Data-driven prediction of strain fields in auxetic structures and non-contact validation with mechanoluminescence for structural health monitoring
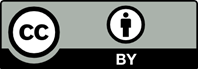
Recent advancements in 3D printing technology have significantly enhanced the potential of auxetic structures, which are notable for their negative Poisson’s ratio, particularly in applications such as sensor technology and structural health monitoring. Central to the performance of these structures is the accurate estimation of the effective strain parameter, a critical metric for assessing structural integrity. However, as structural complexity increases, estimating this parameter becomes increasingly challenging. The fabrication and real-world validation of these structures are equally important challenges. This paper introduces two key innovations for the practical application of auxetic structures. First, we present a multi-kernel hierarchical deep neural network that leverages finite element simulation data to accurately predict effective strain fields in complex auxetic configurations. This model architecture not only reduces the number of parameters requiring training but also enhances feature learning and generalization capabilities, achieving over 90% accuracy in predicting strain fields. Second, we validate these predictions using a 3D-printed specimen embedded with mechanoluminescent (ML) particles. This approach enables direct, non-contact visualization of strain in real-time, offering high spatial and temporal resolution. The alignment observed between predicted and observed strain concentration areas demonstrates the efficacy of integrating ML technology into auxetic designs. This integration significantly improves the reliability and diagnostic capabilities of advanced structural systems.
- Zhang XY, Ren X, Zhang Y, Xie YM. A novel auxetic metamaterial with enhanced mechanical properties and tunable auxeticity. Thin Walled Struct. 2022;174:109162. doi: 10.1016/j.tws.2022.109162
- Xin X, Liu L, Liu Y, Leng J. 4D printing auxetic metamaterials with tunable, programmable, and reconfigurable mechanical properties. Adv Funct Mater. 2020;30(43):2004226. doi: 10.1002/adfm.202004226
- Cheng X, Zhang Y, Ren X, et al. Design and mechanical characteristics of auxetic metamaterial with tunable stiffness. Int J Mech Sci. 2022;223:107286. doi: 10.1016/j.ijmecsci.2022.107286
- Ilani MA, Khoshnevisan M. Powder mixed-electrical discharge machining (EDM) with the electrode is made by fused deposition modeling (FDM) at Ti-6Al-4V machining procedure. Multiscale Multidiscip Model Exp Des. 2020;3(3):173-186. doi: 10.1007/s41939-020-00070-6
- Wang Z, Luan C, Liao G, Liu J, Yao X, Fu J. Progress in auxetic mechanical metamaterials: Structures, characteristics, manufacturing methods, and applications. Adv Eng Mater. 2020;22(10):2000312. doi: 10.1002/adem.202000312
- Yu X, Zhou J, Liang H, Jiang Z, Wu L. Mechanical metamaterials associated with stiffness, rigidity and compressibility: A brief review. Prog Mater Sci. 2018;94: 114-173. doi: 10.1016/j.pmatsci.2017.12.003
- Evans KE, Alderson A. Auxetic materials: Functional materials and structures from lateral thinking! Adv Mater. 2000;12(9):617-628. doi: 10.1002/(SICI)1521-4095(200005)12:9<617:AID-ADMA617>3.0.CO;2-3
- Li Y, Luo S, Yang MC, Liang R, Zeng C. Poisson ratio and piezoresistive sensing: A New route to high-performance 3D flexible and stretchable sensors of multimodal sensing capability. Adv Funct Mater. 2016;26(17):2900-2908. doi: 10.1002/adfm.201505070
- Zhai X, Gao J, Liao H, Kirk CD, Balogun YA, Chen WW. Mechanical behaviors of auxetic polyurethane foam at quasi-static, intermediate and high strain rates. Int J Impact Eng. 2019;129:112-118. doi: 10.1016/j.ijimpeng.2019.03.002
- Grima JN, Evans KE. Auxetic behavior from rotating squares. J Mater Sci Lett. 2000;19:1563-1565. doi: 10.1023/A:1006781224002
- Tretiakov KV. Negative poisson’s ratio of two-dimensional hard cyclic tetramers. J Non Cryst Solids. 2009;355(24- 27):1435-1438. doi: 10.1016/j.jnoncrysol.2009.05.043
- Shin HG, Timilsina S, Sohn KS, Kim JS. Digital image correlation compatible mechanoluminescent skin for structural health monitoring. Adv Sci. 2022;9(11):2105889. doi: 10.1002/advs.202105889
- Feng A, Smet PF. A review of mechanoluminescence in inorganic solids: Compounds, mechanisms, models and applications. Materials (Basel). 2018;11(4):484. doi: 10.3390/ma11040484
- Timilsina S, Kim JS, Kim J, Kim GW. Review of state-of-the-art sensor applications using mechanoluminescence microparticles. Int J Precis Eng Manuf. 2016;17(9): 1237-1247. doi: 10.1007/s12541-016-0149-y
- Zhang JC, Wang X, Marriott G, Xu CN. Trap-controlled mechanoluminescent materials. Prog Mater Sci. 2019;103:678-742. doi: 10.1016/j.pmatsci.2019.02.001
- Ni S, Zhu H, Neuzil P, Yobas L. A SiN microcalorimeter and a non-contact precision method of temperature calibration. J Microelectromech Syst. 2020;29(5):1103-1105. doi: 10.1109/JMEMS.2020.3008867
- Ennaceur C, Laksimi A, Hervé C, Cherfaoui M. Monitoring crack growth in pressure vessel steels by the acoustic emission technique and the method of potential difference. Int J Press Vessels Piping. 2006;83(3):197-204. doi: 10.1016/j.ijpvp.2005.12.004
- Wang JY, Guo JY. Damage investigation of ultra high performance concrete under direct tensile test using acoustic emission techniques. Cem Concr Compos. 2018;88:17-28. doi: 10.1016/j.cemconcomp.2018.01.007
- Wen TK, Yin CC. Crack detection in photovoltaic cells by interferometric analysis of electronic speckle patterns. Sol Energy Mater Sol Cells. 2012;98:216-223. doi: 10.1016/j.solmat.2011.10.034
- Zarate EA, Custodio GE, Treviño-Palacios CG, Rodríguez- Vera R, Puga-Soberanes HJ. Defect detection in metals using electronic speckle pattern interferometry. Sol Energy Mater Sol Cells. 2005;88(2):217-225. doi: 10.1016/j.solmat.2004.03.009
- Liang Z, Qin F, Zheng Y, Zhang Z, Cao W. Noncontact thermometry based on downconversion luminescence from Eu3+ doped LiNbO3 single crystal. Sens Actuators A Phys. 2016;238:215-219. doi: 10.1016/j.sna.2015.12.018
- Antić Ž, Dramićanin MD, Prashanthi K, Jovanović D, Kuzman S, Thundat T. Pulsed laser deposited dysprosium-doped gadolinium–vanadate thin films for noncontact, self-referencing luminescence thermometry. Adv Mater. 2016;28(35):7745-7752. doi: 10.1002/adma.201601176
- Schlothauer JC, Grabmayer K, Hintersteiner I, Wallner GM, Röder B. Non-destructive 2D-luminescence detection of EVA in aged PV modules: Correlation to calorimetric properties, additive distribution and a clue to aging parameters. Sol Energy Mater Sol Cells. 2017;159:307-317. doi: 10.1016/j.solmat.2016.09.011
- Stepanova LV, Yakovleva EM. Asymptotic stress field in the vicinity of a mixed-mode crack under plane stress conditions for a power-law hardening material. J Mech Mater Struct. 2015;10(3):367-393. doi: 10.2140/jomms.2015.10.367
- Berto F, Lazzarin P. Recent developments in brittle and quasi-brittle failure assessment of engineering materials by means of local approaches. Mater Sci Eng R Rep. 2014;75(1):1-48 doi: 10.1016/j.mser.2013.11.001
- Altenbach H, Hitzler L, Johlitz M, Merkel M, Öchsner A, editors. Lectures Notes on Advanced Structured Materials 2. Vol. 203. Switzerland: Springer Nature; 2024. doi: 10.1007/978-3-031-49043-9
- Qin Y, Singh SC, Grevemeyer I, Marjanović M, Roger Buck W. Discovery of flat seismic reflections in the mantle beneath the young Juan de Fuca Plate. Nat Commun. 2020;11(1):4122. doi: 10.1038/s41467-020-17946-3
- Yang Z, Yabansu YC, Jha D, et al. Establishing structure-property localization linkages for elastic deformation of three-dimensional high contrast composites using deep learning approaches. Acta Mater. 2019;166:335-345. doi: 10.1016/j.actamat.2018.12.045
- Fu T, Monaco F, Li J, et al. Deep-learning-enabled crack detection and analysis in commercial lithium-ion battery cathodes. Adv Funct Mater. 2022;32(39):2203070. doi: 10.1002/adfm.202203070
- Park D, Park M, Ryu S. Expanding design spaces in digital composite materials: A multi-input deep learning approach enhanced by transfer learning and multi-kernel network. Adv Theory Simul. 2023;6(11):2300465. doi: 10.1002/adts.202300465
- Ammasai Sengodan G. Prediction of two-phase composite microstructure properties through deep learning of reduced dimensional structure-response data. Compos B Eng. 2021;225:109282. doi: 10.1016/j.compositesb.2021.109282
- Lee J, Park D, Lee M, et al. Machine learning-based inverse design methods considering data characteristics and design space size in materials design and manufacturing: A review. Mater Horiz. 2023;10(12):5436-5456. doi: 10.1039/d3mh00039g
- Tan RK, Zhang NL, Ye W. A deep learning-based method for the design of microstructural materials. Struct Multidiscip Optim. 2020;61(4):1417-1438. doi: 10.1007/s00158-019-02424-2
- Li HN, Ren L, Jia ZG, Yi TH, Li DS. State-of-the-art in structural health monitoring of large and complex civil infrastructures. J Civil Struct Health Monit. 2016;6(1):3-16. doi: 10.1007/s13349-015-0108-9
- Diamanti K, Soutis C. Structural health monitoring techniques for aircraft composite structures. Prog Aerosp Sci. 2010;46(8):342-352. doi: 10.1016/j.paerosci.2010.05.001
- Ye XW, Su YH, Han JP. Structural health monitoring of civil infrastructure using optical fiber sensing technology: A comprehensive review. Sci World J. 2014;2014:652329. doi: 10.1155/2014/652329
- Li C, He Q, Wang Y, et al. Highly robust and soft biohybrid mechanoluminescence for optical signaling and illumination. Nat Commun. 2022;13(1):3914. doi: 10.1038/s41467-022-31705-6
- Song H, Park E, Kim HJ, et al. Free-form optimization of pattern shape for improving mechanical characteristics of a concentric tube. Mater Des. 2023;230:111974. doi: 10.1016/j.matdes.2023.111974
- Lee S, Choi W, Park JW, et al. Machine learning-enabled development of high performance gradient-index phononic crystals for energy focusing and harvesting. Nano Energy. 2022;103:107846. doi: 10.1016/j.nanoen.2022.107846
- Park D, Jung J, Gu GX, Ryu S. A generalizable and interpretable deep learning model to improve the prediction accuracy of strain fields in grid composites. Mater Des. 2022;223:111192. doi: 10.1016/j.matdes.2022.111192
- Luján-García JE, Yáñez-Márquez C, Villuendas-Rey Y, Camacho-Nieto O. A transfer learning method for pneumonia classification and visualization. Appl Sci (Basel). 2020;10(8):2908. doi: 10.3390/APP10082908
- Reddy CKA, Xiaochuan N, Zou W, et al. ICASSP 2021 Deep Noise Suppression Challenge. In: Proceedings of the IEEE International Conference on Acoustics, Speech and Signal Processing (ICASSP). IEEE; 2021. p. 6628-6632. doi: 10.1109/ICASSP39728.2021.9413831
- Laureto JJ, Pearce JM. Anisotropic mechanical property variance between ASTM D638-14 type I and type IV fused filament fabricated specimens. Polym Test. 2018;68:294-301. doi: 10.1016/j.polymertesting.2018.04.029
- Yoo JC, Han TH. Fast normalized cross-correlation. Circuits Syst Signal Process. 2009;28(6):819-843. doi: 10.1007/s00034-009-9130-7
- Song H, Timilsina S, Jung J, Kim TS, Ryu S. Improving the sensitivity of the mechanoluminescence composite through functionalization for structural health monitoring. ACS Appl Mater Interfaces. 2022;14(26):30205-30215. doi: 10.1021/acsami.2c07286
- Qi C, Jiang F, Yang S. Advanced honeycomb designs for improving mechanical properties: A review. Compos B Eng. 2021;227:109393. doi: 10.1016/j.compositesb.2021.109393
- Han D. Comparison of commonly used image interpolation methods. In: Proceedings of the 2nd International Conference on Computer Science and Electronics Engineering (ICCSEE 2013). Atlantis Press; 2013. p. 1556-1559.