Fuzzy-PID and interpolation: a novel synergetic approach to process control
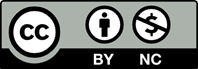
This paper presents a novel approach for tuning a fuzzy-based proportional-integral-derivative (PID) controller to enhance the control performance of a chemical process control system. The proposed approach combines the advantages of fuzzy- PID and interpolation to achieve improved control performance. Properly tuned PID controllers can help maintain process stability, minimize deviations from setpoints, and ensure efficient operation in industrial systems. Fuzzy logic allows for the incorporation of expert knowledge and linguistic rules, enabling the controller to handle uncertain and imprecise process information. Fuzzy PID controllers combine fuzzy logic and conventional PID control to enhance control performance, particularly in systems with complex or nonlinear dynamic such as chemical plant. It dynamically adjusts the PID parameters—proportional gain (Kp), integral gain (Ki), and derivative gain (Kd)—based on error e(t) and change of error Delta e(t). Interpolation plays a crucial role in this context by filling in the gaps or handling situations not explicitly covered by the fuzzy rules. Comparative studies are conducted to evaluate the performance of the fuzzy PID controller against conventional PID controllers and other advanced control techniques. It is demonstrated that the synergy between fuzzy logic and interpolation not only enhances control performance but also offers a more intuitive and adaptable solution for addressing the complexities of modern chemical process control systems.
[1] Ziegler, J. G & Nichols, N.B. (1942). Optimum settings for automatic controllers: The new global context. Transactions of the American society of mechanical engineers, 64(8), 759-765. https: //doi.org/10.1115/1.4019264
[2] Chien, K. L., Hrones, J. A. & Reswick, J. B. (1952). Optimum settings for automatic controllers: On the automatic control of generalized passive systems. Transactions of the American Society of Mechanical Engineers, 74(2), 175-183. https://doi.org/10.1115/1.4015724
[3] Cohen, G.H. & Coon, G. A. (1953). Optimum settings for automatic controllers: On the automatic control of generalized passive systems. Transactions of the American Society of Mechanical Engineers, 75(5), 827-834. https://doi.org/10.1115/1.4015452
[4] A(˚)str¨om, K. J. & H¨agglund, T. (2004). Revisiting the Ziegler-Nichols step response method for PID control. Journal of process control, 14(6), 635-650. https://doi.org/10.1016/j.jprocont.2004. 01.002
[5] Rivera, D. E., Morari, M., & Skogestad, S. (1986). Internal model control: PID controller design. Industrial & Engineering Chemistry Process Design and Development, 25(1), 252-265. https://doi.org/10.1021/i200032a041
[6] Skogestad, S. & Postlethwaite, I. (2005). Multivariable Feedback Control: Analysis and Design. John Wiley & sons.
[7] O’dwyer, A. (2009). Handbook of PI and PID Controller Tuning Rules. World Scientific. https://doi.org/10.1142/9781848162433
[8] Borase, R. P., Maghade, D. K., Sondkar, S. Y. & Pawar, S. N. (2021). A review of PID control, tuning methods and applications. International Journal of Dynamics and Control, 9, 818-827. https://doi.org/10.1007/s40435-020-00665-4
[9] A(˚)str¨om, K. J., & H¨agglund, T. (1984). Automatic tuning of simple regulators with specifications on phase and amplitude margins. Automatica, 20(5), 645-651. https://doi.org/10.1016/0005-109 8(84)90014-1
[10] Berner, J., Soltesz, K., H¨agglund, T., & A(˚)str¨om,K. J. (2018). An experimental comparison of PID autotuners. Control Engineering Practice, 73, 124-133. https://doi.org/10.1016/j.co nengprac.2018.01.006
[11] U¸cak, K., & Arslant¨urk, B. N. (2023). Adaptive MIMO fuzzy PID controller based on peak observer. International Journal of Optimization & Control: Theories & Applications (IJOCTA) , 13(2), 139-150. https://doi.org/10.11121/i jocta.2023.1247
[12] Demirtas, M., & Ahmad, F. (2023). Fractional fuzzy PI controller using particle swarm optimization to improve power factor by boost converter. An International Journal of Optimization and Control: Theories& Applications (IJOCTA), 13(2), 205-213. https: //doi.org/10.11121/ijocta.2023.1260
[13] Tzafestas, S. & Papanikolopoulos, N. P. (1990). Incremental fuzzy expert PID control. IEEE Transactions on Industrial Electronics, 37(5), 365-371. https://doi.org/10.1109/41.103431
[14] Salem, F. A. (2013). New efficient model-based PID design method. European Scientific Journal, 9(15), 181-199.
[15] Mirrashid, N., Alibeiki, E., & Rakhtala, S. M. (2022). Development and control of an upper limb rehabilitation robot via ant colony optimization-PID and fuzzy-PID controllers. International Journal of Engineering, 35(8), 1488-1493. https://doi.org/10.5829/IJE.2022.35.08B.04
[16] Yesil, E., Kumbasar, T., Dodurka, M. & Sakalli, A. (2014). Peak observer based self-tuning of type- 2 fuzzy PID controller. 10th IFIP International Conference on Artificial Intelligence Applications and Innovations (AIAI), Rhodes, Greece, 487- 497. https://doi.org/10.1007/ 978-3-662-44654-6_48