Revolutionizing drug response prediction: An unmet requirement for patients unresponsive to precision medicine
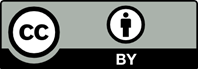
Precision cancer therapies frequently fail due to tumors’ evolving clonal diversity rather than drug efficacy. Even when initial treatment succeeds, resistance often emerges, leading to relapse. Clinicians then find themselves in the same cycle of repeating the process of testing a new drug until therapeutic exhaustion. The cycle escalates with each new treatment until no further options are available. The real-life experience of precision therapy will undeniably lead to an upgrade – from biomarker testing to drug response prediction – accordingly to favor more effective treatment options, more clinical benefit, and more patient coverage to include non-responders. While biomarker tests (or companion diagnostics) advance precision medicine by identifying only a fraction of patients as responders, drug response prediction aims to expand treatment options – particularly for non-responders – by tailoring personalized therapies to optimize outcomes while minimizing side effects. Artificial intelligence-driven approaches (e.g., deep learning and predictive modeling) leverage large datasets to generate these predictions. However, such systems remain experimental, not yet ready for clinical use. Patient-derived gene expression-informed anticancer drug efficacy (PGA) is the ultimate answer to the unmet clinical need for a quick turnaround and cost-efficient drug response prediction technology. With PGA, therapeutic non-responders now are able to benefit from more drug options than ever before. Since the technology is fitted with patient testing, gene activity detection, data mapping, drug matching, and efficacy ranking capabilities, clinicians can be quickly notified of potentially effective drugs, winning the decisive time for decision-making.
- World Health Organization. Cancer Fact Sheet. World Health Organization; 2022. Available from: https://www. who.int/news-room/fact-sheets/detail/cancer [Last accessed on 2024 Aug 13].
- Wong CH, Siah KW, Lo AW. Corrigendum: Estimation of clinical trial success rates and related parameters. Biostatistics. 2018;20(2):366-366. doi: 10.1093/biostatistics/kxy072
- Davis C, Naci H, Gurpinar E, Poplavska E, Pinto A, Aggarwal A. Availability of evidence of benefits on overall survival and quality of life of cancer drugs approved by European Medicines Agency: Retrospective cohort study of drug approvals 2009-13. BMJ. 2017;359:j4530. doi: 10.1136/bmj.j4530
- Kim C, Prasad V. Cancer drugs approved on the basis of a surrogate end point and subsequent overall survival: An analysis of 5 years of US Food and Drug Administration Approvals. JAMA Intern Med. 2015;175(12):1992-1994. doi: 10.1001/jamainternmed.2015.5868
- Lin A, Giuliano CJ, Palladino A, et al. Off-target toxicity is a common mechanism of action of cancer drugs undergoing clinical trials. Sci Transl Med. 2019;11(509):eaaw8412. doi: 10.1126/scitranslmed.aaw8412
- Idrisova KF, Simon HU, Gomzikova MO. Role of patient-derived models of cancer in translational oncology. Cancers (Basel). 2022;15(1):139. doi: 10.3390/cancers15010139
- Bashor CJ, Hilton IB, Bandukwala H, Smith DM, Veiseh O. Engineering the next generation of cell-based therapeutics. Nat Rev Drug Discov. 2022;21:655-675. doi: 10.1038/s41573-022-00476-6
- Kim J, Koo BK, Knoblich JA. Human organoids: Model systems for human biology and medicine. Nat Rev Mol Cell Biol. 2020;21:571-584. doi: 10.1038/s41580-020-0259-3
- Yeh C. Enabling real-world data to accelerate the development of innovative cancer biomarkers. Glob Med Genet. 2023;10:97-100. doi: 10.1055/s-0043-1768993
- Haslam A, Kim MS, Prasad V. Updated estimates of eligibility for and response to genome-targeted oncology drugs among US cancer patients, 2006-2020. Ann Oncol. 2021;32(7): 926-932. doi: 10.1016/j.annonc.2021.04.003
- Yeh C, Lin ST, Lai HC. A transformative technology linking patient’s mRNA expression profile to anticancer drug efficacy. Onco. 2024;4(3):143-162. doi: 10.3390/onco4030012
- He D, Liu Q, Wu Y, Xie L. A context-aware deconfounding autoencoder for robust prediction of personalized clinical drug response from cell-line compound screening. Nat Mach Intell. 2022;4:879-892. doi: 10.1038/s42256-022-00541-0
- Sagingalieva A, Kordzanganeh M, Kenbayev N, Kosichkina D, Tomashuk T, Melnikov A. Hybrid quantum neural network for drug response prediction. Cancers (Basel). 2023;15(10):2705. doi: 10.3390/cancers15102705
- Liu X, Zhang W. A subcomponent-guided deep learning method for interpretable cancer drug response prediction. PLoS Comput Biol. 2023;19(8):e1011382. doi: 10.1371/journal.pcbi.1011382
- Yang Y, Li P. GPDRP: A multimodal framework for drug response prediction with graph transformer. BMC Bioinformatics. 2023;24:484. doi: 10.1186/s12859-023-05618-0
- Taj F, Stein LD. MMDRP: Drug response prediction and biomarker discovery using multi-modal deep learning. Bioinform Adv. 2024;4(1):vbae010. doi: 10.1093/bioadv/vbae010
- Liu H, Wang F, Yu J, et al. DBDNMF: A dual branch deep neural matrix factorization method for drug response prediction. PLoS Comput Biol. 2024;20(4):e1012012. doi: 10.1371/journal.pcbi.1012012
- Pfohl U, Pflaume A, Regenbrecht M, et al. Precision oncology beyond genomics: The future is here-it is just not evenly distributed. Cells. 2021;10(4):928. doi: 10.3390/cells10040928
- Malone ER, Oliva M, Sabatini PJB, Stockley TL, Siu LL. Molecular profiling for precision cancer therapies. Genome Med. 2020;12:8. doi: 10.1186/s13073-019-0703-1