Improvement of multiaxial fatigue life prediction performance based on contrastive learning feature extraction
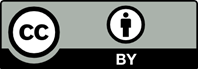
Accurate prediction of multiaxial fatigue life was crucial for structural integrity assessment, yet the variability in material responses under complex loading paths made it challenging for both classical and data-driven models to achieve high accuracy. To address this issue, a contrastive learning-based framework was proposed in this study, enabling the construction of more generalized low-dimensional feature representations across different loading paths. This framework enhanced the robustness of fatigue life prediction without relying on mechanical assumptions. Experimental validation demonstrated that, compared to existing methods, the contrastive learning model learned more suitable feature encodings, significantly improving prediction performance. This framework provided a reference solution for engineering applications requiring reliability assessment under multiaxial stress conditions.

- Towashiraporn P, Gall K, Subbarayan G, et al. Power cycling thermal fatigue of Sn-Pb solder joints on a chip scale package. Int J Fatigue. 2024;26(5):497-510. doi: 10.1016/j.ijfatigue.2003.09.004
- Donnerbauer K, Bill T, Starke P, et al. Fatigue life evaluation of metastable austenitic stainless steel AISI347 based on nondestructive testing methods for different environmental conditions. Int J Fatigue. 2024;179:108056. doi: 10.1016/j.ijfatigue.2023.108056
- Zhao B, Xie L, Song J, et al. Fatigue life prediction of aero-engine compressor disk based on a new stress field intensity approach. Int J Mech Sci. 2020;165:105190. doi: 10.1016/j.ijmecsci.2019.105190
- Liang Q, Peng C, Li X. A multi-state Semi-Markov model for nuclear power plants piping systems subject to fatigue damage and random shocks under dynamic environments. Int J Fatigue. 2023;168:107448. doi: 10.1016/j.ijfatigue.2022.107448
- Yu Z, Sun X, Xing R, Chen X. Unified prediction of uniaxial ratcheting deformation at elevated temperatures with physics-informed multimodal network. Int J Plast. 2025;187:104275. doi: 10.1016/j.ijplas.2025.104275
- Maniar Y, Konstantin G, Sharma A, et al. Solder joint lifetime modeling under random vibrational load collectives. JOM. 2020;72(2):898-905. doi: 10.1007/s11837-019-03947-1
- Wijker JJ. Spacecraft Structures. Berlin: Springer Science & Business Media; 2008.
- Min KD, Lee BS, Kim SJ. Effects of oxide on fatigue crack growth behaviour of type 347 stainless steel in PWR water conditions. Fatigue Fract Eng Mater Struct. 2015;38(8):960-969. doi: 10.1111/ffe.12290
- Agency IAE. Assessment and Management of Ageing of Major Nuclear Power Plant Components Important to Safety: PWR Pressure, IAEA-TECDOC-1556, IAEA, Vienna; 2007.
- Guo C, Yu D, Sun X, et al. Fatigue failure mechanism and life prediction of a cast duplex stainless steel after thermal aging. Int J Fatigue. 2021;146:106161. doi: 10.1016/j.ijfatigue.2021.106161
- Kishore P, Mondal A, Trivedi A, et al. A microstructure sensitive machine learning-based approach for predicting fatigue life of additively manufactured parts. Int J Fatigue. 2025;192:108724. doi: 10.1016/j.ijfatigue.2024.108724
- Yang J, Kang G, Liu Y, Kan Q. A novel method of multiaxial fatigue life prediction based on deep learning. Int J Fatigue. 2021;151:106356. doi: 10.1016/j.ijfatigue.2021.106356
- Zhang M, Sun CN, Zhang X, et al. High cycle fatigue life prediction of laser additive manufactured stainless steel: A machine learning approach. Int J Fatigue. 2019;128:105194. doi: 10.1016/j.ijfatigue.2019.105194
- Li J, Yang Z, Qian G, Berto F. Machine learning based very-high-cycle fatigue life prediction of Ti-6Al-4V alloy fabricated by selective laser melting. Int J Fatigue. 2022;158:106764. doi: 10.1016/j.ijfatigue.2022.106764
- Jing G, Ma T, Wang Z, et al. Physical hierarchical neural network for low cycle fatigue life prediction of compacted graphite cast iron based on small data. Int J Fatigue. 2024;188:108509. doi: 10.1016/j.ijfatigue.2024.108509
- Sun X, Zhou K, Shi S, Song K, Chen X. A new cyclical generative adversarial network based data augmentation method for multiaxial fatigue life prediction. Int J Fatigue. 2022;162:106996. doi: 10.1016/j.ijfatigue.2022.106996
- Sun X, Zhou T, Song K, Chen X. An image recognition based multiaxial low-cycle fatigue life prediction method with CNN model. Int J Fatigue. 2023;167:107324. doi: 10.1016/j.ijfatigue.2022.107324
- Zhou T, Sun X, Yu Z, Chen X. A generalization ability-enhanced image recognition based multiaxial fatigue life prediction method for complex loading conditions. Eng Fract Mech. 2024;295:109802. doi: 10.1016/j.engfracmech.2023.109802
- Wang H, Zhang J, Li B, et al. Machine learning-based fatigue life prediction of laser powder bed fusion additively manufactured Hastelloy X via nondestructively detected defects. Int J Struct Integr. 2024;16(1):104-126. doi: 10.1108/IJSI-09-2024-0161
- Zhao F, Cui J, Yuan M, Zhao J. A weakly supervised pairwise comparison learning approach for bearing health quantitative evaluation and remaining useful life prediction. Eng Computat. 2023;40(7/8):1593-1616. doi: 10.1108/EC-12-2022-0747
- Mao M, Wang W, Lu C, Jia F, Long X. Machine learning for board-level drop response of BGA packaging structure. Microelectron Reliabil. 2022;134:114553. doi: 10.1016/j.microrel.2022.114553
- Long X, Lu C, Shen Z, Su Y. Identification of Mechanical Properties of Thin-Film Elastoplastic Materials by Machine Learning. Acta Mechanica Solida Sinica. 2023;36(1):13-21. doi: 10.1007/s10338-022-00340-5
- Long X, Mao M, Lu C, Li C, Li R. Modeling of heterogeneous materials at high strain rates with machine learning algorithms trained by finite element simulations. J Micromech Mol Phys. 2021;6(1):2150001. doi: 10.1142/S2424913021500016
- Cao W, Sun X, Li Y, et al. Multiaxial damage parameter evaluation by neural network-based symbolic regression. Eng Fract Mechan. 2025;315:110809. doi: 10.1016/j.engfracmech.2025.110809
- Jiang L, Hu Y, Liu Y, et al. Physics-informed machine learning for low-cycle fatigue life prediction of 316 stainless steels. Int J Fatigue. 2024;182:108187. doi: 10.1016/j.ijfatigue.2024.108187
- Zhu S, Zhang Y, Zhu B, et al. High cycle fatigue life prediction of titanium alloys based on a novel deep learning approach. Int J Fatigue. 2024;182:108206. doi: 10.1016/j.ijfatigue.2024.108206
- Liao H, Pan J, Su X, Sun X, Chen X. A path-dependent adaptive physics-informed neural network for multiaxial fatigue life prediction. Int J Fatigue. 2025;193:108799. doi: 10.1016/j.ijfatigue.2024.108799
- Chen S, Zhou X, Bai Y. A frequency domain enhanced multi-view neural network approach to multiaxial fatigue life prediction for various metal materials. Int J Fatigue. 2025;190:108620. doi: 10.1016/j.ijfatigue.2024.108620
- Zhang P, Tang K, Wang A, Wu H, Zhong Z. Neural network integrated with symbolic regression for multiaxial fatigue life prediction. Int J Fatigue. 2024;188:108535. doi: 10.1016/j.ijfatigue.2024.108535
- Long X, Li H, Iyela PM, Kang SB. Predicting the bond stress-slip behavior of steel reinforcement in concrete under static and dynamic loadings by finite element, deep learning and analytical methods. Eng Fail Anal. 2024;161:108312. doi: 10.1016/j.engfailanal.2024.108312
- Zhou K, Sun X, Shi S, Song K, Chen X. Machine learning-based genetic feature identification and fatigue life prediction. Fatigue Fract Eng Mater Struct. 2021;44(9):2524-2537. doi: 10.1111/ffe.13532
- Zhou T, Sun X, Chen X. A multiaxial low-cycle fatigue prediction method under irregular loading by ANN model with knowledge-based features. Int J Fatigue. 2023;176:107868. doi: 10.1016/j.ijfatigue.2023.107868
- Long X, Ding X, Li J, Dong R, Su Y, Chang C. Indentation reverse algorithm of mechanical response for elastoplastic coatings based on LSTM deep learning. Materials. 2023;16(7):2617. doi: 10.3390/ma16072617
- Wang CH, Brown MW. A path-independent parameter for fatigue under proportional and non-proportional loading. Fatigue Fract Eng Mater Struct. 1993;16(12):1285-1297. doi: 10.1111/j.1460-2695.1993.tb00739.x
- Fatemi A, Socie DF. A critical plane approach to multiaxial fatigue damage including out-of-phase loading. Fatigue Fract Eng Mater Struct. 1988;11(3):149-165. doi: 10.1111/j.1460-2695.1988.tb01169.x
- Zhan Z, Hu W, Li B, Zhang Y, Meng Q, Guan Z. Continuum damage mechanics combined with the extended finite element method for the total life prediction of a metallic component. Int J Mech Sci. 2017;124-125:48-58. doi: 10.1016/j.ijmecsci.2017.03.002
- Yang S, Hu W, Meng Q, Zhao B. A new continuum damage mechanics-based two-scale model for high-cycle fatigue life prediction considering the two-segment characteristic in S-N curves. Fatigue Fract Eng Mater Struct. 2020;43(2):387-402. doi: 10.1111/ffe.13161
- Brown MW, Miller KJ. A theory for fatigue failure under multiaxial stress-strain conditions. Proc Inst Mech Eng. 1973;187(1):745-755. doi: 10.1243/pime_proc_1973_187_161_02
- Carraro PA, Quaresimin M. A damage based model for crack initiation in unidirectional composites under multiaxial cyclic loading. Compos Sci Technol. 2014;99:154-163. doi: 10.1016/j.compscitech.2014.05.012
- Wang L, Zhu S, Luo C. Physics-guided machine learning frameworks for fatigue life prediction of AM materials. Int J Fatigue. 2023;172:107658. doi: 10.1016/j.ijfatigue.2023.107658
- Dong Y, Yang X, Chang D, Li Q. Predicting fatigue life of multi-defect materials using the fracture mechanics-based physics-informed neural network framework. Int J Fatigue. 2025;190:108626. doi: 10.1016/j.ijfatigue.2024.108626
- Fan JL, Zhu G, Zhu ML, Xuan FZ. A data-physics integrated approach to life prediction in very high cycle fatigue regime. Int J Fatigue. 2023;176:107917. doi: 10.1016/j.ijfatigue.2023.107917
- Gan L, Wu H, Zhong Z. On the use of data-driven machine learning for remaining life estimation of metallic materials based on Ye-Wang damage theory. Int J Fatigue. 2022;156:106666. doi: 10.1016/j.ijfatigue.2021.106666
- Wang H, Li B, Xuan FZ. Fatigue-life prediction of additively manufactured metals by continuous damage mechanics (CDM)-informed machine learning with sensitive features. Int J Fatigue. 2022;164:107147. doi: 10.1016/j.ijfatigue.2022.107147
- Cam LML, Neyman J. Proceedings of the Fifth Berkeley Symposium on Mathematical Statistics and Probability. United States: University of California Press; 1967.
- Kingma DP, Welling M. Auto-Encoding Variational Bayes. In Proceedings of the 2nd International Conference on Learning Representations (ICLR2014); 2014. doi: 10.48550/arXiv.1312.6114
- Zhang CS, Chen J, Li QL, Deng BQ, Wang J, Chen CC. Deep contrastive learning: A survey. Acta Automatica Sin. 2023;49(1):15-39. doi: 10.16383/j.aas.c220421
- Jaiswal A, Babu AR, Zadeh MZ, Banerjee D, Makedon F. A survey on contrastive self-supervised learning. Technologies. 2021;9(1):2. doi: 10.3390/technologies9010002
- Le-Khac PH, Healy G, Smeaton AF. Contrastive representation learning: A framework and review. IEEE Access. 2020;8:193907-193934. doi: 10.1109/ACCESS.2020.3031549
- Liu X, Zhang F, Hou Z, et al. Self-supervised Learning: Generative or Contrastive. In: IEEE Transactions on Knowledge and Data Engineering; 2021. p. 1-1. doi: 10.1109/TKDE.2021.3090866
- van den Oord A, Li Y, Vinyals O. Representation Learning with Contrastive Predictive Coding; 2019. arXiv. Available from: https://arxiv.org/abs/1807.03748 [Last accessed on 2025 Jan 08].
- He K, Fan H, Wu Y, et al. Momentum Contrast for Unsupervised Visual Representation Learning. In: 2020 IEEE/CVF Conference on Computer Vision and Pattern Recognition (CVPR); 2020. p. 9726-9735. Available from: https://ieeexplore.ieee.org/document/9157636 [Last accessed on 2025 Jan 08].
- Grill JB, Strub F, Altché F, et al. Bootstrap your own latent a new approach to self-supervised learning. In: Proceedings of the 34th International Conference on Neural Information Processing Systems. Red Hook, NY, USA: Curran Associates Inc.; 2020. p. 21271-21284.
- Radford A, Kim JW, Hallacy C, et al. Learning Transferable Visual Models from Natural Language Supervision. In: International Conference on Machine Learning. PMLR; 2021. p. 8748-8763.
- Yang C, An Z, Zhou H, et al. Online knowledge distillation via mutual contrastive learning for visual recognition. IEEE Trans Pattern Anal Mach Intell. 2023;45(8):10212-10227. doi: 10.1109/TPAMI.2023.3257878
- Baevski A, Zhou H, Mohamed A, Auli M. wav2vec 2.0: A Framework for Self-Supervised Learning of Speech Representations. Proceedings of the 34th International Conference on Neural Information Processing Systems (NeurIPS 2020); 2020. p. 12449-12460.
- Hershey JR, Chen Z, Le Roux J, Watanabe S. Deep clustering: Discriminative embeddings for segmentation and separation. In: 2016 IEEE International Conference on Acoustics, Speech and Signal Processing (ICASSP); 2016. p. 31-35. Available from: https://ieeexplore.ieee.org/ abstract/document/7471631 [Last accessed on 2025 Jan 13].
- Hu H, Wang X, Zhang Y, Chen Q, Guan Q. A comprehensive survey on contrastive learning. Neurocomputing. 2024;610:128645. doi: 10.1016/j.neucom.2024.128645
- Gutmann M, Hyvärinen A. Noise-contrastive estimation: A new estimation principle for unnormalized statistical models. In: Proceedings of the Thirteenth International Conference on Artificial Intelligence and Statistics. JMLR Workshop and Conference Proceedings; 2010. p. 297-304.
- Caron M, Misra I, Mairal J, Goyal P, Bojanowski P, Joulin A. Unsupervised learning of visual features by contrasting cluster assignments. In: Proceedings of the 34th International Conference on Neural Information Processing Systems. Red Hook, NY, USA: Curran Associates Inc., 2020. p. 9912-9924.
- Reimers N, Gurevych I. Sentence-BERT: Sentence Embeddings using Siamese BERT-Networks. In: Proceedings of the 2019 Conference on Empirical Methods in Natural Language Processing and the 9th International Joint Conference on Natural Language Processing (EMNLP-IJCNLP). Hong Kong, China: Association for Computational Linguistics; 2019. p. 3982-3992.
- Rusak E, Reizinger P, Juhos A, Bringmann O, Zimmermann RS, Brendel W. InfoNCE: Identifying the Gap between Theory and Practice. arXiv; 2024. Available from: https://arxiv.org/ abs/2407.00143 [Last accessed on 2025 Jan 08].
- Luo Y, Chen Z, Hershey JR, Le Roux J, Mesgarani N. Deep clustering and conventional networks for music separation: Stronger together. In: 2017 IEEE International Conference on Acoustics, Speech and Signal Processing (ICASSP); 2017. p. 61-65. Available from: https://ieeexplore.ieee.org/ abstract/document/7952118 [Last accessed on 2025 Jan 08].
- Wu S, Wang Y, Jiang Y, Zhang Q, Liu J. CRATI: Contrastive representation-based multimodal sound event localization and detection. Knowl Based Syst. 2024;305:112692. doi: 10.1016/j.knosys.2024.112692
- Chen T, Kornblith S, Norouzi M, Hinton G. A simple framework for contrastive learning of visual representations. In: Proceedings of the 37th International Conference on Machine Learning. Vol. 119. JMLR; 2020.
- Guo Q, Wang C, Xiao D, Huang Q. A lightweight open-world pest image classifier using ResNet8-based matching network and NT-Xent loss function. Expert Syst Appl. 2024;237:121395. doi: 10.1016/j.eswa.2023.121395
- Kim T, Yoo KM, Lee SG. Self-Guided Contrastive Learning for BERT Sentence Representations. In: Proceedings of the 59th Annual Meeting of the Association for Computational Linguistics and the 11th International Joint Conference on Natural Language Processing (Volume 1: Long Papers); 2021. p. 2528-2540. doi: 10.18653/v1/2021.acl-long.197
- van der Maaten L, Hinton G. Visualizing data using t-SNE. J Mach Learn Res. 2008;9(86):2579-2605.