A numerical scheme for the one-dimensional neural field model
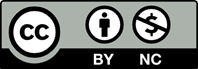
Neural field models, typically cast as continuum integro-differential equations, are widely studied to describe the coarse-grained dynamics of real cortical tissue in mathematical neuroscience. Studying these models with a sigmoidal firing rate function allows a better insight into the stability of localised solutions through the construction of specific integrals over various synaptic connectivities. Because of the convolution structure of these integrals, it is possible to evaluate neural field model using a pseudo-spectral method, where Fourier Transform (FT) followed by an inverse Fourier Transform (IFT) is performed, leading to an identical partial differential equation. In this paper, we revisit a neural field model with a nonlinear sigmoidal firing rate and provide an efficient numerical algorithm to analyse the model regarding finite volume scheme. On the other hand, numerical results are obtained by the algorithm.
[1] Horton, J. C., & Adams, D. L. (2005). The cortical column: a structure without a function. Philosophical Transactions of the Royal Society B: Biological Sciences, 360(1456), 837-862.
[2] DeFelipe, J., Markram, H., & Rockland, K. S. (2012). The neocortical column. Frontiers in Neuroanatomy, 6, 22.
[3] Mountcastle, V. B. (1957). Modality and topographic properties of single neurons of cat’s somatic sensory cortex. Journal of Neurophysiology, 20(4), 408-434.
[4] Martin, R. (2019). Neuroscience Methods: A Guide for Advanced Students. CRC Press.
[5] Beurle, R. L. (1956). Properties of a mass of cells capable of regenerating pulses. Philosophical Transactions of the Royal Society of London. Series B, Biological Sciences, 55-94.
[6] Wilson, H. R., & Cowan, J. D. (1972). Excitatory and inhibitory interactions in localized populations of model neurons. Biophysical Journal, 12(1), 1-24.
[7] Wilson, H. R., & Cowan, J. D. (1973). A mathematical theory of the functional dynamics of cortical and thalamic nervous tissue. Kybernetik, 13(2), 55-80.
[8] Amari, S. I. (1975). Homogeneous nets of neuron-like elements. Biological Cybernetics, 17(4), 211-220.
[9] Amari, S. I. (1977). Dynamics of pattern formation in lateral-inhibition type neural fields. Biological Cybernetics, 27(2), 77-87.
[10] Nunez, P. L. (1974). The brain wave equation: a model for the EEG. Mathematical Biosciences, 21(3-4), 279-297.
[11] Coombes, S. (2010). Large-scale neural dynamics: simple and complex. NeuroImage, 52(3), 731-739.
[12] Ermentrout, G. B., & Cowan, J. D. (1979). A mathematical theory of visual hallucination patterns. Biological Cybernetics, 34(3), 137- 150.
[13] Giese, M. A. (2012). Dynamic neural field theory for motion perception (Vol. 469). Springer Science & Business Media.
[14] Laing, C. R. (2014). PDE Methods for TwoDimensional Neural Fields. In Neural Fields (pp. 153-173). Springer, Berlin, Heidelberg
[15] Coombes, S., beim Graben, P., Potthast, R., & Wright, J. (Eds.). (2014). Neural Fields: Theory and Applications. Springer.
[16] Ermentrout, G. B., & McLeod, J. B. (1993). Existence and uniqueness of travelling waves for a neural network. Proceedings of the Royal Society of Edinburgh Section A: Mathematics, 123(3), 461-478.
[17] Coombes, S. (2005). Waves, bumps, and patterns in neural field theories. Biological Cybernetics, 93(2), 91-108.
[18] Coombes, S., Schmidt, H., & Bojak, I. (2012). Interface dynamics in planar neural field models. Journal of Mathematical Neuroscience, 2(1), 1-27.
[19] Gokce, A. (2017). The interfacial dynamics of Amari type neural field models on finite domains. (Doctoral dissertation, University of Nottingham).
[20] Laing, C. R., & Troy, W. C. (2003). PDE methods for nonlocal models. SIAM Journal on Applied Dynamical Systems, 2(3), 487- 516
[21] Laing, C. R. (2005). Spiral waves in nonlocal equations. SIAM Journal on Applied Dynamical Systems, 4(3), 588-606.
[22] Coombes, S., beim Graben, P., Potthast, R., & Wright, J. (Eds.). (2014). Neural Fields: Theory and Applications. Springer.
[23] Fedak, A. (2018). A compact fourth-order finite volume method for structured curvilinear grids. University of California, Davis.
[24] Cueto-Felgueroso, L. (2009). Finite volume methods for one-dimensional scalar conservation laws. http://juanesgroup.mit.edu/lc ueto/teach.
[25] Zoppou, C., Knight, J. H. (1999). Analytical solution of a spatially variable coefficient advection–diffusion equation in up to three dimensions. Applied Mathematical Modelling, 23(9), 667-685.
[26] Eftekhari, A.A. et al. (2015). FVTool: a finite volume toolbox for Matlab. Zenodo. http: //doi.org/10.5281/zenodo.32745
[27] Nordbotten, J. M. (2014). Cell-centered finite volume discretizations for deformable porous media. International Journal for Numerical Methods in Engineering, 100(6), 399-418.
[28] Mungkasi, S. (2008). Finite volume methods for the one-dimensional shallow water equations. (M. Math. Sc. thesis, Australian National University).
[29] Eymard, R., Gallou¨et, T., Herbin, R., Latch´e, J. C. (2007). Analysis tools for finite volume schemes. Proceedings of Equa Diff 11, 111-136.
[30] Versteeg, H. K., Malalasekera, W. (2007). An introduction to computational fluid dynamics: the finite volume method. Pearson Education.
[31] Abbott, M. B., Basco, D. R. (1997). Computational fluid dynamics: an introduction for engineers. Longman.
[32] Patankar, S. V. (1991). Computation of conduction and duct flow heat transfer. CRC Press.
[33] Patankar, S. V. (2018). Numerical heat transfer and fluid flow. CRC Press.
[34] Patankar, S. V. (1981). A calculation procedure for two-dimensional elliptic situations. Numerical Heat Transfer, 4(4), 409-425.
[35] Evans, L.C. (2010). Partial differential equations. American Mathematical Society, Providence, Rhode Island.
[36] Anderson, J. D., Wendt, J. (1995). Computational fluid dynamics (Vol. 206, p. 332). New York: McGraw-Hill.
[37] Morton, K. W., & Mayers, D. F. (2005). Numerical solution of partial differential equations: an introduction. Cambridge University Press.
[38] LeVeque, R. J. (2007). Finite difference methods for ordinary and partial differential equations: steady-state and time-dependent problems. Society for Industrial and Applied Mathematics.
[39] LeVeque, R. J. (2002). Finite volume methods for hyperbolic problems (Vol. 31). Cambridge university press.
[40] Badr, M., Yazdani, A., & Jafari, H. (2018). Stability of a finite volume element method for the time-fractional advection-diffusion equation. Numerical Methods for Partial Differential Equations, 34(5), 1459-1471.
[41] Syrakos, A., Goulas, A. (2006). Estimate of the truncation error of finite volume discretization of the Navier–Stokes equations on colocated grids. International journal for numerical methods in fluids, 50(1), 103-130.
[42] Fraysse, F., de Vicente, J., Valero, E. (2012). The estimation of truncation error by τ - estimation revisited. Journal of Computational Physics, 231(9), 3457-3482.
[43] Ermentrout, G. B., Folias, S. E., & Kilpatrick, Z. P. (2014). Spatiotemporal pattern formation in neural fields with linear adaptation. In Neural Fields (pp. 119-151). Springer, Berlin, Heidelberg.
[44] Benda, J., & Herz, A. V. (2003). A universal model for spike-frequency adaptation. Neural Computation, 15(11), 2523-2564.