, S. (2008). The ex- act feasibility of randomized solutions of ro- bust convex programs. SIAM Journal on Op- timization, 19(3), 1211–1230.
[17] Birge, J.R. & Wets, R.J.-B. (1986). Design- ing approximation schemes for stochastic op- timization problems, in particular for sto- chastic programs with recourse. Mathematical Programming Studies, 27, 54–102.
[18] DupaYcov,a, J. (2001). Stochastic Program- ming: minimax approach. In: Encyclopedia of Optimization. Kluwer Academic Publish- ers, Dordrecht, The Netherlands.
[19] Shapiro, A. & Ahmed, S. (2004). On a class of minimax stochastic programs. SIAM Jour- nal on Optimization, 14(4), 1237–1252.
[20] Shapiro, A. & Kleywegt, A.J. (2002). Min- imax analysis of stochastic problems. Opti- mization Methods and Software, 17, 523–542.
[21] Epstein, L.G. & Schneider, M. (2003). Re- cursive multiple-priors. Journal of Economic Theory, 113(1), 1–31.
[22] Epstein, L.G. & Schneider, M. (2007). Learn- ing under ambiguity. Review of Economic Studies, 74(4), 1275–1303.
[23] Hansen, L.P. & Sargent, T.J. (2001). Ro- bust control and model uncertainty. American Economic Review, 91, 60–66.
[24] Erdo an, E. & Iyengar, G. (2006). Am- biguous chance constrained problems and robust optimization. Mathematical Program- ming, 107(1–2), 37–61.
[25] Yanlko lu, I(˙), den Hertog, D. (2013). Safe ap-proximations of ambiguous chance constraints using historical data. INFORMS Journal on Computing, 25(4), 666–681.
[26] Ben-Tal, A., El Ghaoui, L., Nemirovski, A.(2009). Robust Optimization. Princeton Press, Princeton, NJ.
[27] Nemirovski, A. (2012). On safe tractable ap- proximations of chance constraints. European Journal of Operations Research, 219(3), 707– 718
[28] Yanlko lu, I(˙) & den Hertog, D. & Kleij-nen, J.P.C. (2016). Robust dual-response op- timization. IIE Transactions, 48(3), 298–312.
[29] Luedtke, J. (2014). A branch-and-cut de- composition algorithm for solving chance- constrained mathematical programs with fi- nite support. Mathematical Programming 146(1–2), 219-244.
[30] Song, Y., Luedtke, J. R., & K¨u>c¨ukyavuz, S. (2014). Chance-constrained binary packing problems. INFORMS Journal on Computing, 26(4), 735-747.
[31] Hanasusanto, G.A., Roitch, V., Kuhn, D. & Wiesemann, W. (2015). A distributionally robust perspective on uncertainty quantifi- cation and chance constrained programming. Mathematical Programming, 151(1), 35–62.
[32] Hanasusanto, G.A., Roitch, V., Kuhn, D. & Wiesemann, W. (2017). Ambiguous joint chance constraints under mean and dispersion information. Operations Research, 65(3), 751– 767.
[33] Jiang, R. & Guan, Y. (2016). Data-driven chance constrained stochastic program. Math- ematical Programming, 158(1-2), 291–327.
[34] Chen, Z., Kuhn, D., & Wiesemann, W.(2018). Data-driven chance constrained pro- grams over Wasserstein Balls. arXiv preprint, arXiv:1809.00210.
[35] Ji, R. & Lejeune, M. (2018). Data-driven dis- tributionally robust chance-constrained pro- gramming with Wasserstein metric. Available at Optimization Online.
[36] Zhang, Y., Jiang, R. & Shen, S. (2016). Dis- tributionally robust chance-constrained bin packing. Available at Optimization Online.
[37] Cheng, J., Delage, E. & Lisser, A. (2014). Distributionally robust stochastic knapsack problem. SIAM Journal on Optimization, 24(3), 1485–1506.
[38] Hu, Z. & Hong, L.J. (2013). Kullback-Leibler divergence constrained distributionally ro- bust optimization. Available at Optimization Online.
[39] Xie, W. & Ahmed, S. (2018). On determin- istic reformulations of distributionally robust joint chance constrained optimization prob- lems. SIAM Journal on Optimization, 28(2), 1151–1182.
[40] Xie, W., Ahmed, S. & Jiang, R. (2017). Optimized Bonferroni approximations of dis- tributionally robust joint chance constraints. Available at Optimization Online.
[41] Xie, W. (2018). On distributionally robust chance constrained program with wasserstein distance. arXiv preprint, arXiv:1806.07418.
[42] Mehrotra, S. & Zhang, H. (2014). Mod- els and algorithms for distributionally robust least squares problems. Mathematical Pro- gramming, 146(1-2), 123–141.
[43] O(¨)zmen, A., Weber, G.W., Batmaz, I(˙) . &Kropat, E. (2011). RCMARS: Robustifica- tion of CMARS with diferent scenarios under polyhedral uncertainty set. Communications in Nonlinear Science and Numerical Simula- tion, 16(12), 4780–4787.
[44] Friedman, J.H. (1991). Multivariate adaptive regression splines. Annals of Statistics, 19(1), 1–141.
[45] Weber, G.W., Batmaz, I(˙)., K¨oksal, G.,Taylan, P. & F. Yerlikaya-O(¨)zkurt, (2012).CMARS: a new contribution to nonparamet- ric regression with multivariate adaptive re- gression splines supported by continuous op- timization. Inverse Problems in Science and Engineering, 20(3), 371–400.
[46] Bemis, C., Hu, X., Lin, W., Moazeni, S., Wang, L., Wang, T. & Zhang, J. (2009). Ro- bust portfolio optimization using a simple fac- tor model. IMA Preprint Series, No. 2284, University of Minnesota, Minneapolis, MN.
[47] Kara, G., O(¨)zmen, A., & Weber, G. W.(2019). Stability advances in robust portfo- lio optimization under parallelepiped uncer- tainty. Central European Journal of Opera- tions Research, 27(1), 241–261.
[48] Postek, K., den Hertog, D. & Melenberg, B. (2016). Computationally tractable coun- terparts of distributionally robust constraints on risk measures. SIAM Review, 58(4), 603- –650.
[49] Gorissen, B.L., Yanlkoglu, I(˙) & den Hertog,D. (2015). A practical guide to robust opti- mization. Omega, 53, 124–137.
[50] Ben-Tal, A., den Hertog, D. & and Vial, J.-P. (2015). Deriving robust counterparts of nonlinear uncertain inequalities. Mathemati- cal Programming, 149(1-2), 265–299.
[51] Yanlkoglu, I(˙) . (2018). Selected Topics in Ro-bust Optimization. In: Optimization Tech- niques for Problem Solving in Uncertainty. IGI Global, PA, USA.