Multi-objective regression modeling for natural gas prediction with ridge regression and CMARS
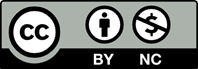
Residential customers are the main users generally need a great quantity of natural gas in distribution systems, especially, in the wintry weather season since it is particularly consumed for cooking and space heating. Hence, it ought to be noninterruptible. Since distribution systems have a restricted ability for supply, reasonable planning and prediction through the whole year, especially in winter seasons, have emerged as vital. The Ridge Regression (RR) is formulated mainly to decrease collinearity results through shrinking the regression coefficients and reducing the impact in the model of variables. Conic multivariate adaptive regression splines ((C)MARS) model is constructed as an effective choice for MARS by using inverse problems, statistical learning, and multi-objective optimization theories. In this approach, the model complexity is penalized in the structure of RR and it is constructed a relaxation by utilizing continuous optimization, called Conic Quadratic Programming (CQP). In this study, CMARS and RR are applied to obtain forecasts of residential natural gas demand for local distribution companies (LDCs) that require short-term forecasts, and the model performances are compared by using some criteria. Here, our analysis shows that CMARS models outperform RR models. For one-day-ahead forecasts, CMARS yields a MAPE of about 4.8%, while the same value under RR reaches 8.5%. As the forecast horizon increases, it can be seen that the performance of the methods becomes worse, and for a forecast one week ahead, the MAPE values for CMARS and RR are 9.9% and 18.3%, respectively.
[1] Özmen, A., (2021). Sparse regression modeling for short- and long‐term natural gas demand prediction. Annals Operations Research, DOI: https://doi.org/10.1007/s10479-021-04089-x
[2] Özmen, A., Yılmaz Y., & Weber, G.-W. (2018). Natural Gas Consumption Forecasting Model for Residential User. Energy Economics, 70, 357–381.
[3] Aster, R.C., Borchers, B., & Thurber C.H. (2013). Parameter Estimation and Inverse Problems. Academic Press, Boston.
[4] James, G., Witten, D., Hastie, T., & Tibshirani, R. (2013). An introduction of statistical learning, Springer.
[5] Wheeler, D. (2009). Simultaneous Coefficient Penalization and Model Selection in Geographically Weighted Regression: The Geographically Weighted Lasso. Environment and Planning A, 39(3), 722-742.
[6] Friedman, J.H. (1991). Multivariate adaptive regression splines. Annals of Statistics,19,1–67.
[7] Taylan, P., Weber, G.-W., & Beck, A. (2007). A new approach to regression by generalized additive models and continuous optimization for modern applications in finance, science and technology, Optimization. 56, 5-6, 675–698.
[8] Taylan, P., Weber, G. W., & Özkurt, F. Y. (2010). A new approach to multivariate adaptive regression splines by using Tikhonov regularization and continuous optimization. Top, 18(2), 377-395.
[9] Weber, G.-W., Batmaz, İ., Köksal, G., Taylan, P., & Yerlikaya-Özkurt, F. (2012). CMARS: a new contribution to nonparametric regression with multivariate adaptive regression splines supported by continuous optimization. Inverse Problems in Science and Engineering, 20(3), 371-400.
[10] Ayyıldız, E., Purutçuoğlu, V., & Weber, G. W. (2018). Loop-based conic multivariate adaptive regression splines is a novel method for advanced construction of complex biological networks. European Journal of Operational Research, 270(3), 852-861.
[11] Roos, C., Terlaky, T., & Vial, J. (1997). Interior Point Approach to Linear Optimization: Theory and Algorithms, John Wiley & Sons, New York.
[12] Roos, C., Terlaky, T., & Vial, J. (2006). Interior Point Methods for Linear Optimization. Springer Science, Heidelberg/Boston.
[13] MOSEK; software available at http://www.mosek.com.
[14] Tibshirani, R.(1996). Regression shrinkage and selection via the lasso. Journal of the Royal Statistical Soceity B, 267-288.
[15] Hastie, T., Tibshirani, R. & Friedman, J.H. (2001). The Element of Statistical Learning. Springer Seriesin Statistics; New York, USA.
[16] Özmen, A., Kropat, E., & Weber, G.-W. (2014b). Spline regression models for complex multi-modal regulatory networks. Optimization Methods and Software (OMS) 29(3), 515-534.
[17] Kriner, M. (2007). Survival Analysis with Multivariate Adaptive Regression Splines, Dissertation, LMU Munchen: Faculty of Mathematics, Computer Science and Statistics.
[18] Hastie, T. & Tibshirani, R.J. (1996). Discriminant analysis by Gaussian mixtures, Journal of the Royal Statistical Society (Ser. B) 58, 155–176.
[19] Leathwicka, J.R., Elith, J., & Hastie, T. (1993). Comparative performance of generalized additive models and multivariate adaptive regression splines for statistical modelling of species distributions, Ecological Modelling 199 (2), 188-196.
[20] Kuter, S., Weber, G.-W., Özmen, A.,& Akyürek, Z. (2014). Modern applied mathematics for alternative modelling of the atmospheric effects on satellite images, Modeling, Optimization, Dynamics and Bioeconomy I, Springer Proceedings in Mathematics & Statistics, 73, 469-485.
[21] Gürbüz, B., & Sezer, M. (2020). Modified operational matrix method for second-order nonlinear ordinary differential equations with quadratic and cubic terms. An International Journal of Optimization and Control: Theories & Applications (IJOCTA), 10(2), 218-225.
[22] Salford Systems-Data Mining and Predictive Analytics Software: MARS software available at http://www.salfordsystems.com.
[23] Weber, G.-W., Çavuşoğlu, Z, & Özmen, A. (2012).Predicting default probabilities in emerging markets by conic generalized partial linear models and their optimization. Optimization, 61(4), 443- 457.
[24] Özmen A., Weber, G.-W., & Kropat, E. (2012). Robustification of conic generalized partial linear models under polyhedral uncertainty. International IFNA-ANS scientific Journal “Problems of Nonlinear Analysis in Engineering Systems”, 2(38), 104-113.
[25] Özmen, A., Weber, G.-W., Çavuşoğlu, Z., & Defterli, Ö. (2013). The new robust conic GPLM method with an Application to Finance: prediction of credit default. Journal of Global Optimization (JOGO), 56 (2), 233-249.
[26] Özmen, A. (2016). Robust optimization of spline models and complex regulatory networks - theory, methods and application. Contribution to Management Science, Springer.
[27] Özmen, A., Weber, G.-W., Batmaz, I., & Kropat, E. (2011). RCMARS: Robustification of CMARS with Different Scenarios under Polyhedral Uncertainty Set. Communications in Nonlinear Science and Numerical Simulation (CNSNS) 16 (12), 4780-4787.