Copula approach to select input/output variables for DEA
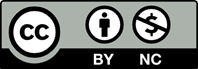
Determination of the input/output variables is an important issue in Data Envelopment Analysis (DEA). Researchers often refer to expert opinions in defining these variables. The purpose of this paper is to propose a new approach to determine the input/output variables, it is important to keep in mind that especially when there is no any priori information about variable selection. This new proposed technique is based on a theoretical method which is called “Copula”. Copula functions are used for modeling the dependency structure of the variables with each other. Also we use the local dependence function which analyzes the point dependency of variables of copulas to define the input/output variables. To illustrate the usefulness of the proposed approach, we conduct two applications using simulated and real data and compare the efficiencies in DEA. Our results show that new approach gives values close to perfection.
[1] Charnes A., Cooper W.W. and Rhodes E., Measuring the Efficiency of Decision Making Units. European Journal of Operational Research, 2(6), 429-444 (1978).
[2] Yoluk, M., Hastane Performasının Veri Zarflama Analizi (VZA) Yöntemi İle Değerlendirilmesi. Thesis (MSc). Atılım University (2010).
[3] Morita, H. and Avkiran, N.K., Selecting Inputs and Outputs in Data Envelopment Analysis by Designing Statistical Experiments. Journal of Operational Research Society of Japan, 52, 163-173 (2009).
[4] Nelsen, R.B., An Introduction to Copulas. Springer, New York (1999).
[5] Bairamov, I. and Kotz, S., On Local Dependence Function for Multivariate Distributions. New Trends in Prob. and Stat., 5, 27-44 (2000).
[6] Bairamov, I., Kotz, S. and Kozubowski, T.J., A New Measure of Linear Local Dependence. Statistics, 37(3), 243-258 (2003).
[7] Ramanathan, R., An Introduction to Data Envelopment Analysis. Sage Publications, New Delhi (2003).
[8] Luo, Y., Bi, G. and Liang, L., Input/Output Indicator Selection for DEA Efficiency Evaluation: An Empirical Study of Chinese Commercial Banks. Expert System with Applications, 39, 1118- 1123 (2012).
[9] Pastor, J.T., Ruiz J.L. and Sirvent, I., A Statistical Test for Nested Radial Dea Models. INFORMS, 50(4), 728-735 (2002)
[10] Jenkins, L. and Anderson, M., A multivariate statistical approach to reducing the number of variables in data envelopment analysis. European Journal of Operational Research, 147, 51–61 (2003).
[11] Ruggiero, J., Impact Assessment of Input Omission on DEA. International Journal of Information Technology and Decision Making, 4(3), 359-368, (2005).
[12] Edirisinghe, N.C.P. and Zhang, X., Generalized DEA Model of Fundamental Analysis and Its Application to Portfolio Optimization. Journal of Banking and Finance, 31, 311-335, (2007)
[13] Madhanagopal, R. and Chandrasekaran, R., Selecting Appropriate Variables for DEA Using Genetic Algorithm (GA) Search Procedure. International Journal of Data Envelopment Analysis and Operations Research, 1(2), 28-33 (2014).
[14] Banker, R.D., Charnes, A. and Cooper W.W., Some Models for Estimating Technical and Scale Inefficiencies in Data Envelopment Analysis. Management Science, 30(9), 1078-1092 (1984).
[15] Fare, R. and Grosskopf, S., and Lovell, C.A.K., Production Frontiers. Cambridge University Press (1993).
[16] Martic, M.M., Novakovic, M.S. and Baggia, A., Data Envelopment Analysis - Basic Models and their Utilization, Organizacija. Journal of Management, Informatics and Human Resources, 42(2), 37-43 (2009).
[17] Cooper, W.W., Seiford, L.M. and Zhu, J., Handbook on Data Envelopment Analysis. Springer New York (2011).
[18] Peňa, V.H. and Ibragimov, R., Sharakhmetov, S., Characterizations of Joint Distributions, Copula Information, Dependence and Decoupling, with Applications to Time Series. IMS Lecture NotesMonograph Series, 2nd Lehmann SymposiumOptimality, 49, 183-209 (2006).
[19] Bairamov, I. and Kotz, S., Dependence Structure and Symmetry of Huang-Kotz FGM Distributions and Their Extensions. Metrica, 56, 55-72 (2002).
[20] Bairamov, I., Kotz, S. and Bekci, M., New Generalized Farlie-Gumbel-Morgenstern Distributions and Concomitants of Order Statistics. Journal of Applied Statistics, 28(5), 521-536 (2001).
[21] Najjari, V., Yeni Arşimedyan Kapula Aileleri ve Finans Alanında Bir Uygulama. Thesis (PhD). Gazi University (2014).
[22] Vandenhende, F., and Lambert, P., Local dependence estimation using semiparametric Archimedean copulas. The Canadian Journal of Statistics, 33(3), 377-388, (2005).