Advancing sustainability: Biodegradable electronics and materials discovery through artificial intelligence
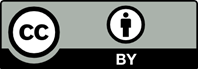
The pressing need for sustainable materials and devices stems from growing environmental concerns and the imperative to mitigate climate change. Traditional materials and devices often rely on non-renewable resources and generate significant waste and pollution throughout their lifecycle. By prioritizing sustainability in material and device design, we can foster innovation, promote circular economies, and build a greener future for generations to come. Artificial intelligence (AI) and machine learning (ML) can analyze vast datasets to identify novel materials with desirable properties by reducing the experimental workload. In this paper, we explore the synergistic relationship between sustainable materials discovery and ML models. By leveraging advanced algorithms, researchers can efficiently explore vast chemical spaces to identify environmentally friendly materials with tailored properties. ML techniques, including predictive modeling and generative models, facilitate the rapid discovery and optimization of sustainable materials for various applications, ranging from renewable energy technologies to eco-friendly consumer products. We present a landscape view of the field with a focus on the most recent developments, focusing mainly on transitory materials such as metals, polymers, and semiconducting materials. Furthermore, classification and regression techniques to model the degradation behavior of polymers have been addressed, pointing to key challenges and proposing solutions for enhanced ML applications. The paper discusses the challenges of scaling up data-driven technologies from small molecules to polymers, underscoring AI’s role in discovering new molecular designs and optimizing existing ones for novel applications. It emphasizes the importance of defining and standardizing polymer systems to enable ML models to create a unified data collection system for AI and automation enhancements. Furthermore, it stresses the necessity of refining ML methods to harness the benefits of data-driven polymer chemistry fully, emphasizing the importance of reliable and diverse datasets for predictive models in polymer synthesis.
- Giddings B, Hopwood B, O’Brien G. Environment, economy and society: Fitting them together into sustainable development. Sustain Dev. 2002;10(4):187-196. doi: 10.1002/sd.199
- Li W, Liu Q, Zhang Y, et al. Biodegradable materials and green processing for green electronics. Adv Mater. 2020;32(33):e2001591. doi: 10.1002/adma.202001591
- Li R, Wang L, Kong D, Yin L. Recent progress on biodegradable materials and transient electronics. Bioact Mater. 2018;3(3):322-333. doi: 10.1016/j.bioactmat.2017.12.001
- Fu KK, Wang Z, Dai J, Carter M, Hu L. Transient electronics: Materials and devices. Chem Mater. 2016;28(11):3527-3539. doi: 10.1021/acs.chemmater.5b04931
- Surge in Global E-waste, Up 21 Per Cent in 5 Years. Available from: https://globalewaste.org/news/surge-global-waste [Last accessed on 2024 Jul 02].
- Liu S, Yang DS, Wang S, et al. Soft, environmentally degradable microfluidic devices for measurement of sweat rate and total sweat loss and for colorimetric analysis of sweat biomarkers. EcoMat. 2023;5(1):e12270. doi: 10.1002/eom2.12270
- Zhao X, Hu C, Pan G, Cui W. Pomegranate-structured electrosprayed microspheres for long-term controlled drug release. Part Part Syst Charact. 2015;32(5):529-535. doi: 10.1002/ppsc.201400199
- Yuan Z, Zhao X, Zhao J, et al. Synergistic mediation of tumor signaling pathways in hepatocellular carcinoma therapy via dual-drug-loaded PH-responsive electrospun fibrous scaffolds. J Mater Chem B. 2015;3(17):3436-3446. doi: 10.1039/c5tb00206k
- Tyler B, Gullotti D, Mangraviti A, Utsuki T, Brem H. Polylactic acid (PLA) controlled delivery carriers for biomedical applications. Adv Drug Deliv Rev. 2016;107:163-175. doi: 10.1016/j.addr.2016.06.018
- Fernández-Calderón MC, Romero-Guzmán D, Ferrández- Montero A, et al. Impact of PLA/Mg films degradation on surface physical properties and biofilm survival. Colloids Surf B Biointerfaces. 2020;185:110617. doi: 10.1016/j.colsurfb.2019.110617
- Yin L, Cheng H, Mao S, et al. Dissolvable metals for transient electronics. Adv Funct Mater. 2014l;24(5):645-658. doi: 10.1002/adfm.201301847
- Fernandes C, Taurino I. Biodegradable Molybdenum (Mo) and Tungsten (W) devices: One step closer towards fully-transient biomedical implants. Sensors (Basel). 2022;22(8):3062. doi: 10.3390/s22083062
- Cha GD, Kang D, Lee J, Kim D. Bioresorbable electronic implants: History, materials, fabrication, devices, and clinical applications. Adv Healthc Mater. 2019;8(11):e1801660. doi: 10.1002/adhm.201801660
- Jeong H, Baek S, Han S, Jang H, Kim SH, Lee HS. Novel eco-friendly starch paper for use in flexible, transparent, and disposable organic electronics. Adv Funct Mater. 2018;28(3):1704433. doi: 10.1002/adfm.201704433
- Luo Q, Hossen MA, Zeng Y, et al. Gelatin-based composite films and their application in food packaging: A review. J Food Eng. 2022;313:110762. doi: 10.1016/j.jfoodeng.2021.110762
- Hwang SW, Song JK, Huang X, et al. High-Performance biodegradable/transient electronics on biodegradable polymers. Adv Mater. 2014;26(23):3905-3911. doi: 10.1002/adma.201306050
- Chatterjee S, Saxena M, Padmanabhan D, Jayachandra M, Pandya HJ. Futuristic medical implants using bioresorbable materials and devices. Biosens Bioelectron. 2019;142:111489. doi: 10.1016/j.bios.2019.111489
- Phan HP. Implanted flexible electronics: Set device lifetime with smart nanomaterials. Micromachines (Basel). 2021;12(2):157. doi: 10.3390/mi12020157
- Samantaray PK, Little A, Haddleton DM, et al. Poly(Glycolic Acid) (PGA): A versatile building block expanding high performance and sustainable bioplastic applications. Green Chem. 2020;22(13):4055-4081. doi: 10.1039/d0gc01394c
- Wang G, Huang D, Ji J, Völker C, Wurm FR. Seawater-degradable polymers-fighting the marine plastic pollution. Adv Sci (Weinh). 2021;8(1):2001121. doi: 10.1002/advs.202001121
- Acar H, Çinar S, Thunga M, Kessler MR, Hashemi N, Montazami R. Study of physically transient insulating materials as a potential platform for transient electronics and bioelectronics. Adv Funct Mater. 2014;24(26):4135-4143. doi: 10.1002/adfm.201304186
- Sander M, Weber M, Lott C, Zumstein M, Künkel A, Battagliarin G. Polymer Biodegradability 2.0: A Holistic View on Polymer Biodegradation in Natural and Engineered Environments. Cham: Springer; 2023. p. 65-110. doi: 10.1007/12_2023_163
- Dagdeviren C, Hwang SW, Su Y, et al. Transient, biocompatible electronics and energy harvesters based on ZnO. Small. 2013;9(20):3398-3404. doi: 10.1002/smll.201300146
- Kang SK, Park G, Kim K, et al. Dissolution chemistry and biocompatibility of silicon- and germanium-based semiconductors for transient electronics. ACS Appl Mater Interfaces. 2015;7(17):9297-9305. doi: 10.1021/acsami.5b02526
- Hwang SW, Park G, Cheng H, et al. 25th anniversary article: Materials for high-performance biodegradable semiconductor devices. Adv Mater. 2014;26(13):1992-2000. doi: 10.1002/adma.201304821
- Yin L, Farimani AB, Min K, et al. Mechanisms for hydrolysis of silicon nanomembranes as used in bioresorbable electronics. Adv Mater. 2015;27(11):1857-1864. doi: 10.1002/adma.201404579
- Hwang SW, Park G, Edwards C, et al. Dissolution chemistry and biocompatibility of single-crystalline silicon nanomembranes and associated materials for transient electronics. ACS Nano. 2014;8(6):5843-5851. doi: 10.1021/nn500847g
- Lee YK, Yu KJ, Song E, et al. Dissolution of monocrystalline silicon nanomembranes and their use as encapsulation layers and electrical interfaces in water-soluble electronics. ACS Nano. 2017;11(12):12562-12572. doi: 10.1021/acsnano.7b06697
- Devabharathi N, Mondal SK, Dasgupta S. Inkjet-printed co-continuous mesoporous oxides for high-current power transistors. Nanoscale. 2019;11(29):13731-13740. doi: 10.1039/c9nr04876f
- Devabharathi N, Parasuraman R, Umarji AM, Dasgupta S. Ultra-high response ethanol sensors from fully-printed co-continuous and mesoporous tin oxide thin films. J Alloys Compd. 2021;865:158815. doi: 10.1016/j.jallcom.2021.158815
- Miyauchi M, Li Y, Shimizu H. Enhanced degradation in nanocomposites of TiO2 and biodegradable polymer. Environ Sci Technol. 2008;42(12):4551-4554. doi: 10.1021/es800097n
- Feig VR, Tran H, Bao Z. Biodegradable polymeric materials in degradable electronic devices. ACS Cent Sci. 2018;4(3):337-348. doi: 10.1021/acscentsci.7b00595
- Shi G, Rouabhia M, Wang Z, Dao LH, Zhang Z. A novel electrically conductive and biodegradable composite made of polypyrrole nanoparticles and polylactide. Biomaterials. 2004;25(13):2477-2488. doi: 10.1016/j.biomaterials.2003.09.032
- Lee JY, Bashur CA, Goldstein AS, Schmidt CE. Polypyrrole-coated electrospun PLGA nanofibers for neural tissue applications. Biomaterials. 2009;30(26):4325-4335. doi: 10.1016/j.biomaterials.2009.04.042
- Armelin E, Gomes AL, Pérez-Madrigal MM, et al. Biodegradable free-standing nanomembranes of conducting polymer: Polyester blends as bioactive platforms for tissue engineering. J Mater Chem. 2012;22(2):585-594. doi: 10.1039/c1jm14168f
- Li M, Guo Y, Wei Y, MacDiarmid AG, Lelkes PI. Electrospinning polyaniline-contained gelatin nanofibers for tissue engineering applications. Biomaterials. 2006;27(13):2705-2715. doi: 10.1016/j.biomaterials.2005.11.037
- Subramanian A, Krishnan UM, Sethuraman S. Axially aligned electrically conducting biodegradable nanofibers for neural regeneration. J Mater Sci Mater Med. 2012;23(7):1797-1809. doi: 10.1007/s10856-012-4654-y
- Lee S, Moon GD, Jeong U. Continuous production of uniform poly (3-Hexylthiophene) (P3HT) nanofibers by electrospinning and their electrical properties. J Mater Chem. 2009;19(6):743-748. doi: 10.1039/b814833c
- Pradhan S, Brooks AK, Yadavalli VK. Nature-derived materials for the fabrication of functional biodevices. Mater Today Bio. 2020;7:100065. doi: 10.1016/j.mtbio.2020.100065
- Herlogsson L, Crispin X, Robinson ND, et al. Low-voltage polymer field-effect transistors gated via a proton conductor. Adv Mater. 2007;19:97-101. doi: 10.1002/adma.200600871
- Minamiki T, Hashima Y, Sasaki Y, Minami T. An electrolyte-gated polythiophene transistor for the detection of biogenic amines in water. Chem Commun. 2018;54(50):6907-6910. doi: 10.1039/c8cc02462f
- Picca RA, Manoli K, Macchia E, et al. A study on the stability of water-gated organic field-effect-transistors based on a commercial p-type polymer. Front Chem. 2019;7:667. doi: 10.3389/fchem.2019.00667
- Rawlings D, Thomas EM, Segalman RA, Chabinyc ML. Controlling the doping mechanism in poly (3-Hexylthiophene) thin-film transistors with polymeric ionic liquid dielectrics. Chem Mater. 2019;31(21):8820-8829. doi: 10.1021/acs.chemmater.9b02803
- Kergoat L, Herlogsson L, Braga D, et al. A water-gate organic field-effect transistor. Adv Mater. 2010;22(23):2565-2569. doi: 10.1002/adma.200904163
- Vargason AM, Anselmo AC, Mitragotri S. The evolution of commercial drug delivery technologies. Nat Biomed Eng. 2021;5(9):951-967. doi: 10.1038/s41551-021-00698-w
- Boethling RS, Sommer E, DiFiore D. Designing small molecules for biodegradability. Chem Rev. 2007;107(6):2207-2227. doi: 10.1021/cr050952t
- Yang Y, Ko TP, Liu L, et al. Structural insights into enzymatic degradation of oxidized polyvinyl alcohol. Chembiochem. 2014;15(13):1882-1886. doi: 10.1002/cbic.201402166
- Stephen M, Nawaz A, Lee SY, Sonar P, Leong WL. Biodegradable materials for transient organic transistors. Adv Funct Mater. 2023;33(6):2208521. doi: 10.1002/adfm.202208521
- Kang SK, Hwang SW, Yu S, et al. Biodegradable thin metal foils and spin-on glass materials for transient electronics. Adv Funct Mater. 2015;25(12):1789-1797. doi: 10.1002/adfm.201403469
- Kang SK, Koo J, Lee YK, Rogers JA. Advanced materials and devices for bioresorbable electronics. Acc Chem Res. 2018;51(5):988-998. doi: 10.1021/acs.accounts.7b00548
- Yu KJ, Kuzum D, Hwang SW, et al. Bioresorbable silicon electronics for transient spatiotemporal mapping of electrical activity from the cerebral cortex. Nat Mater. 2016;15(7):782-791. doi: 10.1038/nmat4624
- Huang X. Materials and applications of bioresorbable electronics. J Semicond. 2018;39(1):011003. doi: 10.1088/1674-4926/39/1/011003
- Cao Q, Hur SH, Zhu ZT, et al. Highly bendable, transparent thin-film transistors that use carbon-nanotube-based conductors and semiconductors with elastomeric dielectrics. Adv Mater. 2006;18(3):304-309. doi: 10.1002/adma.200501740
- Wu X, Ma Y, Zhang G, et al. Thermally stable, biocompatible, and flexible organic field effect transistors and their application in temperature sensing arrays for artificial skin. Adv Funct Mater. 2015;25(14):2138-2146. doi: 10.1002/adfm.201404535
- Li X, Shi W, Yu X, Yu J. Performance improvement of organic field-effect transistor based nitrogen dioxide gas sensor using biocompatible PMMA/Silk fibroin bilayer dielectric. J Mater Sci Mater Electron. 2015;26(10):7948-7954. doi: 10.1007/s10854-015-3448-7
- Tan MJ, Owh C, Chee PL, Kyaw AKK, Kai D, Loh XJ. Biodegradable electronics: Cornerstone for sustainable electronics and transient applications. J Mater Chem C. 2016;4:5531-5558. doi: 10.1039/c6tc00678g
- Rullyani C, Sung CF, Lin HC, Chu CW. Flexible organic thin film transistors incorporating a biodegradable CO2-based polymer as the substrate and dielectric material. Sci Rep. 2018;8(1):8146. doi: 10.1038/s41598-018-26585-0
- Wang X, Ochiai S, Sawa G, et al. Organic field-effect transistors with crosslinkable polyvinyl alcohol insulator and spin-coated/drop-cast poly (3-Hexylthiophene-2,5-Diyl) semiconductor. Jpn J Appl Phys. 2007;46(3B):1337-1342. doi: 10.1143/JJAP.46.1337
- Benvenho ARV, Machado WS, Cruz-Cruz I, Hümmelgen IA. Study of poly(3-Hexylthiophene)/cross-linked poly(Vinyl Alcohol) as semiconductor/insulator for application in low voltage organic field effect transistors. J Appl Phys. 2013;113(21):214509. doi: 10.1063/1.4809285
- Feng L, Tang W, Zhao J, Cui Q, Jiang C, Guo X. All-solution-processed low-voltage organic thin-film transistor inverter on plastic substrate. IEEE Trans Electron Devices. 2014;61(4):1175-1180. doi: 10.1109/TED.2014.2303992
- Nawaz A, Cruz-Cruz I, Rodrigues R, Hümmelgen IA. Performance enhancement of poly(3-Hexylthiophene- 2,5-Diyl) based field effect transistors through surfactant treatment of the poly(Vinyl Alcohol) gate insulator surface. Phys Chem Chem Phys. 2014;17(40):26530-26534. doi: 10.1039/c4cp02245a
- Jastrombek D, Nawaz A, Koehler M, Meruvia MS, Hümmelgen IA. Modification of the charge transport properties of the copper phthalocyanine/poly(Vinyl Alcohol) interface using cationic or anionic surfactant for field-effect transistor performance enhancement. J Phys D Appl Phys. 2015;48(33):335104. doi: 10.1088/0022-3727/48/33/335104
- Irimia-Vladu M, Głowacki ED, Voss G, Bauer S, Sariciftci NS. Green and biodegradable electronics. Mater Today. 2012;15:340-346. doi: 10.1016/S1369-7021(12)70139-6
- Kim DH, Viventi J, Amsden JJ, et al. Dissolvable films of silk fibroin for ultrathin conformal bio-integrated electronics. Nat Mater. 2010;9(6):511-517. doi: 10.1038/nmat2745
- Ko J, Nguyen LTH, Surendran A, Tan BY, Ng KW, Leong WL. Human hair keratin for biocompatible flexible and transient electronic devices. ACS Appl Mater Interfaces. 2017;9(49):43004-43012. doi: 10.1021/acsami.7b16330
- Hemstreet JM. Dielectric constant of cotton. J Electrostat. 1982;13:345-353. doi: 10.1016/0304-3886(82)90052-3
- Jayamani E, Hamdan S, Rahman MR, Bin Bakri MK. Comparative study of dielectric properties of hybrid natural fiber composites. Procedia Eng. 2014;97:536-544. doi: 10.1016/j.proeng.2014.12.280
- Nawaz A, Liu Q, Leong WL, Fairfull-Smith KE, Sonar P. Organic electrochemical transistors for in vivo bioelectronics. Adv Mater. 2021;33(49):2170387. doi: 10.1002/adma.202170387
- Tarabella G, Mahvash Mohammadi F, Coppedè N, et al. New opportunities for organic electronics and bioelectronics: Ions in action. Chem Sci. 2013;4(4):1395-1409. doi: 10.1039/c2sc21740f
- Huang J, Gao Y, Chang Y, Peng J, Yu Y, Wang B. Machine learning in bioelectrocatalysis. Adv Sci (Weinh). 2024;11(2):e2306583. doi: 10.1002/advs.202306583
- Gu GH, Noh J, Kim I, Jung Y. Machine learning for renewable energy materials. J Mater Chem A. 2019;7(29):17096-17117. doi: 10.1039/C9TA02356A
- Ahmed M, Seraj R, Islam SMS. The K-means algorithm: A comprehensive survey and performance evaluation. Electronics (Basel). 2020;9(8):1295. doi: 10.3390/electronics9081295
- Chen A, Zhang X, Zhou Z. Machine learning: Accelerating materials development for energy storage and conversion. InfoMat. 2020;2(3):553-576. doi: 10.1002/inf2.12094
- Zhang W, Huang W, Tan J, Guo Q, Wu B. Heterogeneous catalysis mediated by light, electricity and enzyme via machine learning: Paradigms, applications and prospects. Chemosphere. 2022;308:136447. doi: 10.1016/j.chemosphere.2022.136447
- Cheng F, Ikenaga Y, Zhou Y, et al. In silico assessment of chemical biodegradability. J Chem Inf Model. 2012;52(3):655-669. doi: 10.1021/ci200622d
- Breiman L, Friedman JH, Olshen RA, Stone CJ. Classification and Regression Trees. United Kingdom: Routledge; 2017. doi: 10.1201/9781315139470
- Elsayad AM, Zeghid M, Ahmed HY, Elsayad KA. Exploration of biodegradable substances using machine learning techniques. Sustainability. 2023;15(17):12764. doi: 10.3390/su151712764
- Lunghini F, Marcou G, Gantzer P, et al. Modelling of ready biodegradability based on combined public and industrial data sources. SAR QSAR Environ Res. 2020;31(3):171-186. doi: 10.1080/1062936X.2019.1697360
- Czermiński R, Yasri A, Hartsough D. Use of support vector machine in pattern classification: Application to QSAR studies. Quant Struct Act Relat. 2001;20(3):227-240. doi: 10.1002/1521-3838(200110)20:3<227:AID-QSAR227> 3.0.CO;2-Y
- Davis CW, Camenzuli L, Redman AD. Predicting primary biodegradation of petroleum hydrocarbons in aquatic systems: Integrating system and molecular structure parameters using a novel machine-learning framework. Environ Toxicol Chem. 2022;41(6):1359-1369. doi: 10.1002/etc.5328
- Taunk K, De S, Verma S, Swetapadma A. A Brief Review of Nearest Neighbor Algorithm for Learning and Classification. In: 2019 International Conference on Intelligent Computing and Control Systems (ICCS). IEEE; 2019. p. 1255-1260. doi: 10.1109/ICCS45141.2019.9065747
- Zhang XM, Liang L, Liu L, Tang MJ. Graph neural networks and their current applications in bioinformatics. Front Genet. 2021;12:690049. doi: 10.3389/fgene.2021.690049
- Frazier PI. A Tutorial on Bayesian Optimization, Section 5. 2018. p. 1-22. Available from: http://arxiv.org/ abs/1807.02811 [Last accessed on 2024 Jul 02].
- De Carvalho Rocha WF, Sheen DA. Classification of biodegradable materials using QSAR modeling with uncertainty estimation. SAR QSAR Environ Res. 2016;27(10):799-811. doi: 10.1080/1062936X.2016.1238010
- Nolte TM, Peijnenburg WJGM, van Bergen TJHM, Hendriks AJ. Transition-state rate theory sheds light on “Black-Box” biodegradation algorithms. Green Chem. 2020;22(11):3558-3571. doi: 10.1039/D0GC00337A
- McDonald SM, Augustine EK, Lanners Q, Rudin C, Catherine Brinson L, Becker ML. Applied machine learning as a driver for polymeric biomaterials design. Nat Commun. 2023;14(1):4838. doi: 10.1038/s41467-023-40459-8
- Jaworska JS, Boethling RS, Howard PH. Recent developments in broadly applicable structure-biodegradability relationships. Environ Toxicol Chem. 2003;22(8):1710-1723. doi: 10.1897/01-302
- Huang K, Zhang H. Classification and regression machine learning models for predicting aerobic ready and inherent biodegradation of organic chemicals in water. Environ Sci Technol. 2022;56(17):12755-12764. doi: 10.1021/acs.est.2c01764
- Lee M, Min KA. comparative study of the performance for predicting biodegradability classification: The quantitative structure-activity relationship model vs the graph convolutional network. ACS Omega. 2022;7(4):3649-3655. doi: 10.1021/acsomega.1c06274
- Cencer MM, Moore JS, Assary RS. Machine learning for polymeric materials: An introduction. Polym Int. 2022;71(5):537-542. doi: 10.1002/pi.6345
- Lin A, Uva A, Babi J, Tran H. Materials design for resilience in the biointegration of electronics. MRS Bull. 2021;46:860- 869. doi: 10.1557/s43577-021-00174-5
- Jorgensen RA. Plant science. A window on the sophistication of plants. Science. 2011;333(6046):1103-1104. doi: 10.1126/science.1211194
- Roth B, Savagatrup S, De Los Santos NV, et al. Mechanical properties of a library of low-band-gap polymers. Chem Mater. 2016;28(7):2363-2373. doi: 10.1021/acs.chemmater.6b00525
- Mei J, Bao Z. Side chain engineering in solution-processable conjugated polymers. Chem Mater. 2014;26(11):604-615. doi: 10.1021/cm4020805
- Abetz V, Simon PFW. Phase behaviour and morphologies of block copolymers. In: Advances in Polymer Science. Berlin: Springer; 2005. p. 125-212. doi: 10.1007/12_004
- Sidky H, Chen W, Ferguson AL. Molecular latent space simulators. Chem Sci. 2020;11(35):9459-9467. doi: 10.1039/d0sc03635h
- Gu Y, Zhao J, Johnson JA. A (Macro)molecular-level understanding of polymer network topology. Trends Chem. 2019;1(3):318-334. doi: 10.1016/j.trechm.2019.02.017
- Rubinstein M. Polymer physics-the ugly duckling story: Will polymer physics ever become a part of “Proper” physics? J Polym Sci B Polym Phys. 2010;48:2548-2551. doi: 10.1002/polb.22135
- Göpferich A. Mechanisms of Polymer Degradation and Erosion. Vol. 17. Amsterdam: Elsevier; 1996. doi: 10.1016/B978-008045154-1.50016-2
- Wang R, Lin TS, Johnson JA, Olsen BD. Kinetic Monte Carlo simulation for quantification of the gel point of polymer networks. ACS Macro Lett. 2017;6(12):1414-1419. doi: 10.1021/acsmacrolett.7b00586
- Zhong M, Wang R, Kawamoto K, Olsen BD, Johnson JA. Quantifying the impact of molecular defects on polymer network elasticity. Science. 2016;353(6305):1264-1268. doi: 10.1126/science.aag0184
- Vieira AC, Marques AT, Guedes RM, Tita V. Material model proposal for biodegradable materials. Procedia Eng. 2011;10:1597-1602. doi: 10.1016/j.proeng.2011.04.267
- Jiang S, Liang Y, Shi S, Wu C, Shi Z. Improving predictions and understanding of primary and ultimate biodegradation rates with machine learning models. Sci Total Environ. 2023;904:166623. doi: 10.1016/j.scitotenv.2023.166623
- Wang HSH, Yao Y. Machine learning for sustainable development and applications of biomass and biomass-derived carbonaceous materials in water and agricultural systems: A review. Resour Conserv Recycl. 2023;190:106847. doi: 10.1016/j.resconrec.2022.106847
- Albright VC, Chai Y. Knowledge gaps in polymer biodegradation research. Environ Sci Technol. 2021;55:11476-11488. doi: 10.1021/acs.est.1c00994
- Wilson AN, St John PC, Marin DH, et al. PolyID: Artificial intelligence for discovering performance-advantaged and sustainable polymers. Macromolecules. 2023;56(21):8547-8557. doi: 10.1021/acs.macromol.3c00994
- Roch LM, Häse F, Kreisbeck C, et al. ChemOS: Orchestrating autonomous experimentation. Sci Robot. 2018;3(19):eaat5559. doi: 10.1126/scirobotics.aat5559
- Baum ZJ, Yu X, Ayala PY, Zhao Y, Watkins SP, Zhou Q. Artificial intelligence in chemistry: Current trends and future directions. J Chem Inf Model. 2021;36(9):3197-3212. doi: 10.1021/acs.jcim.1c00619
- Kamkar M, Leonard KC, Ferrer I, et al. Artificial intelligence (AI) for sustainable resource management and chemical processes. ACS Sustain Chem Eng. 2024;12(8):2924-2926. doi: 10.1021/acssuschemeng.4c01004
- Kostal J. Making the case for quantum mechanics in predictive toxicology-nearly 100 years too late? Chem Res Toxicol. 2023;36(9):1444-1450. doi: 10.1021/acs.chemrestox.3c00171
- For chemists, the AI revolution has yet to happen. Nature. 2023;617(7961):438. doi: 10.1038/d41586-023-01612-x
- Open Data and AI: A Symbiotic Relationship for Progress. Available from: https://data.europa.eu/en/publications/ datastories/open-data-and-ai-symbiotic-relationship-progress [Last accessed on 2024 Mar 07].
- Gormley AJ, Webb MA. Machine learning in combinatorial polymer chemistry. Nat Rev Mater. 2021;6(8):642-644. doi: 10.1038/s41578-021-00282-3
- Hong S, Liow CH, Yuk JM, et al. Reducing time to discovery: Materials and molecular modeling, imaging, informatics, and integration. ACS Nano. 2021;15(3):3971-3995. doi: 10.1021/acsnano.1c00211
- Chen G, Shen Z, Iyer A, et al. Machine-learning-assisted de novo design of organic molecules and polymers: Opportunities and challenges. Polymers (Basel). 2020;12(1):163. doi: 10.3390/polym12010163
- Goh GD, Lee JM, Goh GL, Huang X, Lee S, Yeong WY. Machine learning for bioelectronics on wearable and implantable devices: Challenges and potential. Tissue Eng Part A. 2023;29(1-2):20-46. doi: 10.1089/ten.tea.2022.0119