Integration of physics-based data in deep learning model training for predicting the effect of sulfur content in the directed energy deposition process
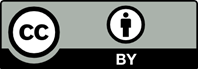
The training of a machine learning model solely on experimental data, encompassing both pre- and post-process information, can reveal the general relationship of the directed energy deposition process. However, models trained in this manner encounter limitations in capturing critical in-process information occurring during deposition. This paper details the training of a deep learning model through the integration of in-process physics-based simulation information and a pre-process experiment dataset. The sulfur content of stainless steel 316L was selected as critical in-process information affecting the final track geometry and was captured using computational fluid dynamics simulation of a single-track deposition process, which cannot be captured accurately through experimentation. The physics-based simulation dataset was generated by obtaining the contour of deposition and dilution of the solidified track cross-section. The experiment was conducted using central composite design, and data augmentation was achieved through curve fitting using a response surface methodology regression model. Statistical analysis assessing the quality of simulation and experiment data was conducted. Among six baseline models, a deep learning model with a specified training sequence of experiment and simulation data, denoted as DL-AugExp-Sim-Exp, exhibited the best-performing R2 and root mean square error prediction accuracy for cross-section track shape. Notably, deep learning models trained with both experiment and simulation information demonstrated a lower R2 value compared to models trained solely with experiment data, revealing a tradeoff between R2 value and additional prediction capability. In summary, in this study, the integration of a physics-based simulation dataset demonstrated the additional prediction capability concerning the effect of sulfur content on track geometry.
- Tsotridis G, Rother H, Hondros ED. Marangoni flow and the shapes of laser-melted pool. Sci Nat. 1989;76(5):216-218. doi: 10.1007/BF00627691
- Zhang YM, Lim CWJ, Tang C, Li B. Numerical investigation on heat transfer of melt pool and clad generation in directed energy deposition of stainless steel. Int J Therm Sci. 2021;165:106954. doi:10.1016/j.ijthermalsci.2021.106954
- Guan X, Zhao YF. Modeling of the laser powder-based directed energy deposition process for additive manufacturing: A review. Int J Adv Manuf Technol. 2020;107(5-6):1959-1982. doi: 10.1007/s00170-020-05027-0
- Yao L, Xiao Z, Huang S, Ramamurty U. The formation mechanism of metal-ceramic interlayer interface during laser powder bed fusion. Virtual Phys Prototyp. 2023;18(1):e2235324. doi: 10.1080/17452759.2023.2235324
- Yao L, Huang S, Ramamurty U, Xiao Z. On the formation of ‘Fish-scale’ morphology with curved grain interfacial microstructures during selective laser melting of dissimilar alloys. Acta Mater. 2021;220:117331. doi: 10.1016/j.actamat.2021.117331
- Gan Z, Yu G, He X, Li S. Surface-active element transport and its effect on liquid metal flow in laser-assisted additive manufacturing. Int Commun Heat Mass Transf. 2017;86:206-214. doi: 10.1016/j.icheatmasstransfer.2017.06.007
- “Grace” Guo W, Tian Q, Guo S, Guo Y. A physics-driven deep learning model for process-porosity causal relationship and porosity prediction with interpretability in laser metal deposition. CIRP Ann. 2020;69(1):205-208. doi: 10.1016/j.cirp.2020.04.049
- Wei HL, Mukherjee T, Zhang, et al. Mechanistic models for additive manufacturing of metallic components. Prog Mater Sci. 2021;116:100703. doi: 10.1016/j.pmatsci.2020.100703
- Goh GD, Huang X, Huang S, Thong JLJ, Seah JJ, Yeong WY. Data imputation strategies for process optimization of laser powder bed fusion of Ti6Al4V using machine learning. Mater Sci Addit Manuf. 2023;2(1):50. doi: 10.36922/msam.50
- Wang C, Tan XP, Tor SB, Lim CS. Machine learning in additive manufacturing: State-of-the-art and perspectives. Addit Manuf. 2020;36:101538. doi: 10.1016/j.addma.2020.101538
- Feenstra DR, Molotnikov A, Birbilis N. Utilisation of artificial neural networks to rationalise processing windows in directed energy deposition applications. Mater Des. 2021;198:109342. doi: 10.1016/j.matdes.2020.109342
- Li J, Sage M, Guan X, Brochu M, Zhao YF. Machine learning-enabled competitive grain growth behavior study in directed energy deposition fabricated Ti6Al4V. JOM. 2020;72(1):458-464. doi: 10.1007/s11837-019-03917-7
- Han Y, Griffiths RJ, Yu HZ, Zhu Y. Quantitative microstructure analysis for solid-state metal additive manufacturing via deep learning. J Mater Res. 2020;35(15):1936-1948. doi: 10.1557/jmr.2020.120
- Gayon-Lombardo A, Mosser L, Brandon NP, Cooper SJ. Pores for thought: Generative adversarial networks for stochastic reconstruction of 3D multi-phase electrode microstructures with periodic boundaries. npj Comput Mater. 2020;6(1):82. doi: 10.1038/s41524-020-0340-7
- Huang S, Kumar P, Lim CWJ, Radhakrishnan J, Ramamurty U. Fracture behavior of PH15-5 stainless steel manufactured via directed energy deposition. Mater Des. 2023;235:112421. doi: 10.1016/j.matdes.2023.112421
- Khanzadeh M, Chowdhury S, Marufuzzaman M, Tschopp MA, Bian L. Porosity prediction: Supervised-learning of thermal history for direct laser deposition. J Manuf Syst. 2018;47:69-82. doi: 10.1016/j.jmsy.2018.04.001
- Khanzadeh M, Chowdhury S, Tschopp MA, Doude HR, Marufuzzaman M, Bian L. In-situ monitoring of melt pool images for porosity prediction in directed energy deposition processes. IISE Trans. 2019;51(5):437-455. doi: 10.1080/24725854.2017.1417656
- Garcia-Moreno AI. Automatic quantification of porosity using an intelligent classifier. Int J Adv Manuf Technol. 2019;105(5-6):1883-1899. doi: 10.1007/s00170-019-04067-5
- Wang S, Zhu L, Fuh JYH, Zhang H, Yan W. Multi-physics modeling and Gaussian process regression analysis of cladding track geometry for direct energy deposition. Opt Lasers Eng. 2020;127:105950. doi: 10.1016/j.optlaseng.2019.105950
- Seifi SH, Tian W, Doude H, Tschopp MA, Bian L. Layer-wise modeling and anomaly detection for laser-based additive manufacturing. J Manuf Sci Eng ASME. 2019;141(8):81013. doi: 10.1115/1.4043898
- Lu ZL, Li DC, Lu BH, Zhang AF, Zhu GX, Pi G. The prediction of the building precision in the laser engineered net shaping process using advanced networks. Opt Lasers Eng. 2010;48(5):519-525. doi: 10.1016/j.optlaseng.2010.01.002
- Zhang B, Hong KM, Shin YC. Deep-learning-based porosity monitoring of laser welding process. Manuf Lett, 2020;23:62-66. doi: 10.1016/j.mfglet.2020.01.001
- Hosseini E, Popovich VA. A review of mechanical properties of additively manufactured inconel 718. Addit. Manuf. 2019;30:100877. doi: 10.1016/j.addma.2019.100877
- Zhang J, Wang P, Gao RX. Deep learning-based tensile strength prediction in fused deposition modeling. Comput Ind. 2019;107:11-21. doi: 10.1016/j.compind.2019.01.011
- Zhan Z, Li H. Machine learning based fatigue life prediction with effects of additive manufacturing process parameters for printed SS 316L. Int J Fatigue. 2021;142:105941. doi: 10.1016/j.ijfatigue.2020.105941
- Wang C, Chandra S, Huang S, Tor SB, Tan X. Unraveling process-microstructure-property correlations in powder-bed fusion additive manufacturing through information-rich surface features with deep learning. J Mater Process Technol. 2023;311:17804. doi: 10.1016/j.jmatprotec.2022.117804
- Huang X, Ng WL, Yeong WY. Predicting the number of printed cells during inkjet-based bioprinting process based on droplet velocity profile using machine learning approaches. J. Intell. Manuf. 2023. doi: 10.1007/s10845-023-02184-3
- Mozaffar M. Data-driven prediction of the high-dimensional thermal history in directed energy deposition processes via recurrent neural networks. Manuf Lett. 2018;1:35-39. doi: 10.1016/j.mfglet.2018.10.002
- Mozaffar M, Liao S, Lin H, Ehmann K, Cao J. Geometry-agnostic data-driven thermal modeling of additive manufacturing processes using graph neural networks. Addit Manuf. 2021;48:102449. doi: 10.1016/j.addma.2021.102449
- Saldi ZS. Marangoni Driven Free Surface Flows in Liquid Weld Pools. Netherlands: TuDelft; 2012.
- Sahoo P, Debroy T, McNallan MJ. Surface tension of binary metal-surface active solute systems under conditions relevant to welding metallurgy. Metall Trans B. 1988;19(3):483-491. doi: 10.1007/BF02657748
- Chen C, Wong SJL, Raghavan S, Li H. Design of experiments informed deep learning for modeling of directed energy deposition process with a small-size experimental dataset. Mater Des. 2022;222:111098. doi: 10.1016/j.matdes.2022.111098
- Zhang D. A coefficient of determination for generalized linear models. Am Stat. 2017;71(4):310-316. doi: 10.1080/00031305.2016.1256839
- Chicco D, Warrens MJ, Jurman G. The coefficient of determination R-squared is more informative than SMAPE, MAE, MAPE, MSE and RMSE in regression analysis evaluation. PeerJ Comput Sci. 2021;7:1-24. doi: 10.7717/PEERJ-CS.623
- Wright S. Correlation and causation. J Agric Res. 1921;20(7):557-585.
- Nevitt J, Hancock GR. Improving the root mean square error of approximation for nonnormal conditions in structural equation modeling. J Exp Educ. 2000;68(3):251-268. doi: 10.1080/00220970009600095
- Kelley K, Lai K. Accuracy in parameter estimation for the root mean square error of approximation: Sample size planning for narrow confidence intervals. Multivariate Behav Res. 2011;46(1):1-32. doi: 10.1080/00273171.2011.543027
- Ivakhnenko AG, Lapa VG. Cybernetics and Forecasting Techniques. In: Modern Analytic and Computational Methods in Science and Mathematics. Netherlands: American Elsevier Publishing Company; 1967. Available from: https://books. google.com.sg/books?id=rgfgaaaamaaj [Last accessed on 2023 Dec 23].
- Abadi M, Agarwal A, Barham P, et al., TensorFlow: Large- Scale Machine Learning on Heterogeneous Distributed Systems. CoRR, 2016;abs/1603.04467. Available from: http://arxiv.org/abs/1603.04467 [Last accessed on 2023 Dec 23].