CockpitGemini: A personalized design framework for smart vehicle cockpits integrating generative model-based multi-agent systems and human digital twins
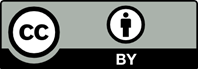
The evolution of smart vehicle cockpits is transitioning from serving as mere driving tools to becoming intimate partners that significantly enhance user experiences through advanced technologies. This research addresses the growing demand for personalized design in smart vehicle cockpits by proposing a framework, CockpitGemini. This framework integrates generative model-based multi-agent systems and human digital twins, enabling tailored designs and services based on user preferences and real-time status. The capabilities of the proposed framework are illustrated through four dimensions: personalized product design, personalized interactive interface design, user state monitoring and personalized regulation, and personalized driving strategy recommendations. A case study on the design of personalized vehicle seats demonstrates the feasibility and usability of the CockpitGemini framework, highlighting its potential to enhance user satisfaction in smart vehicle cockpits.
- Chen L, Li Y, Huang C, et al. Milestones in autonomous driving and intelligent vehicles: Survey of surveys. IEEE Trans Intell Veh. 2023;8(2):1046-1056. doi: 10.1109/TIV.2022.3223131
- Li W, Wu L, Wang C, et al. Intelligent cockpit for intelligent vehicle in metaverse: A case study of empathetic auditory regulation of human emotion. IEEE Trans Syst Man Cybern Syst. 2023;53(4):2173-2187. doi: 10.1109/TSMC.2022.3229021
- Stappen L, Dillmann J, Striegel S, Vögel HJ, Flores-Herr N, Schuller BW. Integrating Generative Artificial Intelligence in Intelligent Vehicle Systems. In: IEEE Conference on Intelligent Transportation Systems, Proceedings, (ITSC). United States: Institute of Electrical and Electronics Engineers Inc.; 2023. p. 5790-5797. doi: 10.1109/ITSC57777.2023.10422003
- Chen H, Gao R, Fan L, et al. Scenario-function system for automotive intelligent cockpits: Framework, research progress and perspectives. IEEE Trans Intell Veh. 2024;9:4890-4904. doi: 10.1109/TIV.2024.3382995
- Dou R, Lin D, Nan G, Lei S. A method for product personalized design based on prospect theory improved with interval reference. Comput Ind Eng. 2018;125:708-719. doi: 10.1016/j.cie.2018.04.056
- Cao Y, Li S, Liu Y, et al. A Comprehensive survey of AI-Generated Content (AIGC): A history of generative AI from GAN to ChatGPT. 2023. doi: 10.48550/arXiv.2303.04226
- Qin HX, Hui P. Empowering the Metaverse with Generative AI: Survey and Future Directions. In: Proceedings-2023 IEEE 43rd International Conference on Distributed Computing Systems Workshops, (ICDCSW 2023). United States: Institute of Electrical and Electronics Engineers Inc.; 2023. p. 85-90. doi: 10.1109/ICDCSW60045.2023.00022
- Ren M, Zheng P. Towards smart product-service systems 2.0: A retrospect and prospect. Adv Eng Inform. 2024;61:102466. doi: 10.1016/j.aei.2024.102466
- Dorri A, Kanhere SS, Jurdak R. Multi-agent systems: A survey. IEEE Access. 2018;6:28573-28593. doi: 10.1109/ACCESS.2018.2831228
- Wang B, Zhou H, Li X, et al. Human digital twin in the context of industry 5.0. Robot Comput Integr Manuf. 2024;85:102626. doi: 10.1016/j.rcim.2023.102626
- Barricelli BR, Casiraghi E, Gliozzo J, Petrini A, Valtolina S. Human digital twin for fitness management. IEEE Access. 2020;8:26637-26664. doi: 10.1109/ACCESS.2020.2971576
- Wang Z, Zhang M, Sun H, Zhu G. Effects of standardization and innovation on mass customization: An empirical investigation. Technovation. 2016;48-49:79-86. doi: 10.1016/j.technovation.2016.01.003
- Myrodia A, Kristjansdottir K, Hvam L. Impact of product configuration systems on product profitability and costing accuracy. Comput Ind. 2017;88:12-18. doi: 10.1016/j.compind.2017.03.001
- Levandowski CE, Jiao JR, Johannesson H. A two-stage model of adaptable product platform for engineering-to-order configuration design. J Eng Des. 2015;26(7-9):220-235. doi: 10.1080/09544828.2015.1021305
- Dong L, Ren M, Xiang Z, Zheng P, Cong J, Chen CH. A novel smart product-service system configuration method for mass personalization based on knowledge graph. J Clean Prod. 2023;382:135270. doi: 10.1016/j.jclepro.2022.135270
- Hu K, Qiu L, Zhang S, Wang Z, Fang N. ICCP: A heuristic process planning method for personalized product configuration design. Appl Intell. 2023;53(24):30887-30910. doi: 10.1007/s10489-023-05186-z
- Ren M, Dong L, Xia Z, Cong J, Zheng P. A proactive interaction design method for personalized user context prediction in smart-product service system. Procedia CIRP. 2023;119:963-968. doi: 10.1016/j.procir.2023.01.021
- Zheng P, Yu S, Wang Y, Zhong RY, Xu X. User-experience based product development for mass personalization: A case study. Procedia CIRP. 2017;63:2-7. doi: 10.1016/j.procir.2017.03.122
- Zheng P, Xu X, Chen CH. A data-driven cyber-physical approach for personalised smart, connected product co-development in a cloud-based environment. J Intell Manuf. 2020;31(1):3-18. doi: 10.1007/s10845-018-1430-y
- Peng DX, Heim GR, Mallick DN. Collaborative product development: The effect of project complexity on the use of information technology tools and new product development practices. Prod Oper Manag. 2014;23(8):1421-1438. doi: 10.1111/j.1937-5956.2012.01383.x
- Devlin J, Chang MW, Lee K, Toutanova K. BERT: Pre-training of deep bidirectional transformers for language understanding. 2018. doi: 10.48550/arXiv.1810.04805
- Touvron H, Lavril T, Izacard G, et al. LLaMA: Open and efficient foundation language models. 2023. doi: 10.48550/arXiv.2302.13971
- Wang B, Zuo H, Cai Z, et al. A Task-decomposed Ai-aided Approach for Generative Conceptual Design. In: Proceedings of the ASME Design Engineering Technical Conference. Vol 6. New York: American Society of Mechanical Engineers (ASME); 2023. doi: 10.1115/detc2023-109087
- Jiang S, Luo J. Autotriz: Artificial ideation with triz and large language models. 2024. doi: 10.48550/arXiv.2403.13002
- Yin H, Zhang Z, Liu Y. The exploration of integrating the midjourney artificial intelligence generated content tool into design systems to direct designers towards future-oriented innovation. Systems. 2023;11(12):566. doi: 10.3390/systems11120566
- Wu Q, Bansal G, Zhang J, et al. AutoGen: Enabling next-gen llm applications via multi-agent conversation. 2023. doi: 10.48550/arXiv.2308.08155
- Hong S, Zhuge M, Chen J, et al. MetaGPT: Meta programming for a multi-agent collaborative framework. 2023. doi: 10.48550/arXiv.2308.00352
- Chen L, Jing Q, Tsang Y, Wang Q, Sun L, Luo J. DesignFusion: Integrating generative models for conceptual design enrichment. J Mech Des. 2024;146(11):111703. doi: 10.1115/1.4065487
- Çelen A, Han G, Schindler K, et al. I-Design: Personalized LLM interior designer. 2024. doi: 10.48550/arXiv.2404.02838
- Xu S, Wei Y, Zheng P, Zhang J, Yu C. LLM enabled generative collaborative design in a mixed reality environment. J Manuf Syst. 2024;74:703-715. doi: 10.1016/j.jmsy.2024.04.030
- Chen D, Cheng S, Hu J, Kasoar M, Arcucci R. Explainable global wildfire prediction models using graph neural networks. 2024. doi: 10.48550/arXiv.2402.07152
- Gong H, Cheng S, Chen Z, et al. An efficient digital twin based on machine learning SVD autoencoder and generalised latent assimilation for nuclear reactor physics. Ann Nucl Energy. 2022;179:109431. doi: 10.1016/j.anucene.2022.109431
- Zhong C, Cheng S, Kasoar M, Arcucci R. Reduced-order digital twin and latent data assimilation for global wildfire prediction. Nat Hazards Earth Syst Sci. 2023;23(5):1755-1768. doi: 10.5194/nhess-23-1755-2023
- Lauer-Schmaltz MW, Cash P, Hansen JP, Maier A. Towards the human digital twin: Definition and design--A survey. 2024. doi: 10.48550/arXiv.2402.07922
- Lin Y, Chen L, Ali A, et al. Human digital twin: A survey. J Cloud Comp. 2024;13:131. doi: 10.1186/S13677-024-00691-Z
- Okegbile SD, Cai J, Niyato D, Yi C. Human digital twin for personalized healthcare: Vision, architecture and future directions. IEEE Netw. 2023;37(2):262-269. doi: 10.1109/MNET.118.2200071
- Chen J, Yi C, Du H, et al. A revolution of personalized healthcare: Enabling human digital twin with mobile AIGC. IEEE Netw. 2024. doi: 10.1109/MNET.2024.3366560
- Fan J, Zheng P, Lee CKM. A Vision-based human digital twin modeling approach for adaptive human-robot collaboration. J Manuf Sci Eng. 2023;145(12):121002. doi: 10.1115/1.4062430
- Aboulsafa EI, Khayat GAE, Elmorsy SA. An Educational Human Digital Twin Proposed Model for Personalized E-Learning. In: 2023 1st IEEE Afro-Mediterranean Conference on Artificial Intelligence, AMCAI 2023 - Proceedings. United States: Institute of Electrical and Electronics Engineers Inc.; 2023. doi: 10.1109/AMCAI59331.2023.10431503
- He Q, Li L, Li D, et al. From digital human modeling to human digital twin: Framework and perspectives in human factors. Chin J Mech Eng. 2024;37(1):9. doi: 10.1186/s10033-024-00998-7
- El Saddik A, Hossain MS, Kantarci B, Editors K. Connected Health in Smart Cities. Berlin: Springer Nature; 2020. doi: 10.1007/978-3-030-27844-1
- Goodwin GC, Seron MM, Medioli AM, Smith T, King BR, Smart CE. A systematic stochastic design strategy achieving an optimal tradeoff between peak BGL and probability of hypoglycaemic events for individuals having type 1 diabetes mellitus. Biomed Signal Process Control. 2020;57:101813. doi: 10.1016/j.bspc.2019.101813
- Karras T, Laine S, Aila T. A style-based generator architecture for generative adversarial networks. 2018. doi: 10.48550/arXiv.1812.04948
- Poole B, Jain A, Barron JT, Mildenhall B. DreamFusion: Text-to-3D using 2D Diffusion. 2022. doi: 10.48550/arXiv.2209.14988
- Gonçalves G, Coelho H, Monteiro P, Melo M, Bessa M. Systematic review of comparative studies of the impact of realism in immersive virtual experiences. ACM Comput Surv. 2022;55(6):115. doi: 10.1145/3533377
- Barricelli BR, Casiraghi E, Fogli D. A survey on digital twin: Definitions, characteristics, applications, and design implications. IEEE Access. 2019;7:167653-167671. doi: 10.1109/ACCESS.2019.2953499
- Manghisi VM, Uva AE, Fiorentino M, Bevilacqua V, Trotta GF, Monno G. Real time RULA assessment using Kinect v2 sensor. Appl Ergon. 2017;65:481-491. doi: 10.1016/j.apergo.2017.02.015
- Tanwar R, Phukan OC, Singh G, Pal PK, Tiwari S. Attention based hybrid deep learning model for wearable based stress recognition. Eng Appl Artif Intell. 2024;127:107391. doi: 10.1016/j.engappai.2023.107391
- Debie E, Fernandez Rojas R, Fidock J, et al. Multimodal fusion for objective assessment of cognitive workload: A review. IEEE Trans Cybern. 2021;51(3):1542-1555. doi: 10.1109/TCYB.2019.2939399
- Ramalakshmi K, Srinivasa Raghavan V, Rajagopal S, et al. An extensive analysis of artificial intelligence and segmentation methods transforming cancer recognition in medical imaging. Biomed Phys Eng Express. 2024;10(4):045046. doi: 10.1088/2057-1976/ad555b
- Wang J, Sun K, Cheng T, et al. Deep high-resolution representation learning for visual recognition. IEEE Trans Pattern Anal Mach Intell. 2021;43:3349-3364. doi: 10.1109/TPAMI.2020.2983686
- Hignett S, Ergonomist LM. Rapid Entire Body Assessment (REBA). Appl Ergon. 2000;31:201-205. doi: 10.1016/S0003-6870(99)00039-3
- Parsa B, Banerjee AG. A Multi-Task Learning Approach for Human Activity Segmentation and Ergonomics Risk Assessment. In: 2021 IEEE Winter Conference on Applications of Computer Vision (WACV); 2021. doi: 10.48550/arXiv.2008.03014
- Wei J, Wang H, Feng J, Lin G, Yap KH. TAPS3D: Text-guided 3D textured shape generation from pseudo supervision. 2023. doi: 10.48550/arXiv.2303.13273
- Radford A, Kim JW, Hallacy C, et al. Learning transferable visual models from natural language supervision. 2021. doi: 10.48550/arXiv.2103.00020
- Deitke M, Schwenk D, Salvador J, et al. Objaverse: A universe of annotated 3D objects. 2022. doi: 10.48550/arXiv.2212.08051