Machine learning techniques for quality assurance in additive manufacturing processes
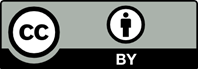
Additive manufacturing (AM) processes have revolutionized manufacturing industries by enabling the production of complex geometries with reduced material waste and lead times. However, ensuring the quality of AM parts remains a significant challenge due to the complexity of the process and inherent variability in material properties. This review investigates the use of artificial intelligence (AI) to enhance quality assurance in AM processes, focusing on specific machine learning techniques such as convolutional neural networks for defect detection, support vector machines for classification of material properties, and reinforcement learning for real-time process optimization. The AI-driven methodologies are applied to predict defects, optimize process parameters, and monitor real-time production quality, utilizing large datasets generated from sensors and in-situ monitoring systems. The study demonstrates significant improvements in the accuracy of defect detection, the reliability of material property classification, and the efficiency of process optimization. In addition, it addresses challenges such as data pre-processing, model interpretability, and integration with existing AM systems. The findings highlight the potential of AI to transform quality assurance in AM and outline future research directions for further integration and enhancement of AI techniques in AM.
- Balhara H, Karthikeyan A, Hanchate A, Nakkina TG, Bukkapatnam ST. Imaging systems and techniques for fusion-based metal additive manufacturing: A review. Front Manuf Technol. 2023;3:1271190. doi: 10.3389/fmtec.2023.1271190
- Goswami SS, Sarkar S, Gupta KK, Mondal S. The role of cyber security in advancing sustainable digitalization: Opportunities and challenges. J Decis Anal Intell Comput. 2023;3(1):270-285. doi: 10.31181/jdaic10018122023g
- Ionașcu AE, Goswami SS, Dănilă A, Horga MG, Barbu C, Adrian ŞC. Analyzing primary sector selection for economic activity in Romania: An interval-valued fuzzy multi-criteria approach. Mathematics. 2024;12(8):1157. doi: 10.3390/math12081157
- Sahoo SK, Goswami SS. Green supplier selection using MCDM: A comprehensive review of recent studies. Spectr Eng Manag Sci. 2024;2(1):1-16. doi: 10.31181/sems1120241a
- Plathottam SJ, Rzonca A, Lakhnori R, Iloeje CO. A review of artificial intelligence applications in manufacturing operations. J Adv Manuf Process. 2023;5(3):e10159. doi: 10.1002/amp2.10159
- Sahoo SK, Goswami SS, Sarkar S, Mitra S. A review of digital transformation and industry 4.0 in supply chain management for small and medium-sized enterprises. Spectr Eng Manag Sci. 2023;1(1):58-72. doi: 10.31181/sems1120237j
- Elahi M, Afolaranmi SO, Lastra JL, Perez Garcia JA. A comprehensive literature review of the applications of AI techniques through the lifecycle of industrial equipment. Discov Artif Intell. 2023;3(1):43. doi: 10.1007/s44163-023-00089-x
- Sahoo SK, Goswami SS, Halder, R. Supplier selection in the age of industry 4.0: A review on MCDM applications and trends. Decis Mak Adv. 2024;2(1):32-47. doi: 10.31181/dma21202420
- Kim H, Lin Y, Tseng TL. A review on quality control in additive manufacturing. Rapid Prototyp J. 2018;24(3):645-669. doi: 10.1108/RPJ-03-2017-0048
- Qin J, Hu F, Liu Y, et al. Research and application of machine learning for additive manufacturing. Addit Manuf. 2022;52:102691. doi: 10.1016/j.addma.2022.102691
- Bonatti AF, Vozzi G, De Maria C. Enhancing quality control in bioprinting through machine learning. Biofabrication. 2024;16(2):022001. doi: 10.1088/1758-5090/ad2189
- Jin Z, Zhang Z, Gu GX. Automated real‐time detection and prediction of interlayer imperfections in additive manufacturing processes using artificial intelligence. Adv Intell Syst. 2020;2(1):1900130. doi: 10.1002/aisy.201900130
- Bikas H, Terzakis MA, Stavropoulos P. Manufacturability-based design optimization for directed energy deposition processes. Machines. 2023;11(9):879. doi: 10.3390/machines11090879
- Stavropoulos P, Tzimanis K, Souflas T, Bikas H. Knowledge-based manufacturability assessment for optimization of additive manufacturing processes based on automated feature recognition from CAD models. Int J Adv Manuf Technol. 2022;122(2):993-1007. doi: 10.1007/s00170-022-09948-w
- Wang C, Tan XP, Tor SB, Lim CS. Machine learning in additive manufacturing: State-of-the-art and perspectives. Addit Manuf. 2020;36:101538. doi: 10.1016/j.addma.2020.101538
- Stavropoulos P, Souflas T, Papaioannou C, Bikas H, Mourtzis D. An adaptive, artificial intelligence-based chatter detection method for milling operations. Int J Adv Manuf Technol. 2023;124(7):2037-2058. doi: 10.1007/s00170-022-09920-8
- Stavropoulos P, Foteinopoulos P, Papapacharalampopoulos A. On the impact of additive manufacturing processes complexity on modelling. Appl Sci. 2021;11(16):7743. doi: 10.3390/app11167743
- Talaat FM, Hassan E. Artificial intelligence in 3D printing. In: Hassanien AE, Darwish A, El-Kader SM, Alboaneen DA, editors. Enabling Machine Learning Applications in Data Science. Algorithms for Intelligent Systems. Singapore: Springer; 2021. doi: 10.1007/978-981-33-6129-4_6
- Rojek I, Mikołajewski D, Dostatni E, Macko M. AI-optimized technological aspects of the material used in 3D printing processes for selected medical applications. Materials. 2020;13(23):5437. doi: 10.3390/ma13235437
- Kantaros A, Ganetsos T. From static to dynamic: Smart materials pioneering additive manufacturing in regenerative medicine. Int J Mol Sci. 2023;24(21):15748. doi: 10.3390/ijms242115748
- Kantaros A, Petrescu FI, Abdoli H, et al. Additive manufacturing for surgical planning and education: A review. Appl Sci. 2024;14(6):2550. doi: 10.3390/app14062550
- Kantaros A, Piromalis D, Tsaramirsis G, Papageorgas P, Tamimi H. 3D printing and implementation of digital twins: Current trends and limitations. Appl Syst Innov. 2021;5(1):7. doi: 10.3390/asi5010007
- Hunde BR, Woldeyohannes AD. Future prospects of computer-aided design (CAD)-A review from the perspective of artificial intelligence (AI), extended reality, and 3D printing. Results Eng. 2022;14:100478. doi: 10.1016/j.rineng.2022.100478
- Zhu Z, Ng DW, Park HS, McAlpine MC. 3D-printed multifunctional materials enabled by artificial-intelligence-assisted fabrication technologies. Nat Rev Mater. 2021;6(1):27-47. doi: 10.1038/s41578-020-00235-2
- Caiazzo B, Murino T, Petrillo A, Piccirillo G, Santini S. An IoT-based and cloud-assisted AI-driven monitoring platform for smart manufacturing: Design architecture and experimental validation. J Manuf Technol Manag. 2023;34(4):507-534. doi: 10.1108/JMTM-02-2022-0092
- Yenugula M, Goswami SS, Kaliappan S, et al. Analyzing the critical parameters for implementing sustainable AI cloud system in an IT industry using AHP-ISM-MICMAC integrated hybrid MCDM model. Mathematics. 2023;11(15):3367. doi: 10.3390/math11153367
- Rahman MA, Saleh T, Jahan MP, et al. Review of intelligence for additive and subtractive manufacturing: Current status and future prospects. Micromach. 2023;14(3):508. doi: 10.3390/mi14030508
- Alshahrani R, Yenugula M, Algethami H, et al. Establishing the fuzzy integrated hybrid MCDM framework to identify the key barriers to implementing artificial intelligence-enabled sustainable cloud system in an IT industry. Exp Syst Appl. 2024;238:121732. doi: 10.1016/j.eswa.2023.121732
- Jan Z, Ahamed F, Mayer W, et al. Artificial intelligence for industry 4.0: Systematic review of applications, challenges, and opportunities. Exp Syst Appl. 2023;216:119456. doi: 10.1016/j.eswa.2022.119456
- Sahoo SK, Das AK, Samanta S, Goswami SS. Assessing the role of sustainable development in mitigating the issue of global warming. J Process Manag New Technol. 2023;11(1-2):1-21. doi: 10.5937/jpmnt11-44122
- Castañé G, Dolgui A, Kousi N, et al. The assistant project: AI for high level decisions in manufacturing. Int J Prod Res. 2023;61(7):2288-2306. doi: 10.1080/00207543.2022.2069525
- Wang K, Ying Z, Goswami SS, Yin Y, Zhao Y. Investigating the role of artificial intelligence technologies in the construction industry using a Delphi-ANP-TOPSIS hybrid MCDM concept under a fuzzy environment. Sustainability. 2023;15(15):11848. doi: 10.3390/su151511848
- Mittal U, Yang H, Bukkapatnam ST, Barajas LG. Dynamics and Performance Modeling of Multi-stage Manufacturing Systems Using Nonlinear Stochastic Differential Equations. IEEE International Conference on Automation Science and Engineering. 2008. p. 498-503. doi: 10.1109/COASE.2008.4626530
- Wei AT, Wang H, Dickens T, Chi H. Co-learning of extrusion deposition quality for supporting interconnected additive manufacturing systems. IISE Transac. 2023;55(4):405-418. doi: 10.1080/24725854.2022.2080306
- Mittal U, Panchal D. AI-based evaluation system for supply chain vulnerabilities and resilience amidst external shocks: An empirical approach. Rep Mech Eng. 2023;4(1):276-289. doi: 10.31181/rme040122112023m
- Shafi I, Mazhar MF, Fatima A, et al. Deep learning-based real time defect detection for optimization of aircraft manufacturing and control performance. Drones. 2023;7(1):31. doi: 10.3390/drones7010031
- Mittal U. Detecting Hate Speech Utilizing Deep Convolutional Network and Transformer Models. International Conference on Electrical, Electronics, Communication and Computers. 2023. p. 1-4. doi: 10.1109/ELEXCOM58812.2023.10370502