YeeZzzy does it: Using Kanye West’s tweets to identify sleep and emotional disturbances through digital rest-activity rhythms analysis
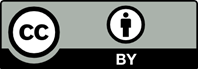
One of the greatest challenges faced by precision medicine is the identification of biomarkers capable of detecting clinically meaningful change at the individual level, not just among large-scale population studies. To this end, the high-volume nature of an individual’s social media data could be leveraged with single-user precision to monitor sleep patterns and tweet content to determine emotional state. However, there is a lack of established methods to detect and estimate sleep and mood using social-media activity. We present here a new approach (digital rest-activity rhythms analysis) to using social media to track both sleep and mood, with potential applications to mental health monitoring and prevention. Our proof-of-concept showed that the emotional content of a single user’s tweets (Kanye West “@Ye”) were influenced by sleep disturbances inferred from usage over a 2-year period. We herein provide an ethical and theoretical-framework of how to proceed among this sensitive yet potentially fruitful field.
- Golder SA, Macy MW. Diurnal and seasonal mood vary with work, sleep, and daylength across diverse cultures. Science. 2011;333(6051):1878-1881. doi: 10.1126/science.1202775
- Roenneberg T. Twitter as a means to study temporal behaviour. Curr Biol. 2017;27(17):R830-R832. doi: 10.1016/j.cub.2017.08.005
- Finan PH, Quartana PJ, Remeniuk B, et al. Partial sleep deprivation attenuates the positive affective system: Effects across multiple measurement modalities. Sleep. 2017;40(1):zsw017. doi: 10.1093/sleep/zsw017
- Tausczik YR, Pennebaker JW. The psychological meaning of words: LIWC and computerized text analysis methods. J Lang Soc Psychol. 2010;29(1):24-54. doi: 10.1177/0261927X09351676
- Reid MJ, Omlin X, Espie CA, Sharman R, Tamm S, Kyle SD. The effect of sleep continuity disruption on multimodal emotion processing and regulation: A laboratory‐based, randomised, controlled experiment in good sleepers. J Sleep Res. 2023;32(1):e13634. doi: 10.1111/jsr.13634
- Nahum-Shani I, Smith SN, Spring BJ, et al. Just-in-time adaptive interventions (JITAIs) in mobile health: Key components and design principles for ongoing health behavior support. Ann Behav Med. 2018;52(6):446-462. doi: 10.1007/s12160-016-9830-8
- Smith MT, McCrae CS, Cheung J, et al. Use of actigraphy for the evaluation of sleep disorders and circadian rhythm sleep-wake disorders: An American Academy of Sleep Medicine clinical practice guideline. J Clin Sleep Med. 2018;14(7):1231-1237. doi: 10.5664/jcsm.7230
- Araujo T, Wonneberger A, Neijens P, de Vreese C. How much time do you spend online? Understanding and improving the accuracy of self-reported measures of internet use. Commun Methods Meas. 2017;11(3):173-190. doi: 10.1080/19312458.2017.1317337
- Vanden Abeele M, Beullens K, Roe K. Measuring mobile phone use: Gender, age and real usage level in relation to the accuracy and validity of self-reported mobile phone use. Mobile Media Commun. 2013;1(2):213-236. doi: 10.1177/2050157913477095
- Levenson JC, Shensa A, Sidani JE, Colditz JB, Primack BA. Social media use before bed and sleep disturbance among young adults in the United States: A nationally representative study. Sleep. 2017;40(9):zsx113. doi: 10.1093/sleep/zsx113
- Shin JC, Kim J, Grigsby-Toussaint D. Mobile phone interventions for sleep disorders and sleep quality: Systematic review. JMIR Mhealth Uhealth. 2017;5(9):e7244. doi: 10.2196/mhealth.7244
- Brown TM, Brainard GC, Cajochen C, et al. Recommendations for daytime, evening, and nighttime indoor light exposure to best support physiology, sleep, and wakefulness in healthy adults. PLoS Biol. 2022;20(3):e3001571. doi: 10.1371/journal.pbio.3001571
- Gubin D, Danilenko K, Stefani O, et al. Blue light and temperature actigraphy measures predicting metabolic health are linked to melatonin receptor polymorphism. Biology (Basel). 2024;13(1):22. doi: 10.3390/biology13010022