Application of machine learning in 3D bioprinting of cultivated meat
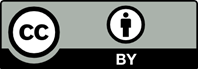
Cultivated meat production, an innovative and sustainable alternative to conventional animal farming, has gained significant attention in recent years. As the demand for ethical and environmentally friendly protein sources continues to rise, the need for efficient and scalable production strategies becomes critical. Notably, the integration of advanced technology, such as machine learning (ML), can enhance the efficiency of the cultivated meat production process. The goal of this review paper is to highlight the advantages and limitations of various ML approaches and provide a balanced discussion on the integration of ML techniques for three-dimensional (3D)-bioprinted cultivated meat. This review paper explores the application of ML techniques in various facets of 3D-bioprinted cultivated meat and highlights the potential for ML to optimize various aspects of the process, from predicting printability and optimizing printing parameters to characterizing meat flavor and monitoring meat quality. ML plays a pivotal role in optimizing the material formulation to improve ink printability and identifying an optimal combination of printing parameters to achieve high printing resolution and accuracy. Furthermore, ML can aid in modeling sensory attributes, ensuring that the cultivated meat replicates the desired meat flavor. Finally, ML can be applied for meat quality control as it facilitates the automated detection of harmful pathogens, ensuring the safety and consistency of 3D-bioprinted cultivated meat.
- Post MJ, Levenberg S, Kaplan DL, et al. Scientific, sustainability and regulatory challenges of cultivated meat. Nat Food. 2020;1(7):403-415. doi: 10.1038/s43016-020-0112-z
- Tuomisto HL, de Mattos M.J.T. Environmental impacts of cultivated meat production. Environ Sci Technol. 2011;45(14):6117-6123. doi: 10.1021/es200130u
- Mantihal S, Kobun, R, Lee BB. 3D food printing of as the new way of preparing food: A review. Int J Gastron Food Sci. 2020;22:100260. doi: 10.1016/j.ijgfs.2020.100260
- Ng WL, Chua CK, Shen YF. Print me an organ! Why we are not there yet, prog. Polym Sci. 2019;97:101145. doi: 10.1016/j.progpolymsci.2019.101145
- Santoni S, Gugliandolo SG, Sponchioni M, Moscatelli D, Colosimo BM. 3D bioprinting: Current status and trends-a guide to the literature and industrial practice. Bio-Des Manuf. 2022;5(1):14-42. doi: 10.1007/s42242-021-00165-0
- Ng WL, Tan ZQ, Yeong WY, Naing MW. Proof-of-concept: 3D bioprinting of pigmented human skin constructs. Biofabrication. 2018;10(2):025005. doi: 10.1088/1758-5090/aa9e1e
- Ng WL, Ayi TC, Liu YC, Sing SL, Yeong WY, Tan BH. Fabrication and characterization of 3D bioprinted triple-layered human alveolar lung models. Int J Bioprint. 2021;7(2):332. doi: 10.18063/ijb.v7i2.332
- Ng WL, Lee JM, Zhou M, Yeong WY. Hydrogels for 3-D bioprinting-based tissue engineering. In: Narayan R, editor. Rapid Prototyping of Biomaterials. Chapel Hill, NC, Elsevier, 2020, p183-204.
- Ng WL, Huang X, Shkolnikov V, Goh GL, Suntornnond R, Yeong WY. Controlling droplet impact velocity and droplet volume: Key factors to achieving high cell viability in sub-nanoliter droplet-based bioprinting. Int J Bioprint. 2022;8(1):424. doi: 10.18063/ijb.v8i1.424
- Ng WL, Goh MH, Yeong WY, Naing MW. Applying macromolecular crowding to 3D bioprinting: Fabrication of 3D hierarchical porous collagen-based hydrogel constructs. Biomater Sci. 2018;6(3):562-574. doi: 10.1039/C7BM01015J
- Ozbolat IT, Hospodiuk M. Current advances and future perspectives in extrusion-based bioprinting. Biomaterials. 2016;76:321-343. doi: 10.1016/j.biomaterials.2015.10.076
- Ng WL, Huang, X, Shkolnikov V, Suntornnond R, Yeong WY. Polyvinylpyrrolidone-based bioink: Influence of bioink properties on printing performance and cell proliferation during inkjet-based bioprinting. Bio-Des Manuf. 2023;6(2):676-690. doi: 10.1007/s42242-023-00245-3
- Ng WL, Lee JM, Yeong WY, Naing MW. Microvalve-based bioprinting-process, bio-inks and applications. Biomater Sci. 2017;5(4):632-647. doi: 10.1039/C6BM00861E
- Ng WL, Lee JM, Zhou M. Vat polymerization-based bioprinting-process, materials, applications and regulatory challenges. Biofabrication. 2020;12(2):022001. doi: 10.1088/1758-5090/ab6034
- Zhaoxuan F, Jinsong L, Dasen Z, Hui S, Jiaqi L, Wenqin B. A novel photocurable pullulan-based bioink for digital light processing 3D printing. Int J Bioprint. 2023;9(2):657. doi: 10.18063/ijb.v9i2.657
- Armstrong AA, Alleyne AG, Johnson AJW. 1D and 2D error assessment and correction for extrusion-based bioprinting using process sensing and control strategies. Biofabrication. 2020;12(4):045023. doi: 10.1088/1758-5090/aba8ee
- Wojnowski W, Majchrzak T, Dymerski T, Gębicki J, Namieśnik J. Electronic noses: Powerful tools in meat quality assessment. Meat Sci. 2017;131:119-131. doi: 10.1016/j.meatsci.2017.04.240
- Pereira PFM, de Sousa Picciani PH, Calado V, Tonon RV. Electrical gas sensors for meat freshness assessment and quality monitoring: A review. Trends Food Sci Technol. 2021;118:36-44. doi: 10.1016/j.tifs.2021.08.036
- Goh GD, Sing SL, Yeong WY. A review on machine learning in 3D printing: Applications, potential, and challenges. Artif Intell Rev. 2021;54(1):63-94. doi: 10.1007/s10462-020-09876-9
- Ng WL, Chan A, Ong YS, Chua CK. Deep learning for fabrication and maturation of 3D bioprinted tissues and organs. Virtual Phys Prototyp. 2020;15(3):340-358. doi: 10.1080/17452759.2020.1771741
- Huang X, Ng WL, Yeong WY. Predicting the number of printed cells during inkjet-based bioprinting process based on droplet velocity profile using machine learning approaches. J Intell Manuf. 2023. doi: 10.1007/s10845-023-02167-4
- Caruana R, Niculescu-Mizil A. An Empirical Comparison of Supervised Learning Algorithms. ICML: Proceedings of the 23rd International Conference on Machine Learning. Pittsburgh, 2006, p161-168. doi: 10.1145/1143844.1143865
- Hastie T, Tibshirani R, Friedman J. Unsupervised learning. In: Hastie T, Tibshirani R, Friedman J, editors. The Elements of Statistical Learning: Data Mining, Inference, and Prediction. New York, Springer, 2009, p485-585.
- Van Engelen JE, Hoos HH. A survey on semi-supervised learning. Mach Learn. 2020;109(2):373-440. doi: 10.1007/s10994-019-05855-6
- Arulkumaran K, Deisenroth MP, Brundage M, Bharath AA. Deep reinforcement learning: A brief survey. IEEE Signal Process Mag. 2017;34(6):26-38. doi: 10.1109/MSP.2017.2743240
- Plathottam SJ, Rzonca A, Lakhnori R, Iloeje CO. A review of artificial intelligence applications in manufacturing operations. J Adv Manuf Process. 2023;5(7):e10159. doi: 10.1002/amp2.10159
- Xu E, Niu, R, Lao J, et al. Tissue-like cultivated fish fillets through a synthetic food pipeline. NPJ Sci Food. 2023;7(1):17. doi: 10.1038/s41538-023-00194-2
- Dutta SD, Ganguly K, Jeong MS, et al. Bioengineered lab-grown meat-like constructs through 3D bioprinting of antioxidative protein hydrolysates. ACS Appl Mater Interfaces. 2022;14(30):34513-34526. doi: 10.1021/acsami.2c10620
- Ianovici I, Zagury Y, Redenski I, Lavon N, Levenberg S. 3D-printable plant protein-enriched scaffolds for cultivated meat development. Biomaterials. 2022;284:121487. doi: 10.1016/j.biomaterials.2022.121487
- Kang DH, Louis F, Liu H, et al. Engineered whole cut meat-like tissue by the assembly of cell fibers using tendon-gel integrated bioprinting. Nat Commun. 2021;12(1):5059. doi: 10.1038/s41467-021-25236-9
- Sealy MP, Avegnon KLM, Garrett A, Delbreilh L, Bapat S, Malshe AP. Understanding biomanufacturing of soy-based scaffolds for cell-cultivated meat by vat polymerization. CIRP Ann. 2022;71(1):209-212. doi: 10.1016/j.cirp.2022.04.001
- Li Y, Liu W, Li S, Zhang M, Yang F, Wang S. Porcine skeletal muscle tissue fabrication for cultivated meat production using three-dimensional bioprinting technology. J Future Foods. 2021;1(1):88-97. doi: 10.1016/j.jfutfo.2021.09.005
- Zhuang P, Ng WL, An J, Chua CK, Tan LP. Layer-by-layer ultraviolet assisted extrusion-based (UAE) bioprinting of hydrogel constructs with high aspect ratio for soft tissue engineering applications. PLoS One. 2019;14(6):e0216776. doi: 10.1371/journal.pone.0216776
- Ng WL, Yeong WY, Naing MW. Microvalve Bioprinting of Cellular Droplets with High Resolution and Consistency. In: Proceedings of the International Conference on Progress in Additive Manufacturing, 2016, p397-402. doi: 10.3850/2424-8967_V02-236
- Lee JM, Suen SKQ, Ng WL, Ma WC, Yeong WY. Bioprinting of collagen: Considerations, potentials, and applications. Macromol Biosci. 2020;21(1):2000280. doi: 10.1002/mabi.202000280
- Lee JM, Ng WL, Yeong WY. Resolution and shape in bioprinting: Strategizing towards complex tissue and organ printing. Appl Phys Rev. 2019;6(1):011307. doi: 10.1063/1.5053909
- Ng WL, Yeong WY, Naing MW. Development of polyelectrolyte chitosan-gelatin hydrogels for skin bioprinting. Procedia CIRP. 2016;49:105-112. doi: 10.1016/j.procir.2015.09.002
- Ng WL, Yeong WY, Naing MW. Polyelectrolyte gelatin-chitosan hydrogel optimized for 3D bioprinting in skin tissue engineering. Int J Bioprint. 2016;2(1):53-62. doi: 10.18063/IJB.2016.01.009
- Suntornnond R, Ng WL, Huang X, Yeow CHE, Yeong WY. Improving printability of hydrogel-based bio-inks for thermal inkjet bioprinting applications via saponification and heat treatment process. J Mater Chem B. 2022;10(31):5989-6000. doi: 10.1039/D2TB00442A
- Ng WL, Yeong WY, Naing MW. Polyvinylpyrrolidone-based bio-ink improves cell viability and homogeneity during drop-on-demand printing. Materials (Basel). 2017;10(2):190. doi: 10.3390/ma10020190
- Bonatti AF, Vozzi G, Chua CK, Maria C. A deep learning quality control loop of the extrusion-based bioprinting process. Int J Bioprint. 2022;8(4):620. doi: 10.18063/ijb.v8i4.620
- Lu Y, Rai R, Nitin N. Image-based assessment and machine learning-enabled prediction of printability of polysaccharides-based food ink for 3D printing. Food Res Int. 2023;173(Pt 2):113384. doi: 10.1016/j.foodres.2023.113384
- Chen H, Liu Y, Balabani S, Hirayama R, Huang J. Machine learning in predicting printable biomaterial formulations for direct ink writing. Research (Wash D C). 2023;6:0197. doi: 10.34133/research.0197
- Oh D, Shirzad M, Kim MC, Chung EJ, Nam SY. Rheology-informed hierarchical machine learning model for the prediction of printing resolution in extrusion-based bioprinting. Int J Bioprint. 2023;9(6):1280. doi: 10.36922/ijb.1280
- Fu Z, Angeline V, Sun W. Evaluation of printing parameters on 3D extrusion printing of pluronic hydrogels and machine learning guided parameter recommendation. Int J Bioprint. 2021;7(4):434. doi: 10.18063/ijb.v7i4.434
- Lavin JG, Lawless HT. Effects of color and odor on judgments of sweetness among children and adults. Food Qual Prefer. 1998;9(4):283-289. doi: 10.1016/S0950-3293(98)00009-3
- Wang G, Hayes JE, Ziegler GR, Roberts RF, Hopfer H. Dose-response relationships for vanilla flavor and sucrose in skim milk: Evidence of synergy. Beverages. 2018;4(4):73. doi: 10.3390/beverages4040073
- Cometto-Muñiz JE. Odor, taste, and flavor perception of some flavoring agents. Chem Senses. 1981;6(3):215-223. doi: 10.1093/chemse/6.3.215
- Prescott J. Flavour as a psychological construct: Implications for perceiving and measuring the sensory qualities of foods. Food Qual Prefer. 1999;10(4-5):349-356. doi: 10.1016/S0950-3293(98)00048-2
- Moskowitz HR, Arabie P. Taste intensity as a function of stimulus concentration and solvent viscosity. J Texture Stud. 1970;1(4):502-510. doi: 10.1111/j.1745-4603.1970.tb00748.x
- Pangborn RM, Szczesniak AS. Effect of hydrocolloids and viscosity on flavor and odor intensities of aromatic flavor compounds. J Texture Stud. 1974;4(4):467-482. doi: 10.1111/j.1745-4603.1974.tb00857.x
- Ferry AL, Hort J, Mitchell J, Cook DJ, Lagarrigue S, Pamies BV. Viscosity and flavour perception: Why is starch different from hydrocolloids? Food Hydrocoll. 2006;20(6):855-862. doi: 10.1016/j.foodhyd.2005.08.008
- Pangborn RM, Gibbs ZM, Tassan C. Effect of hydrocolloids on apparent viscosity and sensory properties of selected beverages. J Texture Stud. 1978;9(4):415-436. doi: 10.1111/j.1745-4603.1978.tb01216.x
- Tournier C, Sulmont-Rossé C, Guichard E. Flavour perception: Aroma, taste and texture interactions. Food. 2007;1(2):246-257.
- Lawless HT, Heymann H. Sensory Evaluation of Food: Principles and Practices. Berlin, Springer, 2010.
- Chambers E 4th, Koppel K. Associations of volatile compounds with sensory aroma and flavor: The complex nature of flavor. Molecules. 2013;18(5):4887-4905. doi: 10.3390/molecules18054887
- Song S, Zhang X, Hayat K, et al. Contribution of beef base to aroma characteristics of beeflike process flavour assessed by descriptive sensory analysis and gas chromatography olfactometry and partial least squares regression. J Chromatogr A. 2010;1217(49):7788-7799. doi: 10.1016/j.chroma.2010.10.046
- Song S, Tang Q, Fan L, et al. Identification of pork flavour precursors from enzyme-treated lard using maillard model system assessed by GC-MS and partial least squares regression. Meat Sci. 2017;124:15-24. doi: 10.1016/j.meatsci.2016.10.009
- Li J, Xu Y, Du W, et al. Comparative analysis of aroma compounds in Chinese traditional dry-rendered fat by HS/ GC-IMS, SPME/GC-MS, and SPME/GC-O. J Food Compost Anal. 2022;107:104378. doi: 10.1016/j.jfca.2021.104378
- Cui J, Wang Y, Wang Q, et al. Prediction of flavor of maillard reaction product of beef tallow residue based on artificial neural network. Food Chem X. 2022;15:100447. doi: 10.1016/j.fochx.2022.100447
- Beattie RJ, Bell SJ, Farmer LJ, Moss BW, Patterson D. Preliminary investigation of the application of Raman spectroscopy to the prediction of the sensory quality of beef silverside. Meat Sci. 2004;66(4):903-913. doi: 10.1016/j.meatsci.2003.08.012
- Zhao M, Nian Y, Allen P, Downey G, Kerry JP, O’Donnell CP. Application of Raman spectroscopy and chemometric techniques to assess sensory characteristics of young dairy bull beef. Food Res Int. 2018;107:27-40. doi: 10.1016/j.foodres.2018.02.007
- Pereira FMV, Bertelli Pflanzer S, Gomig T, Lugnani Gomes C, de Felício PE, Colnago LA. Fast determination of beef quality parameters with time-domain nuclear magnetic resonance spectroscopy and chemometrics. Talanta. 2013;108:88-91. doi: 10.1016/j.talanta.2013.02.070
- Brøndum J, Byrne DV, Bak LS, Bertelsen G, Engelsen SB. Warmed-over flavour in porcine meat-a combined spectroscopic, sensory and chemometric study. Meat Sci. 2000;54(1):83-95. doi: 10.1016/S0309-1740(99)00085-6
- Wang H, Wang XD, Liu D, Wang Y, Li X, Duan J. Evaluation of beef flavor attribute based on sensor array in tandem with support vector machines. J Food Meas Charact. 2019;13:2663-2671. doi: 10.1007/s11694-019-00187-4
- Jiang S, Zhu Y, Peng J, Zhang Y, Zhang W, Liu Y. Characterization of stewed beef by sensory evaluation and multiple intelligent sensory technologies combined with chemometrics methods. Food Chem. 2023;408:135193. doi: 10.1016/j.foodchem.2022.135193
- Tournas V, Stack ME, Mislivec PB, Koch HA, Bandler R. BAM Chapter 18: Yeasts, Molds and Mycotoxins, 2001. https://www.fda.gov/food/laboratory-methods-food/bam-chapter-18-yeasts-molds-and-mycotoxins. [Last accessed on 2023 Nov 14].
- World Health Organization. Microbiological Risk Assessment- Guidance for food. Rome, Food and Agriculture Org., 2021.
- Soni A, Dixit Y, Reis MM, Brightwell G. Hyperspectral imaging and machine learning in food microbiology: Developments and challenges in detection of bacterial, fungal, and viral contaminants. Compr Rev Food Sci Food Saf. 2022;21(4):3717-3745. doi: 10.1111/1541-4337.12983
- Uysal RS, Boyaci IH, Genis HE, Tamer U. Determination of butter adulteration with margarine using Raman spectroscopy. Food Chem. 2013;141(4):4397-4403. doi: 10.1016/j.foodchem.2013.06.061
- Hu S, Li H, Chen C, et al. Raman spectroscopy combined with machine learning algorithms to detect adulterated Suichang native honey. Sci Rep. 2022;12(1):3456. doi: 10.1038/s41598-022-07222-3
- Tao F, Yao H, Hruska Z, Rajasekaran K, Qin J, Kim M. Use of line-scan Raman hyperspectral imaging to identify corn kernels infected with Aspergillus flavus. J Cereal Sci. 2021;102:103364. doi: 10.1016/j.jcs.2021.103364
- Yan S, Wang S, Qiu J, et al. Raman spectroscopy combined with machine learning for rapid detection of food-borne pathogens at the single-cell level. Talanta. 2021;226:122195. doi: 10.1016/j.talanta.2021.122195
- Zheng X, Peng Y, Wang W. A nondestructive real-time detection method of total viable count in pork by hyperspectral imaging technique. Appl Sci. 2017;7(3):213. doi: 10.3390/app7030213
- Argyri AA, Panagou EZ, Tarantilis P, Polysiou M, Nychas GJE. Rapid qualitative and quantitative detection of beef fillets spoilage based on Fourier transform infrared spectroscopy data and artificial neural networks. Sens Actuators B Chem. 2010;145(1):146-154. doi: 10.1016/j.snb.2009.11.052
- Enériz D, Medrano N, Calvo B. An FPGA-based machine learning tool for in-situ food quality tracking using sensor fusion. Biosensors. 2021;11(10):366. doi: 10.3390/bios11100366
- Yang H, Hopkins DL, Zhang Y, et al. Preliminary investigation of the use of Raman spectroscopy to predict beef spoilage in different types of packaging. Meat Sci. 2020;165:108136. doi: 10.1016/j.meatsci.2020.108136
- Panagou EZ, Papadopoulou O, Carstensen JM, Nychas GJ. Potential of multispectral imaging technology for rapid and non-destructive determination of the microbiological quality of beef filets during aerobic storage. Int J Food Microbiol. 2014;174:1-11. doi: 10.1016/j.ijfoodmicro.2013.12.026
- Tsakanikas P, Pavlidis D, Panagou E, Nychas GJ. Exploiting multispectral imaging for non-invasive contamination assessment and mapping of meat samples. Talanta. 2016;161:606-614. doi: 10.1016/j.talanta.2016.09.019
- Yang D, He D, Lu A, Ren D, Wang J. Detection of the freshness state of cooked beef during storage using hyperspectral imaging. Appl Spectrosc. 2017;71(10):2286-2301. doi: 10.1177/0003702817718807
- Huang L, Zhao J, Chen Q, Zhang Y. Rapid detection of total viable count (TVC) in pork meat by hyperspectral imaging. Food Res Int. 2013;54(1):821-828. doi: 10.1016/j.foodres.2013.08.011
- Papadopoulou O, Panagou EZ, Tassou, CC, Nychas GJE. Contribution of Fourier transform infrared (FTIR) spectroscopy data on the quantitative determination of minced pork meat spoilage. Food Res Int. 2011;44(10):3264-3271. doi: 10.1016/j.foodres.2011.09.012
- Wijaya DR, Sarno R, Zulaika E. DWTLSTM for electronic nose signal processing in beef quality monitoring. Sens Actuators B Chem. 2021;326:128931. doi: 10.1016/j.snb.2020.128931
- Li YC, Liu SY, Meng FB, et al. Comparative review and the recent progress in detection technologies of meat product adulteration. Compr Rev Food Sci Food Saf. 2020;19(4):2256-2296. doi: 10.1111/1541-4337.12579
- Fan B, Zhu R, He D, Wang S, Cui X, Yao X. Evaluation of mutton adulteration under the effect of mutton flavour essence using hyperspectral imaging combined with machine learning and sparrow search algorithm. Foods. 2022;11(15):2278. doi: 10.3390/foods11152278
- Nunes KM, Andrade MVO, Santos Filho AMP, Lasmar MC, Sena MM. Detection and characterisation of frauds in bovine meat in natura by non-meat ingredient additions using data fusion of chemical parameters and ATR-FTIR spectroscopy. Food Chem. 2016;205:14-22. doi: 10.1016/j.foodchem.2016.02.158
- Wakhid S, Sarno R, Sabilla SI. The effect of gas concentration on detection and classification of beef and pork mixtures using E-nose. Comput Electron Agric. 2022;195:106838. doi: 10.1016/j.compag.2022.106838
- Alamprese C, Casale M, Sinelli N, Lanteri S, Casiraghi E. Detection of minced beef adulteration with turkey meat by UV-vis, NIR and MIR spectroscopy. LWT Food Sci Technol. 2013;53(1):225-232. doi: 10.1016/j.lwt.2013.01.027
- Meza-Márquez OG, Gallardo-Velázquez T, Osorio- Revilla G. Application of mid-infrared spectroscopy with multivariate analysis and soft independent modeling of class analogies (SIMCA) for the detection of adulterants in minced beef. Meat Sci. 2010;86(2):511-519. doi: 10.1016/j.meatsci.2010.05.044
- Rohman A, Sismindari, Erwanto Y, Man YBC. Analysis of pork adulteration in beef meatball using Fourier transform infrared (FTIR) spectroscopy. Meat Sci. 2011;88(1):91-95. doi: 10.1016/j.meatsci.2010.12.007
- Guntarti A, Martono S, Yuswanto A, Rohman A. FTIR spectroscopy in combination with chemometrics for analysis of wild boar meat in meatball formulation. Asian J Biochem. 2015;10(4):165-172. doi: 10.3923/ajb.2015.165.172